Nylon Calculus 101: Plus-Minus and Adjusted Plus-Minus
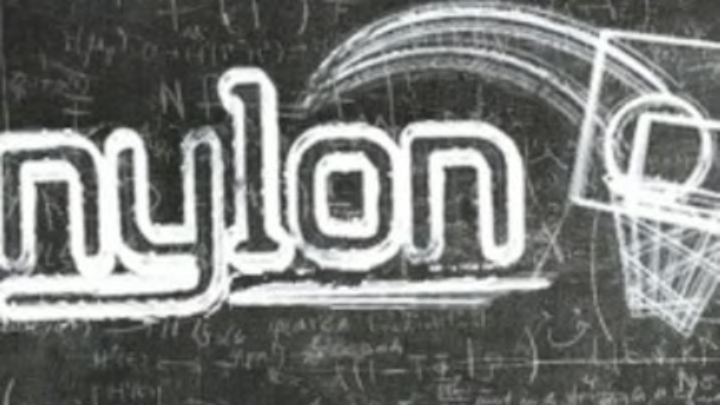
A common lament of fans of any sport is that box scores can only give you so much information. Some of the traditional box score information isn’t even very useful. In basketball, one of the canonical examples is the block. Are blocks really an indicator of good defense? Many blocks do not result in a turnover, and could have little impact on the possession. And yet for decades, steals and blocks were basically the only defensive stats basketball fans had. Naturally, analysts wondered if they could more fully account for a player’s contributions on the court. Enter plus-minus.
Plus-Minus
Plus-minus is a relatively simple stat that is now featured in NBA.com boxscores, among other places (last column):
Calculation of plus-minus is easy. For a given player: count up points scored by the player’s team and points scored against the player’s team when that player is on the floor. Then simply subtract points against from points for. If a player has a negative plus-minus, it means that his team was outscored when he was on the floor. A positive plus-minus means his team scored more points than the opponent while he was on the floor.
This means that the sum of all players’ plus-minus in a game is related to the score. In the game shown above, the final score was 94-83. So the Pacers have a team plus-minus of +11, while the Celtics are -11. If you add up the plus-minus of all the Pacers’ players, you will get +55. This is exactly what you should expect: since there are 5 players on the court at all times, the team’s plus-minus will always be one-fifth of the plus-minus of all players together. Similarly, the plus-minus of all Celtics players will sum to -55.
Why Plus-Minus?
So how does this solve the problem of bad box-score metrics? Plus-minus theoretically accounts for everything the player does on the court. Even if you don’t take a single shot, you might have a strong plus-minus because you are setting good screens for your teammates, enabling better offense, or defending your man well and enabling better defense. Plus-minus can be volatile from game to game, but if you sum a player’s plus-minus for an entire season, you get a reasonably good idea of who some of the league’s top players are. But there are problems. Consider the top 10 plus-minus per game players of 2013-2014:
Reassuringly, Kevin Durant, Chris Paul, and Stephen Curry are in the top 10. But where is LeBron James, and why are there 4 Golden State players? And JJ Redick? The problem is that some players just get lucky. David Lee is not the 5th best player in the league. But he does spend a lot of time on the court with some of the league’s best players, like Stephen Curry and Andre Iguodala. This inflates Lee’s plus-minus, because as a unit Golden State’s five starters generate a fantastic plus-minus. Moreover, Lee gets to play with these players a lot. Golden State plays its starters 18.6 minutes a game, good for 4th in the league among all five-man units.
So the question is, is David Lee an integral part of that unit’s success, or does he just happen to be on the court with four great players? If we took David Lee out of the lineup and replace him with someone else, could that person achieve the exact same plus-minus? This is the question that adjusted plus-minus seeks to answer.
Adjusted Plus-Minus
Plus-minus is an interesting statistic, but you won’t get very far if you insist on wielding it in a debate with an analytics-savvy fan. Adjusted plus-minus, on the other hand, is a truly modern NBA statistic, and almost certainly the best single stat we have for rating players.
