Predicting MLB Success from College Players-Off the Radar
By clinthulsey
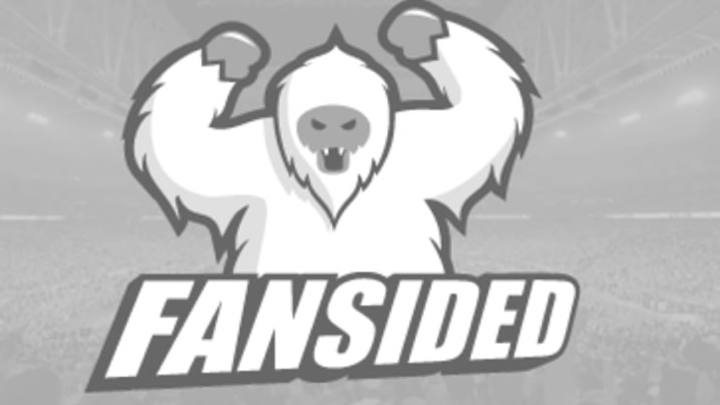
June 21, 2011; Omaha, NE, USA; A general view to the entrance of TD Ameritrade Park prior to the game between the Virginia Cavaliers and the South Carolina Gamecocks. Mandatory Credit: Brace Hemmelgarn-USA TODAY Sports
The book Moneyball is often misunderstood, usually by people that didn’t read it or think it is about on base percentage. One of the key themes in the book (hint: the key to the book was finding players that were not correctly valued by other teams, with David Justice, Chad Bradford, Ricardo Rincon, and Scott Hatteberg being the MLB examples) was the draft, and how the Athletics front office believed that college statistics were an underutilized tool that they could take advantage of in the draft (the book was about the draft as much as it was about the MLB club, but the former doesn’t really show up in the movie). Of course, that was over a decade ago, and the draft, outside of Nick Swisher, was pretty ineffective, but college statistics are used by MLB teams to help evaluate for the draft. In the past, I developed a translation method to predict MLB success using college statistics, but it had a survivor bias in it and the linear method was pretty simplistic, so I don’t really use it. Also, the bats in college have changed, which makes comparing OPS difficult, since offensive numbers are different that they were just a few years ago. So, I mainly use scouting, which I do a lot of at my own site. However, I found myself, when writing about players I have watched in college, peeking at OPS, along with looking at things like steals and homers to see if we can get a glimpse of how the players’ tools are playing in games. I thought it might be wise to make a metric that we can grade college players with, and then test whether or not it is predictive or not. I call it Tool WAA. Here are its components:
I will use the Simple Speed Score that I used when designing my KBO WAR, which is just the stolen base/caught stealing component of the statistic. I will then change the value of over or under average to a percentage, as in if a batter has a 6.5 speed score, change it to 1.5% (since 5 is considered average).
I wanted to add a defensive component, but range factor is the only real number we have for college players, which is fine, except the main NCAA baseball website doesn’t keep it, and individual teams only started really archiving their statistics rather recently (so some schools you can go way back, and some you can’t even really look at 2010. Instead, I just used a positional adjustment. I changed the run value (the traditional one used by Tango and others) to a percentage. As I did for all the numbers, I used The Baseball Cube’s college statistics and information. If they don’t specify which outfield position the player played, we will use -5. If they don’t have any specific position in the infield and are listed as just infield, we will use 0.
The third component measures plate discipline, K%-BB%. MLB Average is usually ~20% for Ks, and 8% for BBs, so we will consider 12% average. You will see that the vast majority of the college players have a better K%-BB%, but they are compared to each other, so it isn’t a big deal if most of them are positive here.
Finally, I used HR %. 2.6% is usually MLB average. While not NCAA average, this seemed like a solid measuring stick, and because we are comparing the players, the number itself isn’t that important, it is what their ranking is in comparisons. You then add all the percentages together. It doesn’t create a run value but should give you an idea of how much better (or worse) the players are based on percentage.
