Introducing Positional Versatility Score
By Guest Post
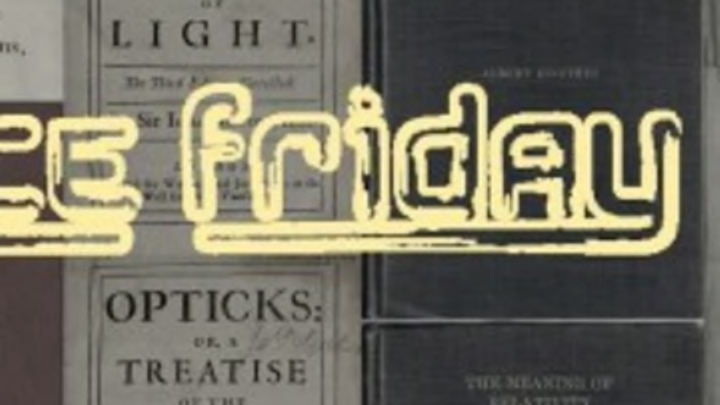
Freelance Fridays is a semi-regular series on Nylon Calculus where we solicit and publish basketball analytics work submitted by readers. (Occasionally we get so excited about a post like this that we can’t wait until Friday.) This week’s edition is from Michael Chang (@michaelofchang) and examines the idea of positional versatility. Michael has been double-checking boxscores since he became a sports fan in middle school. He still tracks play-by-play stats even when at a game. Currently in New York City, he enjoys deconstructing basketball personnel moves and on-court strategies.
Questions, comments or submissions for Freelance Fridays should be directed to TheNylonCalculus at gmail dot com
Last season, LeBron James questioned the meaning of “valuable” when Stephen Curry won the MVP award. He suggested valuable might mean all-around dependency on a single player as opposed to say, overall individual statistical brilliance — in other words, versatility with quality. With the modern game encouraging smaller lineups and more homogenous skill sets, is “versatility” becoming more of a premium attribute?
Versatility is not really addressed specifically in any public metric. The issue is arriving at a definition for versatility. Ultimately, there are two dimensions:
Position playing time distribution: Playing more positions is better but more importantly is an even distribution of minutes. A 90/10 minute split between two positions is not as indicative of versatility as a 50/50 split between two positions.
Performance by position: This is correlated to No. 1 as coaches will probably play players according to their skills, but it also has its own variance. The ideal most versatile player allows a coach to just put his best players on the court and not worry about fit.
The first element can be unpacked using 82games.com’s position performance data for 2015-2016. Here is what a typical position profile looks like (Dirk Nowitzki in this case).
This table tells us Dirk played 52 percent of the Dallas Mavericks’ power forward minutes and 6 percent of the center minutes, along with how the team performed with him at each spot. This data is not weighted for environmental variables like opponent strength, teammates/roster context, which could influence some positional data but more likely is muted through a whole season. For our purposes here, players who played on multiple teams were excluded from the data set due to varying acquisition times and what they mean for total minutes. Those players represent 7 percent of the total minutes played last season so the sample population is still very robust.
Position distribution
Let’s start by looking at how many positions most players play:
The average player plays 2.25 positions with a standard deviation of about .75 so about 70 percent of NBA players play two to three positions. Looking at the extremes, there are about 120 one-position players and only a small minority of players can play four or five positions. Next, let’s look at which positions are played the most:
This indicates that point guards and centers are the most specialized while 30-60 more players show up at the swingmen (hence the name) spots. This distribution reflects what we see in terms of physical player makeup (the average height of a NBA player is most like a shooting guard-small forward) and on the court (point guards and centers have more specialized roles with offense facilitation and rim protection, etc). It is interesting to see fours spike a bit more — possibly with teams playing traditional small forwards at the four spot more in smallball lineups.
Let’s go a little deeper and look at the distribution of position minutes by player appearance.
If a player plays 100 percent of their minutes at point guard, they are at the top left of this chart. If they play 50 percent of their minutes at SG and 50 percent at SF, they show up twice, once at .5 for the SG column and once at .5 for the SF column.
The main takeaway here that the specialist positions (PG, C)’s central tendencies drift towards 100 percent whereas the swingman positions (SG, SF, PF) are more diversified. This boxplot’s averages (the purple lines) are the opposite horizontal reflection of the prior bar chart, which makes sense as more players play a position their minute share of that position becomes more diluted.
Versatility measures
A standard measure of equality is the Gini coefficient, which is commonly used to measure things like income inequality in a country. The Gini coefficient can be adapted to basketball position minutes to see how much a player’s minutes are concentrated in a particular position, which can be contrasted to the ideally versatile player (who theoretically plays all positions evenly).
The resulting difference between the actual player and ideal versatile player is a position versatility gap/score, that can be ordered, analyzed and then mapped to other statistics. The higher the position versatility score, the worse, like golf. It means less positional versatility.
