What We Know: The Value Comes From The Questions
By Ian Levy
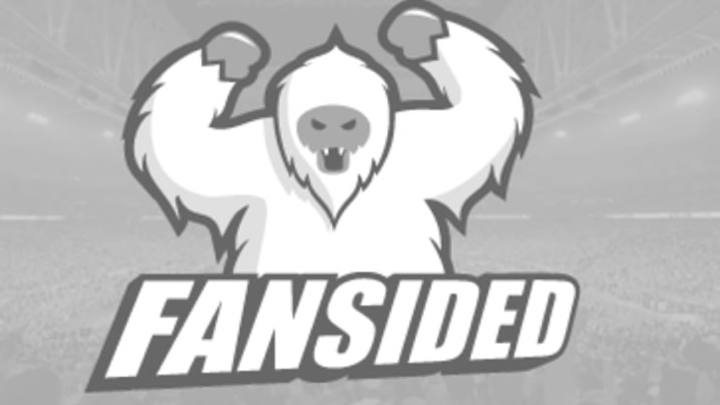
One of our core values here at The Nylon Calculus is accessibility, not just of our content but of the guiding ideas of basketball analytics. What We Know is a series that aims to do just that. We want to press pause, take a deep breath, and recap the ground that has been sprinted over in the past few seasons. This is not about formulas or specific statistics, this is about the big ideas that statistical analysis has brought to the table.
As basketball analytics have continued their steady march into the mainstream, the variety of statistical tools and resources available to the public has grown at an exponential rate. One of the things we’ve been trying to capture here at Nylon Calculus, with our What We Know series and our Glossary (still under construction), is how these statistics are all intertwined and connected. However, for many people basketball analytics are still divided into two groups — basic and advanced — with the only delineation being some vaguely defined date of origination around five years ago. Essentially, if you could find it in the sports page of your local newspaper when you were growing up, it’s basic, everything else is advanced.
Plenty has been written on the argument that advanced statistics are not really advanced, many are simply the same ratios we’ve always seen just with a slightly different denominator.[1. Rebounds Percentage requires nothing more in the math skills department than the simple division used for Rebounds per Game, it just uses opportunities as the divisor instead of games played.] With such a wealth of data points available to choose from, people naturally gravitate towards the favorites. That in and of itself is not a problem, but pair that with this artificial distinction between basic and advanced, and a perception starts to form that statistics can also be categorized as good and bad.
Statistics are just pieces of information, they don’t hold any intrinsic value. When it comes to deciding on the value of a statistic everything is tied to the questions they are being used to answer. For example, trying to determine if a player is good is very different from trying to determine what kind of player they are. Both of those questions are entirely separate from trying to identify the quality of a player’s contributions in a specific game. For each of those questions some statistics would be appropriate for offering useful insights, while others would be inappropriate, sometimes dramatically so.
In my experience, the questions that can be answered with basketball statistics can be group into four basic categories:
- Evaluative: These are questions of quality, good and bad and to what degree.
- Descriptive: These are questions about style, how a team or player functions and what sorts of things they are good or bad at.
- Narrative: These are questions about what happened and why.
- Predictive: These are questions about what will happen and why.
There are questions that overlap between these categories and statistics that serve multiple purposes, but knowing exactly what you’re asking is what determines the relative value of a statistic.
Take for example Pythagorean Win Percentage. This is a projection of what percentage of their games we would expect a team to win, based on their point differential. It is not perfect but, given a healthy sample size of games on which the point differential is based, it has been shown to have a relatively strong relationship with a team’s future success. However, the value of this statistic goes almost entirely to questions that are evaluative or predictive. Pythagorean Win Percentage offers information about how good a team is and how good they are likely to be in the future. It offers precious little information about why a team is good or bad, and the story of exactly how that has manifested over a specific period of time.
Another perfect example is raw single-game plus/minus—a team’s point differential in a single game while a certain player was on the floor. This statistic is incredibly noisy and is often derided as one of the more useless products of basketball analytics. For many questions this statistic is essentially useless—it covers far too many variables to be used as a measure of how good a player was in a particular game and it offers no descriptive information about why things happened. However, as a narrative tool it is absolutely valuable. If the Houston Rockets were +10 in the 31 minutes Dwight Howard played, we can’t assume much about what sorts of things Howard did during the game or how effective he was, but it’s still something that happened during that game and might help explain the story of those particular 48 minutes. Add some other statistics to the mix and a story begins to emerge.
I’m certainly drawing a dichotomous distinction here. Even when a statistic and question are matched appropriately there is still a spectrum of how much information is offered. If you want to measure how good a rebounder someone is, rebound percentage offers more detailed information than rebounds per game. However, rebounds per game offers a different sort of information by factoring in the amount of playing time a player receives—a noisy variable but one that could help answer a question that was framed beyond just “how good is player X at skill Y.” If you really want to find out how good a rebounder someone is, the best way to find a comprehensive answer is to look at multiple statistics, each measuring the skill in a slightly different way.
The point is, there are no inherently good or bad stats, no perfect ones or useless ones either. Statistics can be used badly and carelessly, but the fault is in the application, not the measures themselves. The value of a statistic is always derived from how well it matches the question you are asking, and what other statistics you can put around it to add as much context as possible.