Nylon Questions: How will spacing and shot selection evolve to maximize offensive efficiency?
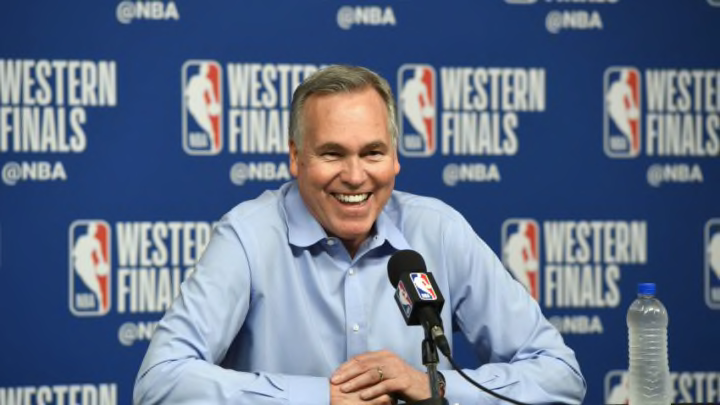
Sports analytics is a constantly evolving field and keeping up can be a challenge, especially with so much work being divided between the public and private spheres. As we head into the 2018-19 season, Nylon Calculus wanted to take stock of where we are and of what’s coming next.
This project is a throwback to work Keith Woolner did for Baseball Prospectus nearly two decades ago, and an update to Kevin Pelton’s basketball-specific version from five years ago. Our staff compiled 10 questions whose answering will likely guide the next few years of public analytic work. Not knowing what has been accomplished in private by NBA teams and consulting firms, we focused on questions that could be worked on in the public sphere, wouldn’t have to be answered with existing datasets (we don’t want to imagine the data we have now is all we’ll ever have) and things that would theoretically have an effect on how teams operate on and off the court.
Hopefully, these questions will help spark, refocus, and recalibrate conversations and lead to collaborative progress here at Nylon and everywhere else sports analytic work is being done.
2. How will spacing and shot selection evolve to maximize offensive efficiency?
Diffusion of innovations, popularized by Everett Rogers, is a theory attempting to explain how ideas and innovations are spread, and, eventually, adopted. The theory finds its roots in agriculture, or, more specifically, rural sociology, which studied the adoption of new farming equipment in the early 20th century. Ideas are adopted in five distinct steps: knowledge, persuasion, decision, implementation, and confirmation. Populations, similarly, are split into five categories: innovators, early adopters, early majority, late majority, and laggards. Innovators and early adopters find the most benefit from the new ideas. For seeing the 3s worth, Mike D’antoni is an innovator. On rest, Tom Thibodeau is laggard. Most, though, fall somewhere in the middle — early or late majority.
It is my firm belief that the earliest adopter of the 3-point shot is the man that truly brought a championship to Miami in 2006, Antoine Walker. Walker was not a superb shooter, just a 32 percent 3-point shooter for his career, but he led the league in 3-point attempts three seasons in a row. Similarly, the Boston Celtics led the league in 3-point attempt rate each of those seasons. Setting the bar for adoption at a three-point attempt rate of .252 — the rate at which the Celtics attempted threes in the 2000-01 season, the same season Antoine Walker averaged a career-high 23 points-per-game — we can visualize the adoption of the three over time, measured as the percent of teams that met or surpassed the benchmark in a given season.
This past season was the first in which all teams reached the benchmark. The adoption was slow going — in part due to the fact that teams that shot a lot of 3s often weren’t all that efficient in the early days — but the revolution hit a clear spike in 2014. The 3-point revolution culminated in the first time a team had a 3-point attempt rate above .5 – the Houston Rockets had a rate of .502 this season.
The past two seasons I’ve wondered, a lot, whether or not there is a tipping point for the 3 — if there are diminishing returns to shooting more 3s, and when that loss would reach a significant enough magnitude to matter. The results are clear, a 3 is a more efficient shot than a mid-range jumper, but as defenses adjust, desired shooting zones become smaller and thus offenses become more predictable. Is it possible the two meet at an equilibrium? Essentially, the question is whether or not a 3-pointer’s quality is a function of its frequency.
Certainly, viewed at the extremes, the quality of the shot will depend on its frequency. A team that has decided to only shoot 3s will struggle to consistently create quality looks from deep. The offense will have become too predictable, and the defense will have no reason to worry about any other zone on the court allowing them to sell out on shooters. Opposite that, a team that shoots only one 3 per-game will find it very easy to locate one high-quality shot.