Players with a position versatility score (PVS) of two are one-position players and the more a player’s minutes were spent outside their primary position, the lower the PVS. A zero would be the unicornesque five-position player. Let’s see how PVS stacks up vs. a player’s primary position share of minutes to verify its accuracy:
The metric looks pretty stable, rewarding those with extra position diversity (those points that drift to the left) but does a good job of capturing how much a player is spending in one position. The overall spread of PVS is about 1.25 to 2. Since the points are bunched together at the cliff, let’s see what the spread of versatility scores look like:
The histogram shows a spike in PVS for 2, which represents single-position players, which is why it is so high. However, the bulk of the rest of the players appear to score a PVS between between 1.6 and 2, of which there seems to be an even distribution. This indicates that the range of individual player versatilities is pretty stable outside the one-position players. Let’s see how the PVS does against vs. net plus minus total:
While there isn’t a singular conclusion, there are some interesting patterns. We see that PVS is relatively well distributed across good (above 0) and bad players (below 0), meaning that a good PVS, or being versatile, doesn’t automatically make a player good or bad. The dots do seem to converge vertically on the left side of the chart indicating that low PVS players may have a more neutral but more predictable performance. On the other hand, there appears to be a wider range of performance on the right side of the chart, indicating that higher PVS, or less versatile, players have more fluctuation to their performance.
This could be a volume issue, so here is the same graph but with the Y-axis changed to net points per minute, making it a pure efficiency measure. Other than revealing a few low-performers in the specialists group (this is mostly due to smaller sample size), the main body of the scatterplot looks similar to the prior. The lack of low-performers on the left side might indicate that a more versatile player’s floor is higher.
Overall, PVS vs plus-minus is still a bit spread out. By putting players into PVS buckets (one-position specialists and then splitting the remaining sample evenly), the differences in net points ranges become more visible.
In the boxplot, one-position specialists break apart from the less-specialized players and become relatively predictable performers. This makes intuitive sense as they are either stars with firm roles or have more situational skillsets (think Andrew Bogut or Tiago Splitter) that can be deployed when appropriate. Versatile players (defined as having a versatile score below 1.75) look to be as predictable as those one-position specialists.
Finding predictable performers is very valuable for competitive teams. The 82-game season can result in 250+ possible lineup combinations for each team. Players who can play multiple positions are an advantageous asset for dealing with injuries, foul trouble and winning chess matches with opposing playing styles. The same advantages are offered to the front office. Predictable players de-leverage riskier transactions and are easier to price value from a free agency or trade standpoint.
A peek at the players with the best (lowest) versatility scores on the left reveals a not so sexy list (eight of the top 10 have a negative plus-minus per minute or pmPM) but that is not surprising given versatility isn’t necessarily correlated with a positive plus-minus as seen by the earlier scatterplots.
When you do factor in plus-minus, like those on the right, you see a different kind type of player appear than those with just a pure high plus-minus. Aldridge stands out but for the most part, there are players who can wear many hats and who can play in different types of lineups.
Interestingly, Draymond Green did not appear on this list. Draymond’s PVS is 1.77, which is solid but not exceptional. The reason is he played about 76 percent of his minutes at the power forward, which is right around the 50th percentile for % of minutes for your primary position. Draymond also had no minutes on the wing or at point guard. By comparison, Aldridge was almost a 50/50 split almost between power forward and center.
One other reason Draymond gets an outsized impression of being versatile is he played 2700+ minutes last year, which means he played nearly 700 minutes at center, forming a strong impression. Aldridge, with his near 50/50 split, logged about 1000 minutes at center by comparison. This is one of the two extra layers to versatility I alluded to in the article — being versatile with volume against just being versatile.
Related Story: Player Tracking Plus-Minus 2015-16
Also, five of the top six were Spurs/Warriors! This is a bit of self-selection as we filtered positive plus-minus, but this suggests versatility can help us identify specific key players for winning teams, or perhaps system-oriented vs star-oriented teams.
As mentioned in the beginning of the article, capturing the second half of the equation (performance by position) would be more useful than a net plus-minus. Having both position appearance distribution and position efficiency distribution would allow us to spot players who play multiple positions and play them equally well easier. It would also be interesting to see how PVS correlates with salary, other statistics (which statistics are leading indicators of versatility, likely not blocks for example) and by team (do winning teams feature more versatile players?). Stay tuned for more work on some of these issues.
At the end of the day, GMs and coaches are looking for margin values and tracking versatility quantitatively, like through PVS, may grant them an edge in predicting player performance.
Notes about analysis:
Translating minute percentages to actual minutes results in two small roundings: 1) the percentages are whole numbers so there will be some minor rounding errors. 2) To convert position percentages to total minutes, 3970 minutes was used, which is slightly larger than 3936 (48 MPG * 82 games). The real minutes played per player depends on the number of over-time games a team. Both changes should be <1%.