Just for fun, Mike Trout’s Tool WAA from 2012 in the Majors (used the regular speed score instead of simple speed score) was 8.89, while Miguel Cabrera’s was 11.21. To test the tool and see if it had any predictive validity, I looked at the Big 12 (A major NCAA conference that gives us over 90 players of a sample size, and enough time that we pretty much know whether or not the players are going to succeed or not) from 2006 (every hitter with over 100 At-bats) and calculated their Tool WAAs below along with their highest level played in:
Name | Speed Score % | Positional Adj. % | K %- BB % | HR % | Tool WAA | Highest Level | Tool WAA -PA | Rank | OPS | Draft |
Drew Stubbs | 1.87 | 2.5 | 4.1 | 2.55 | 11.02 | MLB | 8.52 | 2 | 1019 | 1 |
Corey Brown | 1.16 | 2.5 | 5.06 | 3.42 | 12.14 | MLB | 9.64 | 3 | 1106 | 1 |
Jackson Williams | -1.57 | 12.5 | 10.2 | -1.11 | 19.84 | AAA | 7.34 | 57 | 801 | 1 |
Jordy Mercer | 1.29 | 7.5 | 1.5 | 0.4 | 10.69 | MLB | 3.19 | 726 | 3 | |
Roger Kieschnick | -0.06 | -7.5 | 4.83 | 1.2 | -1.53 | AAA | 5.97 | 22 | 966 | 3 |
Kyle Russell | -1.24 | -7.5 | -11.3 | 3.5 | -16.54 | AAA | -9.04 | 32 | 0.911 | 3 |
Shelby Ford | -2.47 | 2.5 | 5.9 | 3.03 | 8.96 | AAA | 6.46 | 28 | 979 | 3 |
Tyler Mach | -1.89 | 2.5 | 8 | 3.8 | 12.41 | A- | 9.91 | 5 | 1044 | 4 |
Bradley Suttle | -3 | 2.5 | 9.35 | -0.8 | 8.05 | AA | 5.55 | 47 | 795 | 4 |
Tyler Reves | -5.5 | 12.5 | 4.71 | 2.66 | 14.37 | A+ | 1.87 | 37 | 869 | 4 |
Joe Dunigan | -0.27 | -7.5 | -9.19 | 0.79 | -16.17 | AA | -8.67 | 825 | 5 | |
Jeff Christy | -0.78 | 12.5 | 3.04 | 1.38 | 16.11 | AAA | 3.61 | 813 | 6 | |
Ryan Rohlinger | -2 | 2.5 | 15.52 | 2.48 | 18.5 | MLB | 16 | 1 | 1067 | 6 |
Jordan Danks | 1.29 | 2.5 | 9.42 | -0.88 | 12.33 | MLB | 9.83 | 61 | 946 | 7 |
Ty Wright | -1 | -7.5 | 5.42 | 0.03 | -3.05 | AAA | 4.45 | 87 | 812 | 7 |
Luke Gorsett | -2 | -5 | 6.69 | 4.65 | 4.33 | AA | 9.33 | 13 | 1071 | 7 |
Beamer Weems | -2.23 | 7.5 | 3.81 | 0.85 | 9.93 | AAA | 2.43 | 26 | 874 | 8 |
Jared Goedert | 0.85 | -5 | 19.61 | 3.92 | 19.38 | AAA | 24.38 | 11 | 1074 | 9 |
Evan Frey | 0.33 | 2.5 | 9.89 | -2.6 | 10.12 | AAA | 7.62 | 40 | 847 | 10 |
Seth Fortenberry | 1.29 | -5 | 0.99 | 1.36 | -1.36 | AAA | 3.64 | 10 | 993 | 11 |
Jacob Priday | 0.33 | -5 | -2.59 | 2.12 | -5.14 | A | -0.14 | 36 | 848 | 11 |
Kyle Colligan | -0.27 | -7.5 | -7.16 | -0.1 | -15.03 | A+ | -7.53 | 792 | 12 | |
Craig Stinson | -3 | 12.5 | 9.74 | -1.1 | 18.14 | A- | 5.64 | 45 | 681 | 12 |
Jake Opitz | -3 | 2.5 | 8.1 | -1.62 | 5.98 | AAA | 3.48 | 84 | 780 | 12 |
