Nylon Calculus: The statistical ramifications of changing teams
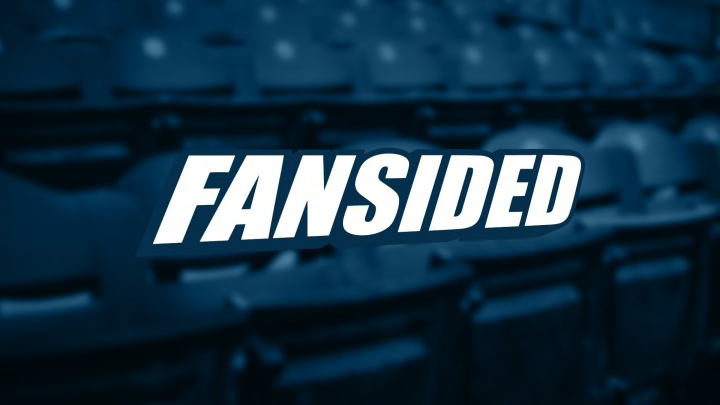
What happens when a “good stats-bad team” player gets traded to a better team? This is a common question facing many a contender at the trade deadline every year. If you make a trade for a productive players, are you getting a guy feasting on big minutes and bigger opportunities in meaningless games, or are you getting a guy who can really provide some production in areas of need?
Using methodology previously discussed, I wanted to look at how changing team strength affects a player’s statistical output. For example, when I looked at how team strength impacts shot selection, I found that players who moved to better teams tended to see an increase in shot quality. But are those gains generalizable? What about for some other statistics?
To examine this, I looked at correlations between changes in individual statistics and the SRS difference the teams. Here were the results:
Many of the correlations are very weak or nonexistent. And for many of these stats, that makes sense. For example, a player’s block percentage isn’t going to get better simply because they moved to a better team. You either have the ability to block shots or you don’t. Playing with better teammates isn’t going to mean you can increase that ability.
However, there are a few statistics with some interesting correlations. First, the correlation for true shooting percentage is weak to borderline nonexistent for players with 200+ minutes but because true shooting percentage includes both effective field goal percentage and free throw rate, we don’t really know what the correlation would be for shooting-only stats. It’s possible that the free throw rate is dragging down the overall correlation for true shooting percentage. Certainly, we know that shot quality improves when moving to a better team and so it would not be surprising to see the shooting percentages follow this same trend. So for now, we’ll move on to the other two interesting correlations: assist percentage and usage rate.
Both of these statistics are more team dependent than the rest of the statistics and so it’s not surprising that they have the strongest correlations with team quality. Both correlations make perfect sense: as a player, your usage is likely to drop if you’re going to a better team since that team will have better players who are already higher usage. The same holds true for assist percentage: if you’re going to a better team, who is presumably already good at assisting shots, your assist percentage is more likely to drop as the team doesn’t “need” your assists as much as your previous team may have.
Additionally, you’re likely to have less opportunities to rack up assists as the team you’re going to may already have players who are getting many assist opportunities. Essentially, as it relates to both assist percentage and usage rate the ball generally needs to be shared more on better teams. And yet, as we saw in previous research, there’s a pretty strong correlation between usage and assist percentages on the first team and on the second team. These points seem to contradict each other. In that previous work, I theorized that within a season, it’s difficult to ask a player to change his role too much and that’s why there was a high correlation. But it could be that in the extreme cases where a player moves to a drastically better or worse team, they do need to change their role a lot, either to carry the team in the case of a bad team or to fit in in the case of the great team. This is another area that’s worth digging into a bit more.
Next, let’s look at some of the results for the all-in-one stats. Theoretically, if the statistic is doing a good job at truly capturing individual value, there should be a low correlation.
The first thing you may notice is that the defensive statistics have a higher correlation with team success than any of the rest. This isn’t surprising: defensive statistics are A LOT worse than offensive ones, never mind the fact that both of the defensive statistics listed above are based on the box score, which has a total of two individual defensive statistics. Additionally, both defensive Box Plus-Minus (BPM) and especially defensive Win Shares have a team adjustment component to it. And this is the reason that both BPM and Win Shares have stronger correlations with team success than PER, which outside of steals and blocks, does not factor in defense.
Speaking of PER, the negative correlation is interesting. This suggests that players’ raise their PER when going to worse teams. Given that PER is so usage dependent, this makes some sense. If a player is going to a worse team, and forced to increase their usage due to bad teammates, their PER is more likely to go up because of the higher load. Also interesting: the correlations with respect to the different minute bins. This may be noise but there’s a higher (negative) correlation for the players in the 200-500 minute bin than in the 500+ minute bin. These players are typically going to be bench level players and it’s possible that by going to a worse team, they may start to get starter level minutes or at the very least, witness a much increased role relative to the non bench players who switch teams. This might explain the larger negative correlation. Or, as I said earlier, it could just be noise.
Finally, when comparing the three all-in-one stats (BPM, Win Shares and PER), Win Shares is the most team dependent and perhaps the worst of the three in terms of capturing true individual value. The main reason for this is that while offensively, Win Shares doesn’t seem to be any worse than it’s counterparts, defensively, there’s a strong correlation between DWS/48 change and team strength change. That’s not necessarily a bad thing but only as long as you’re aware of how team dependent the defensive Win Shares statistic is.
Next: LeBron James is playing an astounding number of minutes
I’ll end with this: of the all-in-one stats that are included here (and there are many superior metrics like Dredge, PT-PM, HBox, etc. that aren’t included here), BPM is the winner here. While PER has a weak correlation with team strength change, it also remains mostly an offensive metric and therefore more likely to be stable for players changing teams midseason. When comparing PER to OBPM, we see that OBPM change has the weaker correlation with team strength change. Overall, BPM change has a pretty weak correlation with team strength change and if the goal is to find a truly individual metric, BPM seems to come closest to achieving this while considering both ends of the court.