Gambling for Steals: What’s the expected payoff?
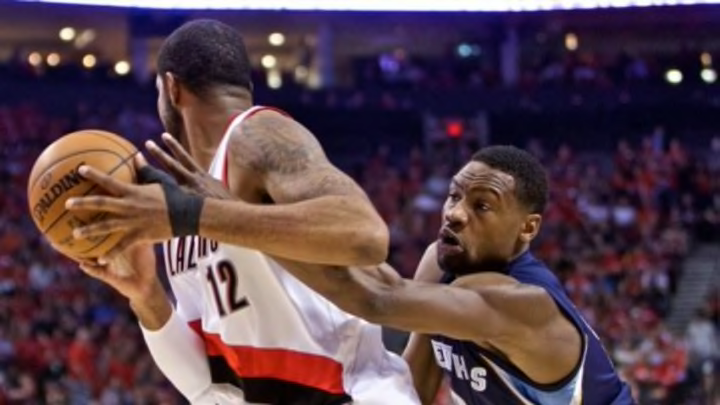
Everyone loves a steal.
Previous research has shown that steal rate is one of the most important stats for projecting NCAA performance into the NBA and one of the stats that is most correlated between the NCAA and the NBA. Furthermore, we’ve seen the importance steals can have in the NBA. Watch any steal turn into a fastbreak layup in the other direction and it’s easy to see why they’re one of the most important plays in basketball.
Others have looked at the value of the steal so I’m going to take a different approach towards looking at the data. Stealing the ball often requires some risk, trading a gambling defense play for a possibly bigger payoff. The new variables of the SportVU data can let us see in much more detail why steals lead to such high value offensive possessions. Which leads us to the big question: How do we quantify that risk of going for a steal? That last question is important and I’ll propose a framework for calculating that in the future.
Before getting into the value of the steal, let’s look at some of the ways shot selection changes immediately after a steal[1. The same thing applies as footnote #3 in this article: the steal directly precedes the FGA with no intervening action in the play-by-play logs.] versus all other situations[2. A bit about the data: I scraped play-by-play data from NBA.com and with the help of Seth’s pbp scraper, converted it into a usable format, which I then merged with the shot logs. This merged dataset is different from the one most of us have been using at Nylon as this was my own handywork. Unfortunately, because I’m not as adept a scraper/merger as Darryl, my dataset is a bit more incomplete. I wanted to be on the safe side, so I removed any duplicate shots from both datasets (pbp and shot logs). This meant that about 96% of the shots were retained. Given the way I merged the data- shots in the pbp had to be within 5 seconds of the shot logs- there were a lot of likely duplicate putbacks/offensive rebounds. For example, if a player attempted a shot, missed, got his own offensive rebound, missed again within 5 seconds, that shot would’ve likely shown up as a duplicate. I did merge by both points and FGM so any made shots are less likely to be duplicates since being a made FG would differentiate it from a missed FGA, even within the 5 second range. So we’re really talking about shots where a player missed twice within 5 seconds while getting his own offensive rebound. For reference, I merged the two datasets by game, player, period, made field goal, points, and the game clock being within +/- 5 seconds of each other. Given the removal of duplicates, this should produce a very accurate dataset that suffers from some data loss, most likely of the offensive rebounding kind. But this shouldn’t affect the analysis in this post.].
Using my aXPPS+[3. For details of the metric, see these posts. And for the actual model, see the footnote here.] methodology, shots directly after a steal are worth 20 points per/100 more than all other field goal attempts, 1.18 points per shot to 0.98. This effect is primarily due to early offense, as a steal leads both to more early and better early offense opportunities[4. 1.25 PPS in early offense off steals versus 1.09 PPS by aXPPS+.].
Unsurprisingly, shot location distribution changes significantly after a steal[4. Radar plot in R!]:
As expected, we see significantly more shots taken near the basket off of steals than in all other situations. But as Seth pointed out in this article, there’s not much benefit later in the shot clock after stealing the ball. After all, if you’ve gotten deeper into the shot clock, chances are the defense was able to get back and get set. So from now on, we’ll only be looking at shots early in the shot clock[5. Defined as 24-15 seconds remaining.]. However, given that about 75% of shots following a steal are early in the shot clock, the shot distribution doesn’t look much different:
Digging into each location category, the shots themselves are ore open following a steal vs. other possessions.
As expected, teams get more open shots following a steal from everywhere on the floor but the area between the rim and midrange, which likely constitute floaters over retreating defenders and similar looks.
We can also look at a few other stats, such as catch and shoot versus off the dribble.
What is interesting is that there is a significantly higher percentage of shots coming off the dribble in the less than five feet zone. Generally, these shots are
but in this case, it’s likely a lot of these off the dribble shots are actually dunks:
We can also look at the Assist% on shots following a steal versus all other shots:
As we see here, shots are uniformly assisted more following steals with the largest gap coming within five feet of the basket. Let’s look at Touch Time. Presumably, players are also holding the ball less on shots following steals:
How about who is shooting following steals? Are lower usage players more likely to be taking shots following a steal? How about more efficient players?
The usage and true shooting percentage of the player shooting following a steal is the same as all other situations.
Finally, let’s look at one last visualization that should drive home the point regarding the value of the steal.
An idea: What’s the expected risk of going after a steal?
Now that we’ve more fully explored the value of a steal, it becomes possible to model the expected risk of going after the steal. Unfortunately, we’re not at the point of plugging in numbers yet so for now, we’ll just introduce the formula.
Given the nature of the steal and how it can potentially lead to larger runs, for simplicity’s sake, we’ll limit the formula to two possessions: the initial possession of the opposing team where the steal occurs and the possession right after the steal where the defending team is now on offense.
Team A will be the team who goes after the steal while Team B will be the team who is currently possessing the ball and trying to protect the ball from Team A’s theft.
We can estimate the expected risk of a successful steal with the following formula:
Steal Success%[7. Unknown at this point but I would venture to guess that we’re close to having this data available.] x TeamA PPP_After Steal[8. Also unknown but unlike the previous variable, it’s probably possible to derive this from the play-by-play data.] – 0[9. The 0 points represent the number of points Team B scored on the initial possession. Since Team A stole the ball from Team B, Team B scored 0 points.]
And we can estimate the expected risk of a failed steal attempt:
Steal Fail%*(Expected points of Team A following current possession – Team B PPP_AfterWhiffedStealAttempt)[12. All of these variables are unknown.]
We can add this to the expected value of a successful steal attempt and then compare these totals to Team A choosing not to go for a steal.
(Steal Success%*TeamA PPP_After Steal-0) + (Steal Fail%*(Expected points of Team A following current possession – Team B PPP_AfterWhiffedStealAttempt))
vs.
No Steal attempt = Expected points of Team A following current possession – Team B PPP_SetDefense
where Expected points of Team A following current possession = (probability of FT + probability of FG make + probability of Deadball)*PPP of those events[13. which are all similar based on Seth’s article] + probability of steal*TeamA PPP_AfterSteal + probability of miss*PPP_AfterMiss.
Those probabilities would/could theoretically change based on if a player whiffed on a steal attempt. So for example, if Team A’s player whiffed on the steal attempt, was out of position, then it’s possible that Team B might offensive rebound at a higher rate because one of the players is out of position.
Unfortunately, given the public data available, we aren’t really any close to plugging in any numbers into the above equations. Although it may be possible to make some educated guestimates, which is an idea for a future post. But for teams who may have the data available, I’d definitely suggest running this analysis. Based on the high offensive value of a steal, going after the steal might be advantageous in all but the lowest “Steal success%” situations.