Blake Stouffer | -0.096 | 2.5 | 8.55 | -0.88 | 10.07 | A | 7.57 | 39 | 751 | 13 |
Gus Milner | 0.85 | -5 | 2.19 | 0.042 | -1.92 | AAA | 3.08 | 7 | 915 | 14 |
Carson Kainer | -1.34 | -5 | 5.51 | -0.87 | -1.7 | AA | 3.3 | 20 | 975 | 14 |
Matt Smith | -5.5 | 12.5 | 13.64 | 1.23 | 21.87 | A+ | 9.37 | 17 | 1059 | 14 |
Kody Kaiser | 0.6 | -5 | 1.38 | 0.26 | -2.75 | AA | -2.25 | 8 | 894 | 15 |
Hunter Mense | 0.33 | -5 | 3.55 | -1.66 | -2.78 | AAA | 2.22 | 54 | 717 | 17 |
Chance Wheeless | -3.59 | -12.5 | 6.98 | -1.69 | -10.8 | A | 1.7 | 82 | 716 | 17 |
Deik Scram | -0.06 | -7.5 | 2.5 | 0.97 | -4.09 | AAA | 3.41 | 15 | 1077 | 18 |
Ritchie Price | -2.23 | 0 | 4.41 | -1.91 | 0.27 | A- | 0.27 | 18 | 704 | 18 |
Andrew Brown | -6.3 | -7.5 | -0.06 | 3.78 | -10.08 | MLB | -2.58 | 59 | 897 | 18 |
Nick Peoples | 2.17 | 2.5 | 4.5 | -1.28 | 7.89 | A | 5.39 | 16 | 791 | 19 |
Brandon Buckman | -2.09 | -12.5 | 15.04 | 3.4 | 3.85 | AA | 16.35 | 24 | 980 | 19 |
Ryan Wehrle | 3.19 | 0 | 8.9 | 0.94 | 13.03 | A- | 13.03 | 12 | 1026 | 20 |
Kevin Russo | 2.65 | 2.5 | 13.29 | -1.74 | 16.7 | MLB | 14.2 | 29 | 736 | 20 |
Zach Dillon | -1 | 12.5 | 27.53 | -0.66 | 38.37 | AA | 25.87 | 14 | 996 | 20 |
Matt Clarkson | -4.43 | 12.5 | -0.14 | -0.46 | 7.47 | A- | -5.03 | 755 | 20 | |
Joey Callender | 0.55 | 2.5 | 13.2 | -2.2 | 14.05 | A+ | 11.55 | 34 | 764 | 21 |
Aaron Reza | -1.42 | 7.5 | 1.86 | -2.14 | 5.8 | A+ | -1.7 | 27 | 826 | 21 |
Byron Wiley | -1.24 | -5 | 11.31 | 0.85 | 5.92 | A+ | 0.92 | 64 | 907 | 22 |
Brock Bond | -1.33 | 2.5 | 9.01 | -1.6 | 8.58 | AAA | 6.08 | 41 | 858 | 24 |
Keanon Smith | 0.85 | -5 | 2.91 | 0.13 | -1.11 | A | 3.89 | 31 | 910 | 25 |
Rebel Ridling | -4.43 | -12.5 | 4.6 | -0.75 | -13.08 | AA | -0.58 | 868 | 25 | |
Cristen Tapia | -4.43 | -12.5 | 5.9 | -0.31 | -11.34 | NCAA | 1.16 | 76 | 781 | 28 |
Parker Dalton | 1.4 | 0 | -3.03 | -1.95 | -3.58 | A | -3.58 | 69 | 617 | 29 |
Kyle Martin | -3.91 | 0 | 5.02 | 2.05 | 3.16 | A | 3.16 | 885 | 29 | |
Brian Capps | -2.57 | 0 | 6.04 | -1.68 | 1.79 | A- | 1.79 | 66 | 875 | 30 |
Jared Schweitzer | -3 | 2.5 | 12.41 | 1.96 | 13.87 | A | 11.37 | 6 | 1018 | 30 |
Preston Clark | -4.1 | 12.5 | 4.6 | -0.29 | 12.71 | NCAA | 0.21 | 42 | 749 | 33 |
Ryan Lollis | -1.89 | 2.5 | 6.06 | -1.61 | 5.06 | AAA | 2.56 | 820 | 37 | |
Chuckie Caufield | 0.33 | -7.5 | 6.46 | 0.72 | 0.015 | AAA | 7.515 | 4 | 958 | 39 |
Eli Rumler | -2.09 | 7.5 | 1.4 | -2.6 | 4.21 | AA | -3.29 | 65 | 678 | 39 |
Kevin Smith | -3.91 | 2.5 | 5.08 | 0.86 | 4.53 | AA | 2.03 | 9 | 899 | 39 |
