Guest Post: Dispatches from NESSIS 2015
By Seth Partnow
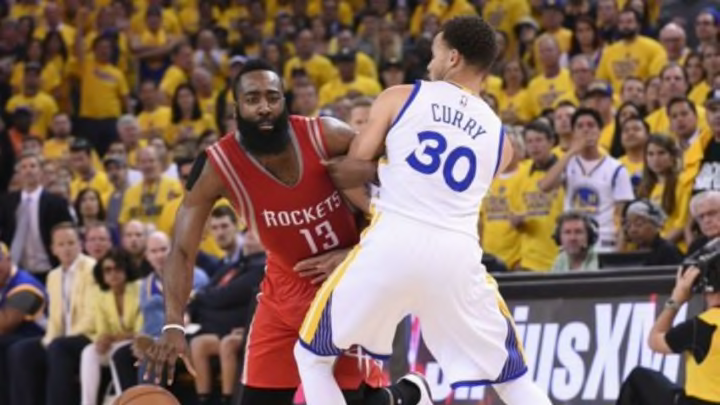
(The 2015 New England Symposium on Statistics in Sports was held this past weekend in Boston. Will Schreefer was there, and fills us in with this guest post. Will lives in New Jersey, where he works and studies as a civil engineer. He does some college basketball stat work on the side[1. Including a future Freelance Friday piece!], and is always sure this is the the year Villanova makes it past the first weekend.)
This past Saturday, the biennial-since-2007 ‘New England Symposium on Statistics in Sports’ – or NESSIS – was held at Harvard University’s Science Center. The brainchild of Mark Glickman and Scott Evans, distinguished New England-based academics with a passion for (and serious expertise in) the intersection between sports and statistics, the conference is characterized on the program as ‘a meeting of statisticians and quantitative analysts connected with sports teams, sports media, and universities to discuss common problems of interest in statistical modeling and analysis of sports data.’ Describing it, though, is as simple as ‘heaven.’ Heaven, at least, for that population slice who’d want to hear Jeremias Engelmann explain how to recreate Adjusted +/- and RAPM for a pickup basketball game and then take questions on his choice of ridge (Tikhonov) regression vs. ordinary least squares regression from an audience mostly composed of people who know exactly what the choice means.
Though the scale and popularity of NESSIS, as a Boston/Cambridge-based sports analytics conference, runs a distant second to the annual Sloan Sports Conference, it maintains a personal, accessible, and academic feel that a gathering of over 3,000 people in a convention center (with ticket prices set at $575) may not be able to. With low ticket prices ($25-$50 for early registrants!) and content aimed at and generated by students & the academic crowd, the gathering reads more as a somewhat informal academic conference than an event. The format encourages audience participation and informal mingling amongst all participants (presenters, attendees, sponsors) throughout the day. The presentations are given in an open lecture hall, with spaces for questions allotted at the end of each, and poster projects are set up just outside for casual viewing all day – with a space toward the end for interaction with the people who generated them. Topping it all off is a post-conference gathering at a local bar, open to all registrants (240 this year).
One of the only ‘drawbacks’ of the conference is the simultaneous presentation schedule in the afternoon, making it impossible (for a single person) to see all presentations in full. So the notes presented are on those I was in attendance for – for anyone interested in checking out presentations not detailed here, records of the all of them be posted sometime in the (relatively – I’m not positive of the timeline) near future on the conference’s website. Archives of past conferences are also available at the same location.
A few notes on the general proceedings, with an emphasis on the NBA/basketball-centric posters and presentations:
- Dan Cervone opened the basketball-centric presentations with an intriguing look at the ‘value’ of court space. Framing the value of ‘space’ on a basketball court as an analog to the real estate market (as people are willing to pay a higher price to live near things that are more ‘valued,’ basketball players will behave in the same sort of way in their occupation of specific areas on either end of the floor), the analysis went through the development and refinement of parameters determining typical player ‘investment’ (area covered) in a piece of a basketball court, and the ‘property value’ of those same squares. The analysis included all on and off-ball players, and analysis of the space they occupied. The valuation is currently connected to possession outcomes – good results when players are occupying specific areas of the floor. As a first pass, while there are certainly improvements/refinements to be made (as acknowledged in the presentation), it presents an interesting method to characterize the value of being in a certain area of the floor –a way to characterize the value of spacing, on the offensive and defensive ends, in a way that uses the true positions of each player on the floor (rather than a proxy, like 3 point attempts/percentage).
