Splitting the Basketball Atom
By Seth Partnow
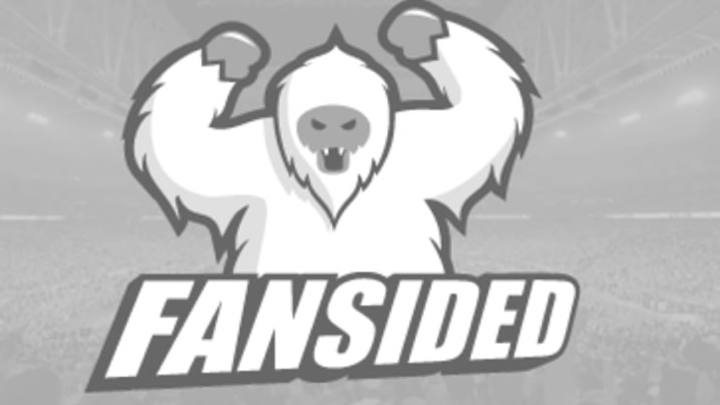
Long before the advent of today’s “analytics” movement, the brighter minds in basketball understood that the box score is an imperfect measure of on-court production. In fact the items which appear in the box score are to a degree arbitrary — there is no particular reason why a steal or a block is considered a box score-worthy event and a charge taken or a pass deflected is not, aside from that’s the way it’s always been done.
“Every year, we come here and have the same discussion: how can the geeks communicate with the jocks?” —Zach Lowe, by way of leading off the “Basketball Analytics” Panel at SSAC 2014.
Among NBA decision-makers the “fantasy scoring categories” traditionally recorded have always served as imperfect proxies for players’ contributions or measures of discernable basketball skill. When we remove the artificial lens of the box score, what can be learned about describing and valuing not only statistical production, but the skills and abilities which allow for those achievements?
The NBA is in the midst of a quantum leap in the ability to address this question. The implementation of various visual, optical and biometric tracking systems allow for the game to be broken into much smaller pieces, giving insight into the game at what Brian Kopp of STATS, LLC., operator of the revolutionary SportVU tracking system, described as “molecular if not atomic level of detail.”
Though basketball analysis is often presented as a dichotomy between data-driven number crunching and eyetest-based narrative, there is no great uniformity within the field of analytics itself. The analysis publicly presented to fans often aims to prove who is better between any two individual players, but such “one-number” metrics are of little use to decision-makers within the league itself.
Some of the earliest forays into advanced statistical techniques were attempts to quantify players’ contributions in this holistic way. For example, metrics based on manipulation of on/off and play-by-play data (such as various adjusted plus/minus systems, which attempt to determine the value of players’ contributions controlling for teammates and opponents) were created, tested, refined and tested some more. But while these measures have helped advance understanding of overall player skill in broad strokes, they have yet to gain much in the way of mainstream traction.
One reason for this slow adoption is the relative complexity of the mathematical and statistical techniques involved. Discussion of players that requires a thorough understanding of the intricacies of ridge regression analysis and random forests is going to render only a tiny sliver of the population qualified to comment at all. This selection includes very few people with a similarly thorough understanding of the game itself — that is, basketball played on the floor, rather than just on a spreadsheet.
Similarly, integrating these metrics with coaching or scouting is often an exercise in futility. If the analysis controls too much for context, how is a college scout to identify a prospect capable of “APM-enhancing” plays, or a coach supposed to conduct a film session if analysts can’t tell them what to look for?
Finally, and perhaps more importantly, this one-number style metric provides little in the way of guidance towards the decisions actually facing franchises. Any given APM-style model may suggest that Kevin Love is producing at a higher rate in Minnesota than Chris Bosh is in Miami, but the analysis is so context-dependant in terms of role, scheme and teammates that conscientious practitioners would be loathe to claim anything about what this data point has to say about what would happen to the fortunes of either team if they were magically swapped. To put it another way, the very top level view provided by “one number” style analysis (whether APM, PER, Win Shares, etc.) comes closer to answering questions about what has actually occurred on the court, but it tells us very little about the hows and the whys.
“[APM-style metrics] are great if you’re trying to infer something for which there is no data. They’re absolutely useless if you have some or all of that data.”
