Stat Corner: xG – What is expected goals and why does it matter?
By Dan Voicescu
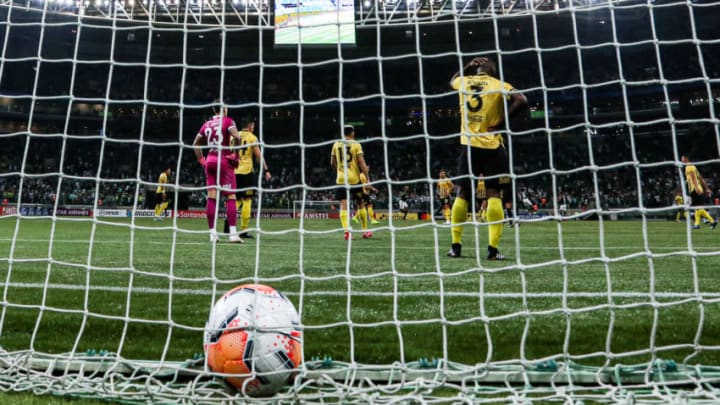
Expected goals, or xG, is one of the most common advanced statistics in soccer. We take a look at what it is and how it can help your understanding of the game.
With the ongoing shift in focus towards a smarter, more analytical understanding of soccer, we are taking advantage of the break in action to describe some of the metrics used by the soccer analytical community. First up is xG (expected goals) — what is it and how does it help your understanding of the game?
What is xG?
The most simplistic plain-English definition of xG is the chance any given shot had in becoming a goal, hence the name expected goals. To take it a step further, xG is the percentage value assigned to an attempt at goal adjusted for situational factors, and it ranges from 0 (unlikely to be a goal) to .99 (absolutely should be a goal).
As example, let’s use an oversimplified situation — a tap in to an empty net from 2 yards out without any pressure has an xG value around .95. Conversely, a shot from outside the 18-yard box with a few defenders (and a goalkeeper) in the path of the shot has an xG somewhere around .01 and .05 depending on the exact positioning of the players involved in that attempt on goal.
xG can be ascribed to an individual player or a whole team. By summing up to the total xG of each individual attempt on goal we get to the Team xG — the numerical value that indicates the total number of goals that a given team was expected to score.
Assuming the two examples above make up the entirety of Team A’s attempts on goal for a given match we can expect their xG to be right around 1. An oversimplified way to interpret that number is to consider it “fair” if Team A actually do end up scoring 1 goal, whether as part of a win, loss or tie. If they fail to score at all, we can safely conclude that someone royally bungled up a goal on the platter and the result may be somewhat unjust. Conversely, if Team A ends up scoring 2 goals, well, good for them on this occasion, but they were “lucky” in a statistical sense and their performance could be difficult to sustain or replicate in the future.
How is xG determined?
Assuming the (relatively) simple reasoning above makes sense, the next question would be how exactly is xG determined (and how can I, Joe Soccerfan poke holes through the logic?)
The short answer is that there are proprietary video tools that analyze every shot towards goal for the past few seasons and thus assign an xG value to each goal-scoring attempt. Based on heaps of data a model is created that creates an xG “score” for each shot. This score is based on the analysis of hundreds or thousands of identical or very similar situations and evaluating the outcomes. Combine together the entirety of the past few seasons worth of games across many leagues into one library and you will be able to extrapolate for any given opportunity.
To illustrate let’s use a simple example: the penalty kick. Coming up with an xG for a penalty kick is (for the most part) as simple as dividing the number of goals scored by the total number of penalty kicks taken – this number is somewhere between .78 (World Cup) and .80 (Premier League) There are only so many factors to control for while assessing the xG for a penalty kick. It is a straight forward game situation, with a determined distance from goal and no other obstacles in front of the keeper.
Obviously, assessing expected goals for all the other dynamic, non-penalty game-play situations is a much more complicated matter as it involves controlling for a number of factors. That is the easy part. The more complicated parts that rely on proprietary models and a bit of “art” combined with the science involve adjustments made for: location of the shot, body part ( a header from 10 yards has a lower xG than a shot from the same place), obstacles or opposition in the way of the shot and the quality of the pass (or potential assist).
The latest and greatest xG models also adjust for the quality of the player as well. Lionel Messi alone on a breakaway will have a higher xG value than a second division center-back in the same situation who is much more likely to shank his shot.
Suffice it to say that there are many factors such as those that go into calculating expected goals, what’s important to realize is that the latest models use more and more variables to get to the most accurate xG. While there is some slight variation between the big players on the market (Opta, Sportec, Statsbomb, Understat, etc) all of the xG models in market are considered very reliable.
Here’s a nice layout of xG and xGA (expected goals against) for each team in the EPL.
The one you will end up seeing is most likely the one that the television channel subcontracts for coverage of their games, but for fans looking to dig into the numbers at home, Understat has free data available online for multiple leagues and Statsbomb xG is available through fbref.com
How does xG improve my enjoyment of the game?
As fans and consumers of the beautiful game we try to contextualize what we see and come up with a somewhat unique narrative for each game. We look at the score, the perceived value of the opponent, the highlights of the game, and our own eye-test to come up with a satisfying conclusion in a “canned” format – “they played great”, “really great game” or “we were robbed by VAR/referees”, “wasn’t our day”, “so many missed chances”
To support all of the above there is a first level of “traditional” stats such as possession pecentage, shots on target, corners etc. These are the most common metrics and they do help somewhat but not nearly as much as xG. One notorious example in the soccer stat-head community is Germany’s famous 7-1 drubbing of hosts Brazil in the 2014 World Cup semifinal. Brazil had the edge in shots AND possession despite being historically embarrassed in a 6 goal loss. Clearly a new stat was needed and thus the xG boom was under way.
Expected goals is a much more meaningful statistic in its own right. An xG higher than the actual number of goals scored supports the “wasn’t our day” narrative and it also renders the shots on goal meaningless.
For the average fan the eye-test will continue to tell the story and drive the narrative. Any seasoned soccer fan will know whether their team missed a lot of chances and should have had more goals. However, it is nice to know that there is a metric that can actually put a number on that feeling. In other words, you don’t have to be a stat nerd to deepen your understanding of the game. Let the nerds do the heavy lifting. All you have to do is take into account one number and your enjoyment and understanding of the game will be taken to the next level.
Next. 5 underrated performances from Lionel Messi’s Barcelona career. dark