Erik Morrison | 1.29 | 2.5 | -1.06 | 3.11 | 5.84 | AA | 3.34 | 21 | 867 | 46 |
Brock Simpson | -1.57 | -5 | 9.07 | -0.16 | 2.34 | A- | 2.66 | 63 | 823 | 46 |
Willie Rueda | 3 | 0 | 19.39 | -2.03 | 20.36 | NCAA | 20.36 | 35 | 786 | |
Brandon Farr | 1.55 | 12.5 | 21.04 | -2 | 33.09 | NCAA | 20.59 | 33 | 821 | |
Joe Roundy | 1.44 | -5 | 4.42 | 1.44 | 2.3 | Rk | 7.3 | 19 | 1042 | |
Nick Jaros | 1.44 | -5 | 4.63 | -0.49 | 0.58 | Ind | 5.58 | 88 | 890 | |
John Allman | 1.29 | 12.5 | 2.3 | -0.07 | 16.02 | A+ | 3.52 | 23 | 906 | |
Barrett Rice | 1.29 | 0 | 0.42 | -1.02 | 0.69 | Ind | 0.069 | 43 | 938 | |
Russell Daley | 1.29 | 0 | 5.82 | -1.5 | 5.63 | AA | 5.63 | 58 | 700 | |
Aaron Ivey | 1.2 | -5 | 14.88 | -1.64 | 9.36 | NCAA | 14.36 | 56 | 725 | |
Bryce Nimmo | 1.07 | -5 | 12.49 | -1.14 | 7.42 | NCAA | 12.42 | 46 | 729 | |
Chase Gerdes | 0.85 | -5 | 4.31 | -1.57 | -1.41 | Ind | 3.59 | 44 | 811 | |
Trevor Helms | 0.85 | 0 | 8.53 | -1.44 | 7.94 | NCAA | 7.94 | 74 | 709 | |
Matt Sodolak | -0.06 | 12.5 | 3.18 | -2.6 | 13.02 | NCAA | 0.52 | 83 | 651 | |
Gary Arndt | -0.14 | 0 | 6.04 | -0.31 | 5.59 | Ind | 5.59 | 80 | 718 | |
Jake Mort | -0.37 | 0 | 3.91 | -2.6 | 0.94 | NCAA | 0.94 | 77 | 691 | |
Austin Boggs | -0.5 | 2.5 | 1.9 | -2.6 | 1.3 | NCAA | -1.2 | 51 | 690 | |
Jose Salazar | -0.62 | 0 | 13.65 | 2.05 | 15.08 | AAA | 15.08 | 50 | 740 | |
Zane Taylor | -0.86 | 0 | 12.41 | -1.78 | 9.77 | NCAA | 9.77 | 30 | 799 | |
Ben Booker | -1 | -5 | -3.24 | -1.38 | -10.62 | NCAA | 5.62 | |||
Blair Wilkins | -1.89 | 0 | 15.01 | -3.04 | 10.08 | NCAA | 10.08 | 78 | 894 | |
John Infante | -2 | -5 | 7.42 | -1.84 | -1.42 | NCAA | 3.58 | 644 | ||
Preston Land | -2.14 | 0 | -5.61 | 3.06 | -4.7 | NCAA | -4.7 | 38 | 994 | |
John McKee | -2.14 | 2.5 | -4 | 0.4 | -3.25 | NCAA | -0.75 | 62 | 842 | |
J.C. Field | -2.14 | 12.5 | 5.17 | -0.12 | 15.41 | Ind | 2.91 | 71 | 712 | |
Buck Afenir | -2.14 | 12.5 | -5.59 | 1.1 | 5.87 | A- | -6.63 | 738 | ||
Jake Vazquez | -2.23 | 12.5 | 2.25 | 1.47 | 13.99 | NCAA | 1.49 | 86 | 783 | |
Tyler Link | -2.33 | 0 | 6.5 | -1.68 | 2.49 | NCAA | 2.49 | 751 | ||
Kevin Sevigny | -2.41 | -5 | 2.87 | -0.77 | -5.31 | NCAA | -0.31 | 60 | 771 | |
Matt Baty | -3 | -5 | 13 | -1.6 | 3.4 | NCAA | 8.4 | 49 | 816 | |
Chais Fuller | -3 | 2.5 | 5.6 | -2.6 | 2.5 | A+ | 0 | 55 | 0.611 | |
Freddy Rodriguez | -3 | 0 | 10.45 | -2.08 | 5.37 | NCAA | 5.37 | 67 | 720 | |
Ryan Hill | -3 | -5 | 11.07 | -1.67 | 1.4 | NCAA | 6.4 | 91 | 702 | |