The idea behind adjusted plus-minus is that to get an accurate feel for a player’s value, we need to control for the presence of other players, both on offense and on defense. Before we get into the nitty gritty details, consider the general idea. Say you have three players and they are playing in a 2 on 2 basketball game. The plus-minus splits look like this:
P1 + P2 on the court: +10 points
P1 + P3 on the court: +8 points
P2 + P3 on the court: +4 points
Just from looking at this, you might reasonably guess that P1 is the best player on the court, but let’s do the math. This is a system of linear equations in three variables, so we can solve it algebraically to decide who contributed most to the team’s success:
P3 is a +1 player, P2 is a +3 player, and P1 is a +7 player. This is a simple example, but what I’ve done is parsed out each player’s contribution, controlling for the other players on the court. I’ve left minutes out of this but imagine that P1 and P3 play together a lot. This will make P3 look good even though P1 is doing most of the work. P3 is the David Lee of this example.[1. No offense to David Lee, who is clearly pretty talented in his own right, but not Curry/Iguodala talented.]
Calculating Adjusted Plus-Minus
That’s a toy example. How does this work in practice? I’m going to give a very quick overview. If you want more details, check out this article by Jacob Frankel, which really gets into the nuts and bolts. First, we have to define the unit of observation. For most adjusted plus-minus models, a row of data is what most people call a stint. The stint is just a period of time in a game where there are no player substitutions. A stint could be 10 minutes or it could be a few seconds. This is critical to our approach however, since it is similar to the algebraic example I gave above: we need to isolate specific combinations of players.
For each stint, we record the plus-minus for that stint and all the players on the floor. Then we run a linear regression that looks a bit like this:
Plus-minus = Player1 + Player2 + Player3 + … + PlayerN
The player variables here are variables that take the value -1 if the player is defending, 0 if the player is off the court, and 1 if the player is on offense. Once we run this regression on the data, we will get a coefficient back for each player.[2. The coefficients are basically equivalent to the numbers I got when I solved for each player in my toy example.] We can interpret these coefficients as the difference in plus-minus that a given player makes in an average stint.
Regression for fun and profit. Credit: Sega Sai, Wikimedia Commons, http://commons.wikimedia.org/wiki/File:Linear_least_squares(2).svg
We can add a lot of complexity to this basic framework. We could change the unit of analysis, include other control variables (for coaches, playing back-to-back games and etc.) and use weighting in the regression to reflect that longer stints give us more/better information. Another common tweak is to use a technique called ridge regression to adjust for collinearity problems. Collinearity arises when two players on the same team always play with each other or never play with each other. Recall the toy example from above. What if P1 and P2 were never on the court together? This would lead to us having 3 variables and only 2 equations.[2. In effect we simply wouldn’t know that P1+P2=10] If you remember your algebra, that means we can’t solve the system of equations. Something similar can happen with our regression, leading to biased (wrong) coefficients. Ridge regression shrinks coefficients to correct for some of this bias, while introducing bias of its own. On the whole, ridge regression coefficients are ‘more correct.’ When an APM uses ridge regression, as most modern ones do, it might be called RAPM, for ‘regularized adjusted plus-minus.’ Daniel Myers has a nice explanation of how collinearity can bias results, along with a bit more history for APM, on his site.
Why are there so many of these things?
There are a lot of APMs available. The reason is that there are just so many different ways to tweak and play with the basic formula. Here’s a non-comprehensive rundown:
The gambling syndicate Talking Practice has one called IPV
Jeremias Engelmann has created one for ESPN called RPM
Engelmann also has his own variant, xRAPM. Jeremias’s APMs use box score metrics as priors.
GotBuckets.com has a prior informed RAPM that is prepared for them by talkingpractice
GotBuckets.com also has an APM that employs no regularization
James Brocato has both a Prior Informed RAPM and one without priors, although he has not updated these publicly in some time
And this is definitely not a comprehensive list! Some of these account for coaches (basically assigning coaches their own RAPM), some have priors that take previous season performance into account, some try to account for things like fatigue, and some we don’t know much about because they are proprietary. I tend to reference xRAPM and RPM in part because these are just the two variants I know the most about, but most produce very similar estimates.
What is a Statistical Plus-Minus?