This favored online pastime of ranking every player in a vacuum is simply not a question faced by many or even any NBA teams. Instead, each franchise faces its own unique set of decisions that drive its data needs. If a team needs a point guard, they probably won’t search for the platonic “best” such player, but the player whose range of skills and talents best fit within their existing team in terms of the areas of need and financial or salary cap flexibility.
As one practitioner in an NBA front office told me “[APM-style metrics] are great if you’re trying to infer something for which there is no data. They’re absolutely useless if you have some or all of that data.” Asked if SportVU fills in those gaps he replied: “Absolutely. Player tracking identifies specific defensive stuff, which obviates the usefulness of APM.” This “defensive stuff” not only highlights which players are doing valuable things on the floor, but starts to clarify what those things are.
Instead of answering the one big question (“Who is better in a vacuum?”) these emerging, more finely-tuned methods of analysis are addressing several smaller problems with much greater specificity (“How many points per possession is Kevin Love’s rebounding worth over Chris Bosh? How much is Bosh’s defensive rotation and rim protection worth relative to Love?”) In exploring these more specific queries, the new tools and technologies are beginning to show their worth.
Apr 4, 2014; Miami, FL, USA; Minnesota Timberwolves forward Kevin Love (42) makes a three point basket as Miami Heat center Chris Bosh (1) defends during the second half at American Airlines Arena. The Minnesota Timberwolves won in 2 overtimes 122-121. Mandatory Credit: Steve Mitchell-USA TODAY Sports
The New Players
As most ardent followers of the NBA are by now well aware, advances in analytics are being driven in large part by the wholesale adoption of the SportVU camera and optical tracking system. Originally designed to record and follow the positions of all 22 players and the ball on a soccer pitch with a combination of high-tech cameras and software adapted from optical tracking of ballistic missiles, STATS acquired the intellectual property to the SportVU system with the intent of not only expanding their reach in European and MLS soccer, but also to redesign the system from the ground up to optimize its use for basketball. While the existing setup for a soccer game involved three tripod-mounted cameras, STATS’ research revealed the need for more camera positions, fixed throughout an arena given the much smaller court on which basketball is played as well as the large amount of time spent with many bodies contesting the even smaller key area. After being installed in around half of the NBA arenas through last season, STATS contracted with the NBA shortly before the start of the 2013-14 regular season to install the system in all 29 arenas and provide content gained from the SportVU system to NBA.com.
In addition to STATS, LLC’s own research team and the proprietary work done by individual franchises, numerous other research groups are pouring over the SportVU data to see what insights can be gleaned. One such group is Second Spectrum, a combination of academic think tank and analytics consulting firm operating out of Los Angeles. Founded by USC professors of Computer Science Rajiv Maheswaran and Yu-Han Chang along with Chief Technology officer Jeff Su, Second Spectrum produced their second award-winning Sloan Conference paper in three years in 2014, detailing what they describe as “the three dimensions of rebounding.” Essentially, Second Spectrum’s approach is to estimate the likelihood of every player on the floor securing a rebound given the position of all ten players and the location of the shot attempt, and calculating rebounding skill using that positional value as a baseline of measurement.
And the data collected by SportVU is not the only game in town. Vantage Sports, a startup founded by former Mergers and Acquisitions attorney and one-time member of BYU’s basketball team Brett McDonald, is attempting, through a proprietary combination of video analysis software and human charting of game film, to catalog every game in the minutest of detail.
The work of these groups both in producing results of their own and providing technology, data and foundational research for the broader analytics community seem on the verge of rearranging our fundamental understanding of the value of actions on the NBA basketball floor.
Molecular Chemistry
The finite pieces of information gleaned from SportVU, Vantage and third-parties utilizing these technologies have certainly whet the appetite of the online analytics community. Rarely a day goes by without a call for more information, different presentations or methods of sorting already available numbers, or even access to the full data set.
“We had around six weeks from when the agreement with the NBA was announced to get something up and running by the start of the season. We had a lot of ideas and eventually settled on the nine reports you see on NBA.com. We wanted it to be both informative and accessible, and we’ve been mostly successful on both fronts.”