Derek Chambers | -4.11 | -12.5 | 8.86 | 0.09 | -7.66 | NCAA | 4.84 | 48 | 818 | |
Tim Jackson | -4.43 | 2.5 | 1.17 | -2.6 | -3.36 | NCAA | -0.86 | 70 | 645 | |
Andy Gerch | -6.33 | -5 | 2.84 | 0.45 | -8.04 | NCAA | -3.04 | 90 | 842 |
25 Players didn’t play in a higher level than the NCAA, they had an average Tool WAA of 3.8
Independent (5): 4.17
A- (8): 7.66 (the one rookie ball player had a 2.3 Tool WAA)
A (8):1.8
A+ (8): 7.09
AA (12): 3.43
AAA (18): 4.88
7 Players made the Majors, they had an average Tool WAA of 10.19
So not a lot of correlation in the middle, but the MLB players were much better than the rest. The NCAA and Independents were worse than most of them, but the A-ball players strangely turned out to be the worst. When you sort by Tool WAA, we don’t see much correlation, as out of the top 20, only 2 players turned out to be MLBers (10 % versus the 7.6%, so better than picking players at random, but not great correlation). In fact, one of the worst 20 players made the Majors (Andrew Brown). Looking at just speed score seemed to be a better predictor, as 5 of the top 20 made the Majors, with Andrew Brown being the 2nd worst and still making the Majors. K-BB % wasn’t a good predictor, with just 2 of the top 20 making the Majors, and Andrew Brown made it despite being in the worst 20. HR % had 4 MLBers in the top 20, with Kevin Russo making the Majors despite being in the bottom 20.
As I was inputting the data, it seemed that the positional values were being weighed too heavily, especially with catchers. 15 players were catchers, none of them made the Majors, and just 2 made AAA. Just to test this out, I took the positional adjustment out and created a 2nd Tool WAA (Tool WAA – PA). This seems to help, as 5 of the MLB players were in the top 21. However, there were still 6 players that didn’t play past college in the top 21, which is a higher percentage per player than in total. There were also just 2 AAA players. So overall, we are seeing that the MLB players tended to have good Tool WAAs in college, but it doesn’t seem to be that predictive. I wanted to introduce some control groups, and I thought of 3, all easily found on Baseball Cube. The first was the draft. Was Tool WAA a better predictor for which players made the Majors than the draft? 2 of the 3 first round picks made the Majors, with the other maxing out at AAA. 5 of the 7 MLB players were drafted in the first 7 rounds (the first 16 players). This is better correlation than the Tool WAA, and the adjusted Tool WAA.
