Fantasy Baseball Projections: How We Developed Our 2014 Numbers
By Tanner Bell
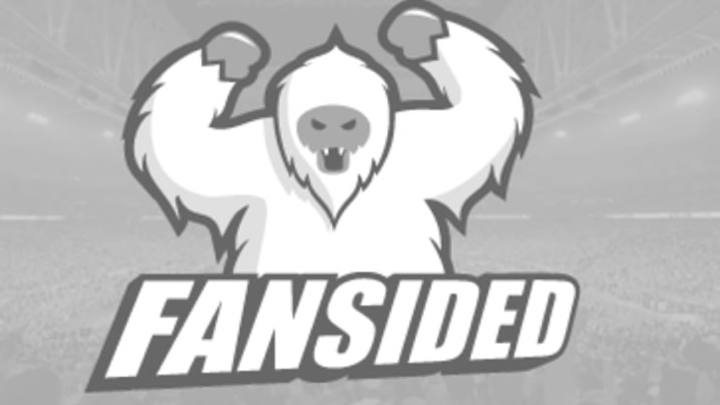
Tim Heitman-USA TODAY Sports
Let me step up onto my soapbox for a moment.
Before you begin to use a set of fantasy baseball projections, you must understand how they were developed. It doesn’t matter the expert’s name, the prestige of the website, or the bells and whistles in the computer program that kicks out the results, you should understand the principles used to formulate the numbers. Don’t just pin the hopes of this six-month-long baseball season on a set of projections you don’t thoroughly trust. Are the projections “best-case scenario”? Do they weigh the possibility of all outcomes? To what level were advanced metrics used in determining the projections?
Blindly trusting a set of projections could be likened to marrying a foreign bride you picked out of a magazine based solely on appearance (Does that still happen? Kind of). So remember, when choosing a bride or a set of fantasy baseball projections, it’s (at least partially) what’s on the inside that counts.
There are likely thousands of fantasy baseball sites out there. So why should you trust the projections at this site?
Judge for yourself. We’re an open book here at Fantasy Baseball Crackerjacks. What follows is a detailed look at the methodology used to develop the 2014 projections you’ll see on the site.
The Big Picture
At a high level, there are three main inputs used in our projections:
- Analyzing Component Stats
- Regression
- The Human Touch
Let’s take closer look at each.
1. Analyzing Component Stats
Baseball is a series of large, difficult to predict events that can be broken down into a series of smaller, easier to predict occurrences. Take a home run for example. It is difficult to determine the exact number of home runs a player will hit. But we can reasonably estimate a player’s batted ball profile (including the percentage of balls that will fly balls, ground balls, and line drives). We can also use a player’s history to determine the percentage of fly balls that become home runs. Finally, we can use our knowledge of the player’s team, lineup competition, and injury history to predict the number of times they will come to bat during the season. Click here to read more about this concept of breaking events into smaller, more predictable pieces.
If you know how many times a player will come to the plate, you can estimate the number of those plate appearances that will result in a fly ball, and have an understanding of how likely each fly ball is to become a home run, then you have yourself a home run projection.
These same principles were used to project batting average and stolen bases for hitters, and to project strikeouts, wins, ERA, and WHIP for pitchers.
How did you project runs and RBI you ask?
2. Regression
Let me give an example to illustrate the concept of regression. A given baseball player, say Mike Trout, has a specific skill level for hitting home runs. Let’s simplify things and say Trout has the skill level to hit 30 home runs in a 162 game period (we can’t “know” this fact, but let’s assume we do for this example). So when Trout came up to the major leagues in 2012 and hit 30 HR in only 139 games, he surpassed this skill level. The principle of regression would suggest that the next season, knowing that Trout’s true skill level is to hit 30 HR in 162 games, Trout will still be expected to hit about 30 HR.
Trout went on to hit 27 HR in 157 games in 2013. Knowing this and his true talent of being able to hit 30 HR in a season, we would project Trout to hit more than 27 HR in 2014.
Stepping out of this simple example, we don’t know what any player’s skill level really is. All we can do is venture a guess based upon the player’s past performance. What we do know is the statistical averages of every major league baseball player. And that it takes a lot of at bats before we can determine a reliable “average” for a given player. So when Trout comes up to the major leagues and hits 30 HR in his first year, how much does that one season tell us about his skill level? If he hits 27 the next year, what does that tell us? How many at bats or home runs do we need to see before we can be sure he’s better than the average replacement level player?