Brian Kopp, Senior Vice President for Sports Solutions of STATS, rejects the premise that there has been anything slow about the rollout of SportVU-related statistics. “We had around six weeks from when the agreement with the NBA was announced to get something up and running by the start of the season. We had a lot of ideas and eventually settled on the nine reports you see on NBA.com. We wanted it to be both informative and accessible, and we’ve been mostly successful on both fronts,” said Kopp in a phone interview, referencing new Commissioner Adam Silver’s well-known belief that access to new analytics and data can be a driver of future interest and engagement with the league.
After the initial launch for the start of the 2013-14 season, STATS, LLC and the NBA have continued to work together to bring even more information to the fan. Shortly before the All-Star Break, with little fanfare, NBA.com added “player tracking box scores,” providing much of the same information already captured in the nine initial reports for each contest, while also providing some new metrics and data previously unpublished. (See here for a quick primer on the “player tracking box score.”)
“There is definitely some learning going on from our end, especially in terms of the experience of the two operators in each arena,” Kopp said. These operators are on hand to ensure the system is running properly before each game as well as to answer prompts from the system when the visual tracking data is insufficient to identify players. For example if none of the cameras can identify a player’s jersey number in a scrum in the paint, the system will require input from one of the operators to ID the player correctly. The operators’ main jobs are to sync the system’s output with the official NBA play-by-play.
“At this point, we want to give primacy to the existing record of the game to be able to tie events which are captured optically to larger, commonly measured game events,” Kopp said. “Maybe someday the play-by-play will be completely automated but we’re not there.” Synchronizing the optical data feed with the play-by-play seems to be the biggest choke point in the system at this point, as before it is cleaned up post-game, events often occur in duplicate or out of order in the running play-by-play as official scorers try to keep up with multiple events happening in quick succession. The “corrections” often necessitate updates and adjustments to the player tracking box score after a game concludes. This need for revision could reduce real or near-real time usefulness of the data, but improvements in both the system itself and the experience level of the operators will reduce this lag time according to Kopp.
Nor is this game-level detail the end of the plans for public presentation of SportVU-based data. During the playoffs, NBA.com ran daily articles based on SportVU matchup data. Even since the season ended, NBA.com has added team-level data for the season, which confirms that yes, the Timberwolves were the worst team at protecting the rim this season, and the Warriors surprisingly completed the fewest passes (by far) of any team in the league.
Though there is certainly more to come, don’t expect a data dump any time soon. “Part of the reason things are rolling out at the pace they are is that we’re spending a lot of time making sure we are capturing the component actions on the court correctly,” said Kopp.
In a way, their coders and researchers are attempting to create the alphabet which will serve to form the vocabulary with which to speak about the game, not unlike the periodic table of elements is a taxonomy of the building blocks of chemistry. Kopp agreed with this analogy, though preferred to compare the level of understanding to molecular rather than atomic chemistry.
“To understand how a certain player uses or defends a ball screen, for example, we first need to define what is and is not a ball screen, and we need to do so accurately,” Kopp said, perhaps alluding to a much maligned Sloan paper and presentation which detailed an algorithm with a success rate rate of 80% in identifying such events. That hit rate sounds good until you realize that means 1 in 5 instances are being missed, and perhaps missed in a systematic way which could badly bias the results in large and unknown ways. With millions of dollars and their own continued employment often in the balance, decision makers for NBA teams need more certainly than that. Hence STATS’ extensive focus on a thorough quality assurance process before releasing new data points.
Apr 13, 2014; Sacramento, CA, USA; Minnesota Timberwolves forward Kevin Love (42) runs up the court after scoring against the Sacramento Kings during the first quarter at Sleep Train Arena. The Sacramento Kings defeated the Minnesota Timberwolves 106-103. Mandatory Credit: Ed Szczepanski-USA TODAY Sports
Isolating the Skill Element
Kevin Love remains a somewhat divisive figure in terms of his stature in the league. While he’s a magnificent scorer and rebounder, to quote Zach Lowe:
"[H]e has shortcomings. He offers no rim protection, he lollygags in transition defense, he’s not going to make spirited second and third rotations on the same defensive possession, and he often fails to challenge shots in order to secure boxout position — and precious rebounds. Love wants his numbers."
The last bit — he wants his numbers — is one of the most salient critiques. Though probably overblown, many of the questions of his defensive effort relate back to his desire to secure rebounds. At the same time, rebounds are good! Defensive rebounds are a crucial part of defense. But how Love gets them matters, and even the basic SportVU stats publicly available don’t shed much light on what is a more or less helpful way to secure a rebound.
