Bounded by Reality: Predicting Team Rebounding
By Justin
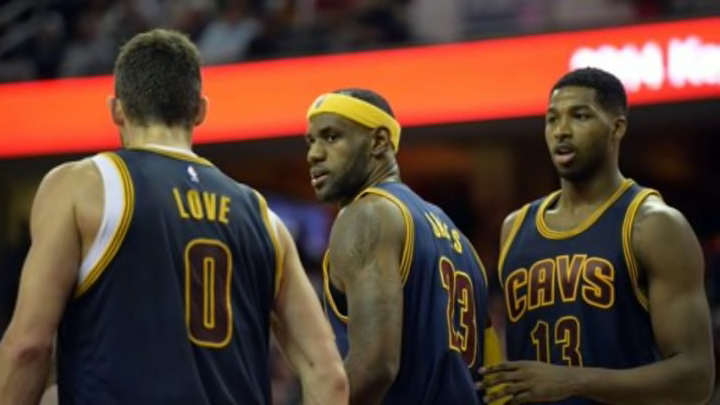
The NBA game is one of subtle complexity. These complexities can make it all difficult to analyze as there are five players on the court at the same time competing against five other players with just one ball. For exqmple, it is crucial to consider this sort of context when several big scorers are thrown together. It was largely predictable that the scoring stats of one or more of each of the “Big Threes” formed around LeBron James in Miami and Cleveland would take a hit. And that’s how it played out.
But there are other consequences to the rivalrous nature of many stats. Defensive rebounding is vital to a contending team and we all analyze rebounding totals for individuals, carefully considering every small edge. Yet individual rebounding is strongly controlled by role and how many rebounds teammates are grabbing. Players have a smaller influence on rebounds, especially on defense, than most people think.
The Form
Given how people discuss rebounding leaders, there’s an implied assumption that rebounding is additive. What does this mean? If you trade a player who averages 10 rebounds per game and replace him with one who averages 14, all things being equal like games played, your team does not net four rebounds in the exchange. Even if the new player averages 14 per game again, some portion of those four “extra” rebounds would have been collected by the team anyway. Elite-level rebounders are not only great at taking boards away from opponents but also from their own teammate. The converse is important though: if you lose a high rebounder, you don’t lose every rebound recorded by that player. This means that rebounding is nonlinear and has to be conceptualized differently.
If you want to see how extreme the effects are, try to predict how a team does for a season based on the past results of their individual players — and this is more illustrative when a team has a few new faces. For example, Cleveland last season brought in Kevin Love, LeBron James, and Timo Mozgov to a frontcourt that still had Anderson Varejao and Tristan Thompson. This frontcourt should dominate the league on the boards. You can predict the team’s performance with, say, defensive rebound rate based on how well the individual players rebounded in 2014, assuming average rates for rookies. The result you get should be somewhere around 85-86%, depending on how you deal with said rookies. In reality they had a DREB% of 74.7, which is only around the league average. The problem wasn’t just Love. Every player with major minutes with the exception of Mike Miller saw his percentage drop. This isn’t just about the players having an off year, of course. You aren’t going to get a normal team grabbing 85% of the boards because DREB% isn’t additive. It’s on a nonlinear scale.
The same is true of offensive boards, but the effect is smaller because there are more available rebounds for offensive players — or, in other words, less opportunity to steal. Plus, as offensive rebounding is less about a strict role, like having a set number of players box out and grab a defensive board, it’s more individualistic.
The simple explanation is that rebounding is bounded on both sides by some limits of reality — you can never grab more than 100% of the available rebounds and never less than 0% — and thus you need a different functional form to understand the results. The logit model is ideal for this scenario. For people who are familiar with diminishing returns, which has been studied in conjunction with rebounding before, this model captures diminishing returns well while controlling the lower and upper limits.
To properly show the behavior of rebounds, I extended the analysis I gave Cleveland in the preceding paragraph to every team and every season since 1979[1. For rookies, I used the average rate (reduced by about 10%) based on the player’s position, where positions were taken from basketball-reference.]. By reducing the prediction error[2. The sum of the squared errors.], I found an optimal form with the corresponding coefficients.[3. With some experimentation I had the most success with this general model:
100 / ( 1 + (1/league avg. reb. rate – 1)*e^(b1*(league avg. reb. rate – team reb. rate) ) )]
Essentially, what the function does is look at how far your team projects above the league average and then regresses hard to said league average in a logit model. Additionally, the projection is based on translated rebounding stats where players on good rebounding teams get boosts and vice versa.
The Results
What’s fascinating about team defensive rebound prediction is that the stats are regressed heavily to the mean. It’s so aggressive that you can perform decently well just by using the league average, which is, frankly, bizarre[3. The average absolute error was 1.4 when just using the league average, but improved only to 1.1 with the model.]. This is not an entirely new observation, but the information isn’t as widespread as it should be and it’s why most basketball metrics value offensive rebounds more than defensive ones.
Using the model, the typical range for the predictions every season is around 4%, which is roughly half the range for the actual results. While this suggests the stats trend to the mean with a bit of volatility, it’s also an indication that other factors are important, like team strategy — no matter how good of a rebounder you are, if you run back when a shot goes up you’re probably not picking up the board.[4. Here’s the full model for team defensive rebounding:
100/( 1 + (1/AvgDr – 1)*e^( 0.4867*(AvgDr – STmDr) ) )
AvgDr: league average defensive rebounding rate (0 to 1 scale.)
STmDr: sum of translated individual defensive rebounding rates (0 to 1 scale.) The rates are transformed by the factor of ( 1 + 9.68*(TeamDr – AvgDr))*DRB% where all rates are from the previous season.
and for offensive rebounding:
100/( 1 + (1/AvgDr – 1)*e^( 2.116*(AvgDr – STmDr) ) )
AvgDr: league average defensive rebounding rate (0 to 1 scale.)
STmDr: sum of translated individual offensive rebounding rates (0 to 1 scale.) The rates are transformed by the factor of ( 1 + 2.26*(TeamDr – AvgDr))*ORB% where all rates are from the previous season.]
If you don’t know how to interpret those models, that’s fine — there are only simple differences. Defensive rebounding stats are regressed heavily to the league mean while players on good rebounding teams get substantial boosts to their individual rates. While the coefficients are a few times smaller on offense, they still have an effect. Diminishing returns do exist, and generally players on good offensive rebounding teams are being underrated.
For an example, Cleveland in 2015 had a projected rate of 86%[4. This is with the translated individual stats], but the model transformed that rate to 75.9% compared to the 74.9% average that year. In fact, that large disparity can partly explain why the team under-performed overall. On offense, the projected rate was 27.7% and was transformed down to 26.1% where the league average was 25.1%. The adjustment is small, and in some cases you can get away with assuming the rates are additive, but the prediction power is stronger when you need it with the aforementioned nonlinear model.
Conclusion
What’s notable about the trends with rebounding is that defensive rebounding is stable and more of a team activity while offensive rebounding is driven largely by individuals. Witness Andre Drummond’s influence on Detroit, Tristan Thompson’s run in the playoffs with the Cavs, Gobert on Utah, and Kanter on both Utah and Oklahoma City. Yet defensive rebounding is the one strongly correlated with winning and offensive rebounding is actually correlated with losing teams.
With this method, it’s tough to parse how players actually have effects through rebounding. It’s possible that missing information is to blame — boxing out, for one, and separating rebounds by types. Better teams push shots away from the basket, and those rebounds near the rim are the easiest to collect on offense, changing a team’s defensive rebounding stats. But it’s still important to understand how the numbers interact and what influence they really have.