Enter Tom Tango and his monkey Marcel. Last year Clave Jones wrote one of the best explanations of the Marcel projection system I’ve seen. If you’re not already familiar with how Marcel works, open that link in another browser tab and read it after this. That’s an order!
We used the Marcel approach to projecting Runs and RBI because there is no proven accurate means of projecting those two stats. There are no component stats that break RBI down into more digestible parts. Runs and RBI are so dependent upon a hitters surrounding lineup and spot in the batting order. In our minds, the best way to estimate Runs and RBI was to take a pure regression approach to the last three years of actual results. We took each player’s raw RBI and R totals and converted them into per plate appearance numbers (Miguel Cabrera had a ridiculous 0.210 RBI/PA last year). These per PA figures were then run through Marcel which calculates a weighted average of the last three years and then depending on how much MLB experience the player has, regresses that weighted average toward the MLB average.
This method makes us a lot more comfortable than simply trying to guess how many runs Hunter Pence is going to score this season. Over time Marcel has proven to be a very accurate projection system, and we have no reason to believe we can more accurately project stats like R and RBI.
3. The Human Touch
I skipped over a very important part in the “Analyzing Component Stats” segment. While performing those calculations, we look at the last three years of MLB data… And we look at each player one at a time. It’s a long and arduous process. But looking at each player individually allows you to spot trends and oddities that simply averaging the last three years of data does not allow.
The human touch enters greatly into our projection of playing time. A player’s playing time in recent seasons is a big factor in this. If a pitcher missed significant time in recent years, they’re probably not projected for 200 IP. It might be more in the 160 IP range. If a hitter missed significant time, like a Troy Tulowitzki or Carlos Gonzalez, they won’t be projected for 600 plate appearances. And playing time matters. When you take 50-100 PAs from a player’s projection, it makes an 8-16% difference in all their statistics. But the truth is that this is an important adjustment that needs to be made when projecting and ranking players. It would be a mistake to draft Troy Tulowitzki as if he is going to come to the plate 600+ times.
Manual human adjustment does come into play for players that will likely see different spots in the lineup this season. Take Austin Jackson, for example. A straight Marcel regression of his past three years gives him a very strong projection of 0.152 Runs per PA. But he’s batted lead off in front of Miguel Cabrera and Prince Fielder for much of that time. With the addition of Ian Kinsler, who is slotted in as the leadoff hitter, Jackson is currently projected to hit 5th. So instead of hitting in front of two MVP candidates, he’ll be followed by Andy Dirks, Alex Avila, and Nick Castellanos. It wouldn’t be right to leave him projected as an elite run scorer.
You might have also noticed that I have not mentioned Saves yet. And that’s because there is no way to do it! I’m not proud of the method used to project Saves. It’s 100% human touch, “This guy is a reliable closer that’s firmly entrenched in the job on a good team, I’ll give him 35”. Or, “This guy is a strong reliever but he doesn’t have a proven track record and there are two other solid candidates to close. There’s a decent probability he could lose the job at some point, I’ll put him down for 20”. It’s not pretty. But you deserve to know where it came from.
There You Have It.
This process is truly an amalgamation of several different projection approaches, but we feel it’s a strong one with merit. If you’re interested in following this process to make your own projections, you can. It’s easier than you might think, and the process of developing your own projections will help take your fantasy game to the next level.
Much of the model is derived from Mike Podhorzer’s book, “Projecting X: How to Forecast Baseball Player Performance”. The book is an excellent step-by-step guide to the process, provides links to research articles on the various component stats, and even guides you through building an Excel file to calculate everything. I have partnered with Mike to develop the “Smart Fantasy Baseball Projecting X Bundle”, which includes “Projecting X”, an Excel template that allows you to work much more efficiently through the process of making the projections and automatically calculates the R/PA and RBI/PA Marcel regression for each player, and a short e-book guide on how to use the spreadsheet. You can see a couple of short videos demonstrating the bundle here. Or you read more closely about it here. The bundle is available for $14.99.
In addition to the great read by Clave linked above, you can find more information about Marcel here at Tom Tango’s website.