The 2nd was OPS for that season. I talked about how I used to use OPS above, but didn’t really like the linear approach and change of bats made it harder to judge current day players. Did Tool WAA fare better than this? 11 players had an OPS over 1000, 3 of them were the MLBers, with 2 AAA guys mixed in (perhaps most importantly, all of them would play in affiliated baseball). The top 20 as a whole (946 OPS or better) had 4 MLBers. This is worse than speed score, the draft, and the adjusted Tool WAR.
The 3rd was the Baseball Cube hitter rankings, as it ranks the full time hitters based on a formula. The rankings predicted 3 MLB players perfectly, as the top 3 in the rankings all made the Majors. However, you have to go to 29 to see the next MLB player. This seems weaker than OPS as a whole.
I want to go back to the draft and see if we can separate what caused the 11 players that have failed to make the Majors in the top 7 rounds versus the 5 that did. This may give us an idea of which college numbers are more predictive.
Speed Score % of the 5 MLB Players: .722
Speed Score % of the 11 nonMLB players: -1.8
Tool WAA (-PA) of the 5 MLB Players: 9.44
Tool WAA (-PA) of the 11 nonMLB Players: 3.34
OPS of the 5 MLB Players: 973
OPS of the 11 nonMLB Players: 899
Rank of 5 MLB Players: 32 (for non ranked players, I gave them the rank of 93)
Rank of the 5 nonMLB Players: 47
All of them were predictive, but it seemed that Tool WAA was the best predictor.
So here I will at the 2012 draft, and look at all the college position players taken in the first 15 rounds of the draft, and list their adjusted (no positional adjustment) college career tool WAA.
Speed Score % | HR % | K%-BB% | Tool WAA | Team | Round | |
Alex Yarbrough | -0.5 | -0.61 | 6.03 | 4.92 | Angels | 4 |
Nolan Fontana | 1.04 | -0.22 | 21.37 | 22.19 | Astros | 2 |
Andrew Aplin | -1.13 | -1.25 | 18.07 | 15.69 | Astros | 5 |
Max Muncy | -0.27 | 1.19 | 10.03 | 10.95 | Athletics | 5 |
Blake Brown | 0.85 | 0.42 | -2.84 | -1.57 | Braves | 5 |
Victor Roache | -1.7 | 7.45 | 9.49 | 15.24 | Brewers | 1 |
Mitch Haniger | -3.3 | 1.89 | 10.5 | 9.09 | Brewers | 1 |
James Ramsey | 1.69 | 1.83 | 12.13 | 15.65 | Cardinals | 1 |
Stephen Piscotty | -0.27 | -0.86 | 11.13 | 10 | Cardinals | 1 |
Patrick Wisdom | -1 | 2.36 | 4.14 | 5.5 | Cardinals | 1 |
Alex Mejia | -2.55 | -2.04 | 6.36 | 1.77 | Cardinals | 4 |
Ronnie Freeman | -1 | 1.2 | 8.35 | 8.55 | Dbacks | 5 |
Mac Williamson | 1.07 | 3.65 | -3.63 | 1.09 | Giants | 3 |
Tyler Naquin | -3 | -1.58 | 6.18 | 1.6 | Indians | 1 |
Chris Taylor | 1.29 | 1.39 | 9.24 | 11.92 | Mariners | 5 |
Patrick Kivlehan | 2.43 | 4.81 | 2.47 | 9.71 | Mariners | 4 |
Mike Zunino | 1.59 | 4.26 | 2.51 | 8.36 | Mariners | 1 |
Austin Nola | -1.33 | -0.84 | 10.37 | 8.2 | Marlins | 5 |
Kevin Plawecki | -3.43 | 0.06 | 15.6 | 12.2 | Mets | 1 |
Matt Reynolds | 1.58 | -0.4 | 9.6 | 10.78 | Mets | 2 |
Tony Renda | 2.77 | -1.03 | 9.15 | 10.89 | Nationals | 2 |
Spencer Kieboom | -4.43 | -1.67 | 14.32 | 8.22 | Nationals | 5 |