- To draw a parallel to another research project presented at the conference – especially on the defensive end, the value of denying optimal passing lanes is undeniable, yet exceedingly difficult to quantify given current available data. The ‘Man in the Middle: Optimal Defensive Strategies and Disrupting Passing Networks in Soccer’ poster made a pass at understanding optimal defensive strategies (in denying passing lanes/disrupting passing networks) in soccer. While it remains difficult to parse exactly how and why teams are able to disrupt passes (in soccer or basketball), combining player tracking information with lower resolution data, like turnovers, baskets, etc., can provide potentially important insights into overall strategies.
- Jeremias Engelmann’s presentation, essentially, was a ‘how-to’ on building adjusted plus/minus, RAPM, and Four-Factor RAPM models, framed in the context of a two-player basketball game. While most readers (and attendees) are certainly familiar with the measures, it was great to have a step-by-step ‘de-black-boxing’ (though, it should be noted, it can certainly be argued they’re not quite black-boxy – just unknown to many people) of the development of the noted metrics. No word on RPM, though.
- Several of the talks centered around bringing statistical methods and strategies commonly seen in other fields to bear on basketball analysis. For example, Weihua An’s social network analysis of NBA teams utilized a tool often seen in sociology to isolate some of the reasons certain franchises beat others. And Steven Mintz’s presentation focused on clustering methods often used in bioinformatics to group play types as detailed in the Synergy Sports data set.
- Speaking of ‘Exploring the Effectiveness of NBA Play Types with Synergy Possession Data’ – it wasn’t exactly what I had expected, based on the abstract – though it’s more than possible that’s due to not-close-enough reading by me. Ultimately came away intrigued with the potential of the approach as the application becomes broader – the analysis of the presentation specifically focused on pick and roll ball handler possessions, as described in the more-granular Synergy Sports play-by-play datasets. Using clustering techniques, these possession descriptions (which ignored defensive reactions & focused on guards/wings with larger samples of the possession type) were grouped by effectiveness & similarity of sequence. Spoiler alert: Steph Curry & James Harden run pick and rolls in a unique way (and better!) when compared to most other players.
- Part of what this presentation highlighted for me is what still remains to be mined from the NBA datasets available to both public and private endeavors. There are scripts that allow just about anyone (motivated enough to do it) to pull player movement data from publically available SportVU data, and yet there’s still plenty to be unpacked from historically available play-by-play logs. The data used in this particular presentation falls somewhere in between the complexity of those two, and remains a fertile ground for future exploration due to the detailed level & language used to describe possessions by Synergy.
- It’s tough, at first glance, to not be skeptical of an automated general manager that, backtested, outperforms every single front office in the league over the past 10 years in the draft (as measured by ‘Wins Made’ and other metrics). However, Philip Maymin (of Vantage Sports) provides all the technical appendices indicating it indeed does at nbagm.pm. While there are a few oddities in there (mainly with regard to international players, which weren’t projected/drafted by the model), I believe the paper/project/poster showed the strength of relying on consistent models that incorporate all available information to make decisions. Making draft choices based on the same framework – especially one as rigorously constructed as what was used – from year to year, and ALWAYS taking the best player available regardless of fit, will typically have better results in the aggregate than a more haphazard & subjective approach. Such a framework can be used as decision making tools for all front offices, as a decision ‘checker’ or key part of the process.
- I had to laugh at the noted inspiration for ‘Player Development in the NBA,’ (Harrison Chase, Nathaniel Ver Steeg, and Daniel Smith) – an Andrew Sharp quote from an article on the Philadelphia 76ers, specifically regarding their lack of veteran leadership (and that it was a bad thing). The project focused on testing for more intangible nodes like ‘veteran leadership’ and ‘playoff experience’ to determine if there were any statistically significant effects on player development. While playoff experience appeared significant in predicting player improvement (relative to the typical aging curve), veteran leadership was not so lucky. Sorry, Wolves.
- There were several other presentations and poster projects that focused on basketball, and a host of others concerning other sports and some more macro-sport-centric concerns. I highly recommend checking out the abstracts (and presentations, when they’re up!) on NESSIS’s home page – certainly do not want to give them short shrift.
Generally, NESSIS presented a great opportunity to meet and listen to a lot of really smart people with a passion for sports analytics. Representatives from sponsors like ESPN Stats & Info, the NBA, and DataRobot were also there, all looking to interact, provide info, and collect resumes. People interested in attending the conference in the future can wait til 2017, when another iteration of the New England version will take place, or look into the rumored 2016 ‘analog’ to NESSIS coming to the Pacific Northwest.