Enter Second Spectrum. The company’s Sloan presentation, “The Three Dimensions of Rebounding,” is an important first step towards a more refined understanding of rebounding. Combining SportVU positional data with a mathematical technique called Voronoi tessellation and using regression modeling to isolate the impact of separate skills, Second Spectrum has been able to parse rebounding into three subskills — Positioning, Hustle and Conversion.
Roughly speaking, “Positioning” measures the general likelihood of the player securing any given rebound given where the shot was taken and the locations of the other nine players on the court both at the time of the release as well as when the ball hits the rim. “Hustle” captures players’ ability to relocate to more advantageous positions while the ball is in the air. “Conversion” reflects how well a player secures the ball when it is in or near his area. (Second Spectrum has released a quick video primer on the methodology which explains in more, though not overwhelmingly complex, detail.) All three metrics are taken for players on both the offensive and defensive ends of the floor.
(sample images taken from “The Three Dimensions of Rebounding” showing division of court into “areas of control” for purposes of examining rebounding)
Applying this framework to Love, it appears that his rebounding totals are not primarily a result of simple basket-hanging. Controlling for initial positioning, Love was the 5th-ranked player in the league in terms of securing additional defensive rebounds over and above what his initial positioning might suggest. (The researchers note that the degree to which there is a skill element to positioning is unclear, whereas hustle and conversion are more obviously tied to individualized attributes.)
However, this does not completely answer the charges levied against Love — he also shows up high on the list of players with the highest “crash” rating for repositioning once the ball is in the air. As one of Love’s alleged demerits is his unwillingness to fully contest shots in order to focus on rebounding positioning, this high “crash” rating might not be a net positive when both initial defense and rebounding taken into account, as his repositioning to secure rebounds could well reflect a less than diligent attention to contesting the initial shot.
Even though the net effect of Love’s ability to “crash” the boards is left unanswered, it is a much smaller and more manageable problem than the much broader “How valuable is Kevin Love’s rebounding, really?” A next iteration of research can perhaps weigh the additional rebounds this crashing gains against the shots which he might have been able to better contest (In 2013-14, Love allowed 57.4% on shots at the rim he was in position to contest, well above the league average of just under 50% for big men, and he unsurprisingly grades out as one of the poorer rim protectors in the league.)
“We try to avoid mistakes by knowing which things we can’t know yet.”
The researchers remain relatively humble about the degree of certitude to place in their findings. Said Maheswaran, “We try to avoid mistakes by knowing which things we can’t know yet.” This approach, learning many little things about the game rather than searching for a grand theory of everything is a method much more likely to gain acceptance within the league. He continued, “We see the data as resource which the teams can put to any use within reason. The data is a big pile of wood. We aren’t interested in fashioning that wood into tables or chairs or houses; we provide teams the tools to do that themselves.”
Reverse Engineering a Video Game
If the SportVU system and researchers stop short of attempting to split the basketball atom, Vantage’s aims seem just that high. With a truly dizzying array of actions and interactions cataloged on every single play, the Vantage system seems to offer the ability to answer any conceivable question about how a player has performed in any imaginable scenario.
“It is a balancing act between what shows and doesn’t show up on one of our scouting reports. We can do a lot of stuff algorithmically, but it’s more about communication of meaningful insights.”
According to Brett McDonald, CEO Competitive Analytics Consulting, L.L.C. (the company behind the Vantage system), they consulted with experts such as Ryan Blake, Senior Director of Scouting Operations for the NBA. From those sessions “we essentially wrote out all the questions we still had about the game, and we started identifying the data that would be required to answer those questions. We didn’t believe the existing solutions could answer those questions and gather the right kinds of data, and thought we could do better.”