Brandon Miller | -2.09 | 5.81 | 2.19 | 5.91 | Nationals | 4 |
Christian Walker | -2.09 | 1.47 | 16.48 | 15.86 | Orioles | 4 |
Jeremy Baltz | 2.11 | 2.91 | 10.55 | 15.57 | Padres | 2 |
Travis Jankowski | 3.91 | -1.34 | 9.3 | 11.87 | Padres | 1 |
Fernando Perez | 0.33 | -0.83 | 8.47 | 7.97 | Padres | 3 |
Dane Phillips | -3 | -1 | 0.81 | -3.19 | Padres | 2 |
Chris Serritella | 0.85 | 2.27 | 2.75 | 5.87 | Phillies | 4 |
Barrett Barnes | 2.82 | 2.58 | 7.13 | 12.53 | Pirates | 1 |
Brandon Thomas | 2 | -1 | 7 | 8 | Pirates | 4 |
Pat Cantwell | 0.62 | -1.66 | 10.66 | 9.62 | Rangers | 3 |
Preston Beck | -3.34 | 0.54 | 12.3 | 9.5 | Rangers | 5 |
Richie Shaffer | 2 | 2.3 | 9.23 | 13.53 | Rays | 1 |
Andrew Toles | 1.93 | -0.98 | 11.73 | 12.68 | Rays | 3 |
Deven Marrero | 1.47 | -0.5 | 7.45 | 8.42 | Red Sox | 1 |
Jeffrey Gelalich | 2.32 | 0.36 | 1.72 | 4.4 | Reds | 1 |
Tom Murphy | -0.27 | 2.4 | 5.7 | 7.83 | Rockies | 3 |
Kenny Diekroeger | -4 | -1.23 | -0.63 | -5.86 | Royals | 4 |
Adam Walker | 4.92 | 3.77 | -2.9 | 5.79 | Twins | 3 |
Joey Demichle | 0.1 | 1.15 | 5.75 | 7 | White Sox | 3 |
Peter O’Brien | -4.58 | 4.1 | 2.22 | 1.74 | Yankees | 2 |
The problem is that it doesn’t adjust for competition and conference. Which stat was the most predictive for draft position.
Which stats were more predictive for draft position?
The top 10 Speed Score players were drafted in the 2.2 round on average. The worst 10 were drafted in the 2.9 round on average.
The top 10 HR % players were drafted in the 2.4 round on average. The worst 10 were drafted in the 3.1 round on average.
The top 10 K-BB% players were drafted in the 3 round on average. The worst 10 were drafted in the 2.9 round on average.
The top 10 Tool WAA players were drafted in the 2.1 round on average. The worst 10 were drafted in the 2.7 round on average.
It is tough to see a great correlation there, but top Tool WAA players were the most likely to be drafted the highest, though the worst Tool WAA players were drafted higher than the worst of any of the other metrics. Players that did not hit homers were the least likely to be drafted high. K-BB % clearly had the worst correlation, which makes sense since it didn’t predict MLBers in the sample we looked at.
The Padres and Cardinals both had 4 college players in the top 5 rounds, and the Padres had a 5th that went to a community college (and thus didn’t have statistics on The Baseball Cube). The only one the Braves picked had a negative Tool WAA, as was the Royals. The Astros’ had the highest average Tool WAA, with both of their picks with extremely high numbers, but the Rays weren’t that far behind, with two players that had very high Tool WAAs. The Athletics only pick had a 10.95 Tool WAA, and the 3 picks the Mariners had an average of 10 Tool WAA, and the Orioles only pick had a Tool WAA of nearly 16.
So it seems we have found a group of statistics that may help us predict college players success in professional ball a little better. As we get closer to the draft, we will talk more about Tool WAA, and look at current college players.