Nylon Calculus: Donovan Mitchell, Brandon Ingram, and lessons in the development of NBA players
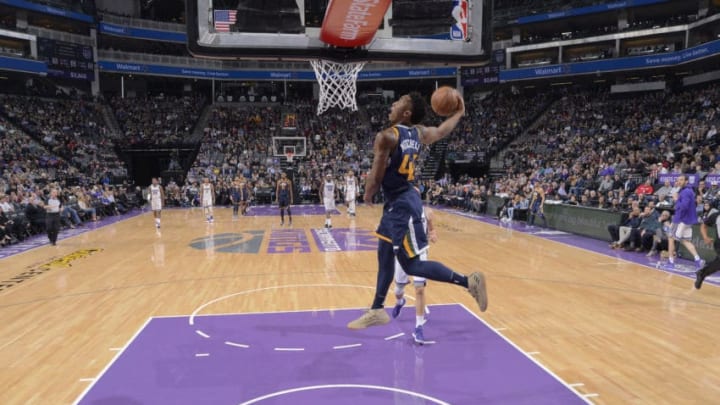
Coming out of college, Brandon Ingram was getting compared to Kevin Durant. At the end of his rookie season in the NBA, he was being labeled an outright bust. Now, midway through his sophomore campaign, Ingram is slowly coming into his own, and the outlines of a really good two-way wing are beginning to take shape.
On the other hand, Donovan Mitchell has been an absolute revelation for the Utah Jazz right out of the gate, especially on the offensive end. After losing Gordon Hayward, the Jazz entered a retooling phase, but Mitchell has looked every bit the part of a future superstar and made the process of coping with Hayward’s departure much easier than Jazz fans could have ever imagined.
NBA fans have notoriously low patience and tolerance for young players, complete with extremely reactionary tendencies. We all want our favorite team’s youth to be challenging for Rookie of the Year from the get-go, more so if they’re a high draft pick. Of course, the reality of the situation is often different. There are basically an infinite number of variables that affect the development timelines of various prospects. Mitchell is 21 years old; Ingram was 19 as a rookie. Mitchell currently has a usage rate of 28.8 percent; Ingram was at 16.8 percent as a rookie. And so on the list could continue.
Read More: Week 13 in Review — Lou Williams and the Rockets creative spacing
The contrasts in the beginnings of both players’ careers provides a useful backdrop to step back and try to distill the fairy dust that is the draft into some slightly more concrete and (potentially) scientific components. Player development is not an exact science, but that doesn’t mean that there isn’t room to find some patterns and analyze certain trends. Inspired heavily by Namita Nandakumar’s work on prospect development in hockey, I used survival analysis to see just what some of those overarching trends might be.
Survival analysis is a field of study used to essentially measure the life cycle of various entities, be it demographic (how long do various types of governments last?), biological (how long do certain diseases incubate?), sociological (what factors affect the timeline of recidivism?) or anything in between. This actually feeds nicely into the field of sports, where we can temporally model discrete milestones for player development as “survival.” Within basketball, I’ve found a few previous instances of survival analysis for various purposes. Katherine Evans used survival analysis early last year to model the accrual of personal fouls in an NBA game. More closely related to what I’m attempting to observe, a paper was published in 2012 using survival analysis to look at the overall duration of player careers.
I won’t get into the nitty-gritty math behind survival analysis (if you’re interested, the documentation for the lifelines Python package does a really good job), but there are a few things to note. I will be starting off by modeling survival curves using the Kaplan-Meier method, which is one of the most popular and widely used estimators for survival functions. I chose to use a dataset of first-round draft picks from 2013-2015, largely to avoid the variability that by and large accompanies second-round picks in the NBA.
The milestone that I chose was Basketball-Reference’s Game Score metric, a rough encapsulation of single-game productivity. More specifically, I will be looking at how long it takes players (if at all) to reach certain amounts of games with a game score of 10 or greater (an “average game” baseline), by number of regular season games since being drafted (which means redshirted seasons are counted). While there are issues with game score (and certainly if you conflate it with true skill and impact), it does provide a solid publicly available game-to-game metric for our purposes. So let’s put a visual to all the concepts.
This chart shows the estimated “survival” curves based on all 90 first-round picks between 2013 and 2015, demarcating how long it’s expected to take players to reach 20, 40, and 80 games with a game score of at least 10 (Namita used the 40 and 80 games benchmark in her study as well, which works out just fine since the NHL and NBA both play 82 game regular seasons). Think of it like this — how quickly can these players be expected to start contributing? As should already be apparent, it becomes increasingly difficult and takes longer to accrue more and more great games. Who knew! But this is only where the fun begins. Let’s do another sanity check. Hypothesis: high draft picks should mature quicker than lower draft picks.
Thus far, we’re two-for-two. Higher draft picks remain the best way to try and capture production. There is a large disparity between the survival of draft picks from the top of the first round to draft picks in the bottom of the first round, especially when using the 80 games benchmark. So let’s go back to Donovan Mitchell and Brandon Ingram for a second. They were both picked in the top half of the first round. Which means it’s time for hypothesis No. 2: position impacts development timelines.