As an example of the truly microscopic level of detail capture, for every screen set on the court, Vantage codes various actions and outcomes for both the screen setter and the player using the screen:
(Screenshot used with permission from Vantage)
Similarly, every offensive touch is categorized across multiple phases of activity. The “pre-acquisition” phase (such as using a screen, making a basket cut or simply spotting up), what the player does once he receives the ball (number and type of dribbles) and an outcome (shots, passes, turnovers). Defensive activity is captured in terms of ball-pressure, hand position on shot attempts and metrics such as “keep in front %” capturing the ability to deny dribble drives. Rebounding includes boxing out or failing to do so. The locations on the floor where these actions occur are also recorded. All of this data leads to over 16,000 individual data points for each and every game. Responding to a comment that the data appears like an attempt to reverse engineer a basketball video game, McDonald chucked and admitted, “We think this data is going to be used by video game designers to make their games more entertaining and true-to-life.”
Vantage achieves this level of data with a combination of automated video analysis system designed by former Googler Cameron Tangey, along with human coding and charting of every game by one of Vantage’s 50-plus full time analysts. “Just using technology and not reflecting orientation or if a hand is up, you can’t get to the kinds of insights that we wanted to get to, so we built a hybrid system.”
Kopp of STATS stresses that part of the reason the raw SportVU data isn’t “all” publicly available is that a large portion of the work done by STATS (and along parallel lines, companies like Second Spectrum) is an attempt to thread the needle between capturing enough detail to identify all the nuance and skill differentiation required for an end user’s needs, without providing so much data and extraneous information as to become computationally unwieldy and organizationally unusable.
The level of granularity of the data Vantage gathers only exacerbates this problem. At one point during his demo of the system, McDonald drilled down into shots attempted by Michael Carter-Williams after he received the ball on a cut at or near the left elbow. There were about a half-dozen plays broken into four different sub-actions. McDonald readily admits that from an analytic perspective, this kind of overly narrow focus renders the data less than useful — understandings of context and sample size are a necessity for appropriate use of the platform.
For that reason (as well as cost, given the extensive manpower required to track this level of detail), Vantage is marketed almost exclusively to professionals employed by teams at present or to individual players, coaches or organizations in terms of a detailed scouting service. McDonald recognizes the latter is almost as much if not more about presentation than providing an exhaustive analysis. “It is a balancing act between what shows and doesn’t show up on one of our scouting reports. We can do a lot of stuff algorithmically, but it’s more about communication of meaningful insights.”
That’s not to say the Vantage system is not being used for more foundational research. Krishna Narsu, a Masters candidate in statistics at University of Rhode Island, is one of several researchers working with the system and underlying data set to glean insights into the game. “I was always interested in research concerning shot defense.” To this end, Narsu has produced such work as his examination of “The Intersection of Defense, Shot Location and Clock,” which preliminarily suggesting insights such as in general terms, even a contested 3 pointer is better than a midrange shot at any point until very late in the shot clock:
These are initial results, requiring extensive caveats. For example, the underlying data does not take into account the possibility that early shots are heavily weighted towards better shooters. The research is not nearly definitive enough to suggest a full adoption of D’Antoni-style “Seven Seconds or Less” offense.
Narsu also believes that one can account for “99 percent of variation in team defensive efficiency” by looking at a selection of 14 defined metrics measured by Vantage. These metrics capture things such as propensity to foul, ability to actively and fully contest shots, and ability to prevent shots in the paint. If this discovery proves accurate, the potential value to both coaches and general managers becomes more clear in terms of identifying what areas to spend practice time or what skills and player profiles the team should look to acquire (or in many cases, jettison.)
Though he doesn’t want to be labelled as a specialist in only shot defense, Narsu recognizes the depth of data collected by Vantage allows for limitless exploration: “We’re really just at the beginning of the research they [Vantage] can do.”
Jun 5, 2014; San Antonio, TX, USA; Miami Heat head coach Erik Spoelstra talks to his team during a timeout in the second half against the San Antonio Spurs in game one of the 2014 NBA Finals at AT&T Center. Mandatory Credit: Bob Donnan-USA TODAY Sports
Applications
In a way, the advent of these sorts of in-depth tracking and charting systems both advances and impedes the process of convincing often skeptical “basketball lifers” of the importance and usefulness of analytics. The minutiae of the technology or mathematical technique can either overwhelm or bore a non-practitioner. “The point isn’t the technology, the point is in providing actionable information in useful formats,” says McDonald. Advancement in the race to discover the natural laws of basketball is better left to the academics, as those in the league are much more concerned with competitive advantage.
