Nylon Calculus: Over and under-performing expected win totals
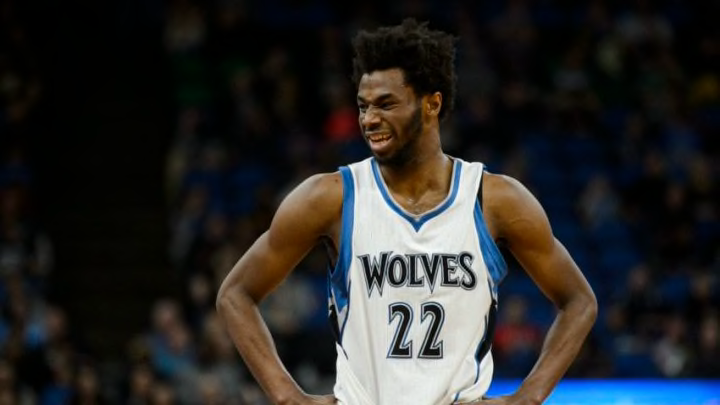
&nbPrior to their eventful offseason, the Minnesota Timberwolves had an interesting 2016-17 campaign under new head coach Tom Thibodeau. Their 111 offensive rating and 112 defensive rating nearly broke even, and their total point differential of -91 ranked 20th in the league. These numbers, though underwhelming, suggested a 38-win season. Yet the Wolves finished with just 31 victories, seven games below expectation and six games behind the Detroit Pistons, whose net rating (-1) and total point differential (-92) were practically identical.
Obviously, contextual factors helped shape the final standings. Minnesota, for example, played in the West and had the fifth most difficult schedule in the NBA, while the strength of Detroit’s predominantly Eastern Conference slate hovered below league average. Nonetheless, a seven-win gap still seemed noteworthy. How often does a team see such a discrepancy between expectation and performance?
Before answering this question, let’s establish how expected wins are typically measured. The standard approach is to apply the “Pythagorean formula,” a fundamental concept in sports analytics. Despite its intimidating name, this formula simply estimates expected winning percentage based on a measurement of team strength that involves points scored and allowed. When compared to actual records, expected wins help us identify overperforming and underperforming teams, which make for interesting discussions among analysts who are looking for potential regression and bounce-back candidates.
Although the idea is fairly intuitive, results of Pythagorean calculations can vary based on the chosen inputs. For instance, the standard formula uses total points scored and total points allowed, but offensive and defensive ratings are appropriate alternatives. Even more critical is the exponent. While Basketball Reference uses 14, ESPN prefers 16.5 — a number that is supported by previous research from Dean Oliver and John Hollinger. Different methods can also be employed to determine the exponent. The conventional approach is ordinary least-squares, but as noted by Christopher Long, the Bradley-Terry model can serve as the basis for an estimate.
This article relies on the Basketball-Reference formula for a few reasons. First, a 2010 article in the Journal of Quantitative Analysis in Sports determined that 14.05 was the optimal exponent. Justin Kubatko found a similar result three years later, concluding that “unless there is a major change in the way the game is played, using a Pythagorean model with an exponent of 14 is just fine for the modern era.” More recently, John Chen and Tengfei Li provided additional evidence in support of this exponent (although they also pointed out that a lower number would be better for predicting future win percentages).
With the Basketball Reference formula at hand, we can calculate the difference between expected and actual wins for every team in the 3-point era. A positive result means that a team outperformed its Pythagorean expectation; a negative result means that a team underperformed its Pythagorean expectation.
Between the 1979-80 and 2016-17 seasons, approximately 12 percent of teams earned as many wins as expected. Roughly 60 percent were within two wins and 94 percent within five wins in either direction of their Pythagorean expectations.
What if we focus on the modern NBA? A closer look at the data since the 2001-02 season (when illegal-defense rules were first eliminated) yields similar results:
During this time period, about 14 percent of teams had actual wins that matched their expected wins. Approximately 62 percent were within two wins and 94 percent within five wins in either direction of their Pythagorean expectations, mirroring the outcomes for the 3-point era overall.
In light of this context, we can revisit the 2016-17 Timberwolves and see how uncommon their experience was. They are one of only seven teams in the modern era and one of only 18 teams in the 3-point era to finish seven or more wins below expectation. In other words, only one percent of teams in the modern era and two percent of teams in the 3-point era have finished short of their Pythagorean wins to the same degree.
The 2016-17 season had other noteworthy results. As the following table shows, the Charlotte Hornets were right around the Timberwolves at six wins below expectation, while the Boston Celtics and the Orlando Magic were on the opposite end of the spectrum at five wins above expectation.
As the upcoming season inevitably sees its share of overperformance and underperformance, this table can serve as a resource for helping us gauge how significant they might be.