Of far greater importance, though, is not the extremes, but the middle. Houston’s record-setting rate of shooting 3s was “only” 50%. The question becomes how does efficiency and frequency interact when a team attempts 30 percent of their field goals from 3? 50 percent? 60? The slope between these points may, in fact, be insignificant.
Dean Oliver derived skill curves for individual players in his book Basketball on Paper. A skill curve measures a player’s offensive efficiency at various usage percentages. Deriving a modified version of Dean Oliver’s skill curve for a team’s shot type is the first step to the analysis. Determining this relationship is difficult given the noise associated with jump shooting numbers and insignificant variation in the number of attempts for a team or player. Being able to do so, though, would enable us to analyze the optimal shooting distribution for a team.
Given the ability to derive this function, borrowing from economics, this would be a simple optimization problem. We can use a graph to visualize a simplified version of the model, with only a choice between twos and threes.
Traditionally, the K is for capital(C is reserved for denoting costs) and L is for Labor. For our purposes, 3-point shots will be on the vertical axis, and K stands for Klay. Two-pointers are on the horizontal axis, and L stands for LaMarcus. The curve in the center is an isoquant, and denotes a certain level of output — we will interpret it as denoting a number of points — and the combination of inputs that will result in that output. As we move along the curve to the right the number of 2s taken increases, and as you move back to the left the number of 3s increases. The slope of the isoquant is equal to the function describing the efficiency of the 3-pointers divided by the function describing the efficiency of 2-pointers — the slope is also equal to the derivative of the point function with respect to either shot type, in economics this would be known as the marginal production of the shot. A simple linear set-up for this graph would look something like this, the number, of course, are made up and do not reflect reality:
- The total number of shots available is 100
- Expected points per-shot for threes(PPS3)= 1.2-.0025x
- Where x is the number of 3-pointers attempted
PPS2=1-.0005y - Where y is the number 2-pointers attempted and is equal to 100-x
- The slope of the isoquant is(1.2-.005x)/(1-.001y)
- Total points = (X*PPS3)+(Y*PPS2)
X(1.2-.0025x)+Y(1-.0005y)
The final piece of the graph is the straight line that runs from axis to axis. This is the budget constraint. The slope of the budget constraint is described by the costs of the two inputs. Luckily, the cost of both inputs is the same, taking one more of either shot simply costs a shot, therefore the slope of the budget constraint is 1.
The optimal combination of shots is the point at which the budget constraint and the isoquant barely touch — the point at which they are tangent. This also happens to be the point where the slope of the isoquant and the slope of budget constraint equal each other. Because the slope of the budget constraint is 1, the optimization condition it the point at which the marginal production of a 2 and a 3 are equal to each other.
Given the arbitrarily designed scenario above, the optimal number of 3-pointers and 2-pointers are each 50.. Close observers may have noticed that the solution is equal to simply setting the two lines equal to each other and solving for the intersection point — the graph for which is much easier to understand. That is true. However, I do prefer the graph above for its ability to show point levels, corresponding shot combinations, and the inclusion of a budget constraint. The budget constraint, in particular, may come in handy when accounting for turnovers. If a team pursues shots that are more difficult to create, then the costs may not be equal.
Not all 2-pointers were made equal though. Shots at the rim are often the most efficient shot a team can take, while the mid-range is the least. Expanding the model to account for all shot zones is very hard to visualize, but the math is not exceptionally complex. It may not be necessary for the purpose of this article, though. However, for those interested Brian Skinner solved an analogous model for distributing shots to players in a lineup in his paper The price of anarchy in basketball. The math is very important, but the logic and the conclusion are even more so. The conclusion to the model is that the optimal offense is one in which the marginal production of each shot-type–– at the rim, short mid-range, long mid-range, corner and above-the-break 3 — are equal to each other. The rate at which you take a shot is not just dependent on how efficient it is in general, but how that efficiency responds to an increase in frequency — shots at the rim are the most efficient shot in basketball, but that efficiency also likely decays at a fast rate as defenses pack the paint.
Next. Meet the 2018 NBA 25-under-25. dark
It is important to remember that the relationship between a shot’s efficiency and its frequency is dependent on the player’s skills and the offensive system employed. Because James Harden and Chris Paul are so obscenely gifted at distributing the ball and scoring in isolation, Houston is far more capable at sustaining an offense that focuses heavily on shots at the rim and from 3 than most teams. The Warriors have such an exceptionally talented group at shooting that they seem to consistently make shots from deep regardless of the defense.
Deriving the necessary team skill curves, then determining the optimal shot-type combination will allow teams to decide which players to sign, which offensive systems to employ, and how to train their teams. Beyond that, developing theoretical skill curves based on a team’s roster may help evaluate coaching decisions.