Maheswaran agrees: “There are people who love basketball and like computer science and pattern recognition. They end up working for teams and in the media. There are people who love the computer science and pattern recognition aspects and very much like basketball and they end up working for us. If they didn’t they would be working somewhere in the research field.” So, to use Lowe’s phraseology, it will still be the “jocks” or the “basketball lifers” asking the questions. The “geeks” (to the extent this remains a separate group from the basketball lifers) will help provide the information needed to address these team-specific quandaries.
“If you track scoring and highlight scoring, everyone wants to shoot. But once we start tracking close-out rates and keep-in-front percentages, players will take more pride in those aspects as we can give credit or assign blame in those important aspects of the game.”
Certainly, more specific tracking of player tendencies will be one advancement. An example Kopp uses of an actionable piece of intelligence is the ability to measure how many of a player’s drives to the basket result from ball-screens and how many are the player simply beating his man off the dribble in isolation. Without sharing the specific list, Kopp allowed that LeBron James had the highest proportion of his drives without help from teammates setting screens, whereas Tony Parker was among the players who used screens most often to set up a drive. While keeping both players out of the lane is a priority for defenses, understanding how each player is able to get penetration is a key towards designing a game plan to counter these tendencies.
Kopp and McDonald both think the largest initial gains will be made in terms of cataloging and valuing defense. Some of this has already started to occur in terms of measuring rim protection or pick-and-roll defense, but much more is surely on the way in terms of recognizing and performing rotations, challenging shots on the perimeter and simply being able to guard one’s man. This new ability to highlight and measure defensive accomplishment potentially impacts all levels of the game, according the McDonald. “If you track scoring and highlight scoring, everyone wants to shoot. But once we start tracking close-out rates and keep-in-front percentages, players will take more pride in those aspects as we can give credit or assign blame in those important aspects of the game.”
Further, at least in the SportVU system, there is the ability to modify the information presented to fit the needs of specific coaching styles or organizational philosophies. “Initial data shows that across a wide range of shots, being within about four feet of the shooter makes a tremendous difference in terms of shooting accuracy. But if a coach thinks you need to be within three feet to fully contest a shot, we can easily change our parameters to get them that data,” said Kopp.
At the same time, the more finite and tangible the pieces of the game revealed by SportVU and Vantage, the greater the potential for intelligent study of the data to provide just the competitive advantage franchises are seeking. Perhaps Vantage can assist a team with shooters needing a screener to identify the best one on the market, or SportVU can help a coaching staff design a defensive scheme allowing weakside defenders the largest possible effect on the opposition by better illuminating how close a player must be to effectively challenge a shot. These small, incremental advances in understanding can combine the certainty of a large data set with the implementation of practical demonstration on a whiteboard or iPad.
Certainly, the increased use of tracking will improve the quality of data coming out of the college game and thus into the draft process. During the 2013/14 season, Duke, Louisville and Marquette (who share an arena with the Bucks) have implemented SportVU tracking. This advancement is in the nascent stages. Says McDonald: “Too early to tell” in terms of what of this more granular data translates from college to the NBA. “I think a lot of the teammate-independent metrics will be useful, but it’s just too early make any sort of judgments. That will be an ongoing project.”
Similarly, there there was an intriguing data point in Second Spectrum’s presentation given the abundance of players of general high quality on the list of those with top marks in ”defensive conversion,” that is, players most like to secure a defensive rebound in or near their “area” of the floor. In our conversation Maheswaran refused to speculate on whether this was capturing something tangible in terms of ‘Basketball IQ’ or this was simply happenstance. “We need to do a lot more testing to know if it’s something real.”
And it is not just personnel moves or big picture game planning which can be heavily influenced by this sort of data-driven analysis. A team or even an individual player can get a much better idea of specific skills or attributes they need to improve.
As an example, consider Ricky Rubio and his shooting percentage. While he’s a sub-par outside shooter (around 15th percentile of NBA players), where his shooting really nose dives is finishing around the rim, where he was dead last in the league. If he finished at a league average rate, suddenly his shooting becomes merely bad and not abysmal.