Bingo! While this obviously doesn’t magically explain the entirety of the difference between the two players, it does provide a window into observing how certain positions have matured at different rates in recent years. Applying a three-position taxonomy, big men and guards are typically quicker to produce compared to their peers at the wing. This should jive anecdotally as well. In this decade, Andrew Wiggins is the only wing to grab Rookie of the Year honors, but A) it was in one of the weakest drafts in recent memory, and B) he has not progressed beyond his rookie season as hoped, especially on defense. Kawhi Leonard, Otto Porter, Victor Oladipo (among others) are all examples of wings who took some time to gradually translate their versatile potential into consistent on-court results.
Meanwhile, guards have the chance to accumulate numbers faster by sheer virtue of having the most control over the ball, and big men, well… look no further than the arrival of the Unicorn Age of the NBA. But this also leads to a cautionary tale on the danger of overemphasizing early production, especially if it’s empty calories on bad teams. Look no further than two of Philadelphia’s own recent draft picks — Michael Carter-Williams and Jahlil Okafor. Carter-Williams won Rookie of the Year and reached 40 games with a game score of 10+ within his rookie season, however he was never able to evolve beyond “good numbers with high usage on bad team” and was soon traded out of Philadelphia. Big Jah fared even worse. He hit the 40-game mark in his second season in Philadelphia, but was recently traded to the Nets, and unlike MCW is still searching for his 80th game with a 10+ game score, the prospects of accomplishing which get dimmer and dimmer. A lot of players may be able to put up a run of gaudy stats quickly, but sustaining that production for longer stretches is where the wheat really gets separated from the chaff.
Survival analysis goes beyond Kaplan-Meier curves though. We can also perform survival regression, and model the hazard rate based on certain parameters. This gives us the chance to introduce more numeric data such as age at time of draft and player height. Hazard rate is a fairly intuitive concept that flows naturally from survival curves. What is the probability that you’ll reach a milestone at time T, if you haven’t hit it by time T-1? To do this, I can use Cox’s Proportional Hazards model (explained and illustrated very well in this paper by Fox and Weisberg) and observe the impact of five simple and easy to observe variables — position (frontcourt/not), international (yes/no), height, age at draft, and draft slot — against the time it takes to reach 40 games with a game score of 10+. These five variables in the 2013-2015 data set produced a concordance of 0.75 out of 1 in the CoxPH regression, which is generally very solid as far as validation of the survival regression goes.
The multipliers charted above are the resultant estimates of the effects of each variable on the hazard rate. Draft slot was, as expected, the most significant predictor of production, with each additional draft slot leading to a 9 percent drop in hazard rate (which, in practical terms, means less probability of hitting the target milestone). Height was a variable that had an effect in the positive direction, with each additional inch leading to a 13 percent increase in hazard rate. Intuitively, this makes sense; it’s saying that bigger players are more likely to reach the production milestone. This may cause some whiplash then when we see that being a frontcourt player leads to a large dip in hazard rate. What gives? Well, what likely happened is that since I grouped both wings and big men together as frontcourt players, the wings are being penalized, which is being reflected in the overall frontcourt variable.
Likewise, despite the success of players like Giannis Antetokounmpo and Kristaps Porzingis, the recent draft history of international players is still littered with Sergey Karasev’s and Bruno Caboclo’s. We also can’t ignore than many international players (especially those not at the top of the draft) also tend to be “draft-and-stash,” which is a strategy that the Spurs have notably used often at the bottom of the first round. The immediate readiness to contribute is a risk that many teams have to weigh with international players; non-rebuilding teams may not necessarily have the timeline or luxury of depth to wait a couple years for young international players to develop or come over stateside.
Finally, although the age variable was deemed marginally insignificant by P-Value, its relationship can still provide some insight. Each additional year of age showed a 4 percent decrease in hazard rate, meaning that older players were slightly more likely to be more productive quickly. Which makes a lot of sense! We would expect older players to be more developed and younger players to be more raw. While we often prize younger players in the draft, it’s because they will have more room and time to grow into stars, not because we expect younger players to be stars quicker than their older counterparts.
Next: Nylon Notebook -- Kristaps, the clutch Thunder and the surging Timberwolves
Remember, Donovan Mitchell is a 21-year-old rookie playing at the guard spot, and Brandon Ingram was 19 last year playing at the wing. Factors like age and position can make a difference, and it’s all about expectation and perspective. If Mitchell had come out struggling and unable to make any real impact, the conversation would be more about how, at 21, his window for improvement is more limited. In fact, that’s very similar to the preseason dialogue surrounding Kris Dunn. But Dunn too, like Ingram, has shown signs of real improvement this season.
So just keep some of these variables in mind if your favorite rookie is not playing up to your expectations this year. There are a lot of factors that impact player development, and there’s still a whole lot of game left to be played. And if you’re a Jazz fan? All I can say is congratulations.
*Model data sets compiled via data from Basketball Reference