Identifying finishing as a main issue will allow Minnesota’s analysts to watch relevant video (either identified through one of these tracking systems or culled through the traditional video scouting and charting process upon which all 30 teams rely extensively) to examine how to improve this area of his game. Through this video study the Timberwolves or Rubio himself might recognize that the underlying issue is his lack of strength to play through or balance to avoid contact on his forays to the basket. Rather than spending a summer or more on the difficult task of rebuilding a not-terribly-broken jump shot from the ground up, Rubio can focus on strength training and agility drills designed to rectify this specific shortcoming. This could easily result in the most bang for the buck in terms of improved production relative to time put in.
Dec 7, 2013; Minneapolis, MN, USA; Miami Heat shooting guard Dwyane Wade (3) holds his knee in pain during the first quarter against the Minnesota Timberwolves at Target Center. Mandatory Credit: Jesse Johnson-USA TODAY Sports
Training, Recovery and Conditioning
Some of the initial SportVU data dump on NBA.com was not especially useful upon first examination. During the Basketball Analytics Panel at Sloan, now-Pistons’ coach Stan Van Gundy was bemused by some of the metrics. Discussing Paul George’s league lead in distance traveled as of the conference, Van Gundy pondered, “Of what possible use is that information?”
According to Kopp, a great deal. In fact the possibilities for tracking data that has him most excited relate to injury prevention and rehabilitation. Speaking of the newly formed partnershipbetween STATS and Australian wearable technology company Catapult, Kopp said, “We can really start to examine the loads these athletes are putting on their bodies, which has profound implications for suggested playing rotations, training regimens and even identifying players who might be injury risks when looking to acquire new talent.”
The most basic application involves scheduling appropriate levels of practice and training intensity. “A lot of trainers and strength coaches look one week at a time. Layering in the schedule and the travel days [trainers] can see how hard they should have a player go. You can start to see the accumulated load on the player throughout the week. Based on that load you could ratchet up or down what they do. It will tie into what [teams] are doing with guys coming back from injury or guys who might be becoming more prone to injury.”
Kopp is quick to say STATS and Catapult are not trying to usurp the discretion or judgment of existing medical staffs. “We’re very careful to say that we’re providing tools to decision makers. I never want to be the one to say this guy should play or shouldn’t play. That’ll be up to the organizations.”
On the eve of the playoffs, Kopp says STATS had preliminarily identified several players who might have poor post-season runs based on the underlying movement and acceleration data indicating who was simply not moving as quickly than earlier in the season. Though he refused to confirm as to whether Roy Hibbert (he of the notorious late-season drop-off) was one of those players, he did identify Tim Duncan as a player whose physical output showed few signs of diminishing. “Even a couple years ago he wasn’t able to keep his intensity level up [later in the playoffs]. He’s been great throughout the entire playoffs this year. Even playing more minutes, you’re not seeing the tail-off that you did a couple years ago.”
When we spoke during the Finals, he also noted that even early in that series it appeared the Spurs were far less worn down than were the Heat. He suggests the SportVU data can show “the miles, the distance, the minutes, the load on the bodies for each of these teams and how they’ve been managed over the season. It’s become a story because one team certainly doesn’t seem like they are tired and the other team does. The ironic part is the team that doesn’t look tired is the one people have people have been calling old for a while.”
Answering Questions
For all the amazing opportunities these reams of new data provide, their use is still very much in its infancy. Danny Ainge, GM of the Celtics recently downplayed the degree to which SportVU data was being employed: “You have to be careful with how you utilize the information that you have,” Ainge said. “It is sort of fun and intriguing and I understand why media and the fans are intrigued by it all, but I think it’s blown way out of proportion of how much it’s actually utilized.”
Some of this is the sheer newness of the technology. Over time practitioners can not only answer questions coaches, GMs and — increasingly — players might have, but can start to anticipate these needs and present usable solutions to existing problems.
Additionally, there is still a hint of technophobia within the ranks of decisions makers. More than one team has an expensive analytics department in order to say they have an expensive analytics department instead of relying on it for information.
Gradually, as both the analysts themselves and front office types in general become more familiar and comfortable with both the accuracy and usefulness of properly employed fine-grain data the use will increase. Much like the small, discrete improvements proper application of the data will allow, new techniques and modes of thought will be adopted piecemeal over time. We might not be able to identify the tipping point when it happens, but it will occur, even if only through the normal cycles of competitiveness, as teams can no longer afford to be left behind the in the race for improved information.