A statistical plus-minus is a slightly different kind of adjusted plus-minus that uses RAPM as the dependent variable. In an SPM, box score statistics are used to predict a player’s RAPM. This can be interesting because the coefficients on different box score statistics tell you how important those statistics are in determining a player’s overall impact. As one example, SPMs tend to show that steals make a strong contribution to a player’s overall RAPM. This suggests that steals are actually a pretty good proxy for a player’s overall defensive value.
You can also use the coefficients from an SPM to generate a new rating for each player. This can be desirable because it helps to stabilize RPMs, leading to more consistent season to season ratings for players.
Daniel Myers maintains a popular SPM called ASPM, which you can read about and view on his site.
What are the Strengths of APM?
The single best argument for APM is that it accounts for absolutely everything that goes on on the court. We don’t have a box score metric for making good defensive rotations or opening the floor up for your teammates, but if a player is good at these things, then it will show up in their APM. The discovery of how valuable players like Shane Battier can be is often attributed to APM. APMs also indicate that players like Andre Iguodala, Mike Conley, and Amir Johnson are much better than their counting stats would have us believe, largely for their defensive contributions. This can be a double-edged sword. APMs absolutely love Nick Collison and this remains a bit of a mystery to most people.
Simply put, APMs are the best single number player metric we have. If I want to understand at a glance roughly how good a player is and how he compares to other players, APMs are the first place I go. Other all-in-one metrics, like PER or win shares, do not adequately account for defense, in addition to other shortcomings they have.
APMs are also good for making predictions. Gambling groups like talkingpractice use them to predict team performance in the upcoming season. Importantly, APM is a good predictor out of sample. That is, it is good at predicting things that have not yet happened, rather than simply correctly classifying things that have already happened, like games from a past season.
One of the best things about APMs is that they come with error bands, so you can see how certain we are of a particular player’s rating. More on this in the next section…
What are the Drawbacks of APM?
Before moving on to methodological problems, let me address the big theoretical problem with APMs. They don’t tell you anything about why a player is good or bad. Yes, we can divide the measures into a defensive and offensive component, but why is player X good at offense? Is it because he is an efficient shooter? Because he sets good screens? Ability to create off the dribble? Court vision? We have no idea. This can be frustrating because when we see an outlier on an APM, like Nick Collison, we are left scratching our heads. Does he really do something well that we aren’t picking up on, or is this just statistical noise? Moreover, the idea that a team should just pick up as many high APM players as possible might be a little suspect. Again, because we have no idea why a player has a high APM, we don’t know if he will be complementary to another player.
Methodologically, analysts have taken issue with the lack of consistency across seasons in APMs. A player’s APM can undergo substantial fluctuations from one year to another. These fluctuations can be reduced substantially by using a prior of some sort for the regression. Some priors are based on box scores and some are based on previous year APMs. Of course, some inconsistency should be expected and is probably not a bad thing. Players do change after all, and nobody knows what the theoretically correct level of consistency is. I suspect that a component of this has to do with player interactions. Players interact, and new teammates will change a player’s value. It would be nice if we could capture this kind of thing with interaction terms in the model, but since we’ve never observed Kevin Love playing with LeBron James before, we have no data to judge how they will interact.
A second criticism that is leveled at APMs is that the coefficients received by players are not statistically significant. Statistical significance means that the coefficient lies more than about 2 standard deviations from 0. While it is true that many player coefficients in an APM model are not statistically significant,[2. This situation has improved with prior informed RAPMs and etc.] this is, generously, a pretty silly critique for two reasons.[2. Or three, if you count the fact that statistical significance at the 95% level is an arbitrary threshold.]
First, it’s not clear that 0 is an interesting number in the context of APMs. It’s much more interesting to ask if one player is distinct from another, and because APMs come with error bands, we can theoretically see for ourselves whether one player is better than another and can make a statement about the confidence of this belief. For example, we could say something like “I have 65% confidence that LeBron James is a better player than Chris Paul.”
This is a good thing! Having error bands means we have more information. The second reason that this is an erroneous critique is because it is often leveled by people who prefer single number player ratings with no error bands. It is true that many of the common APMs do not provide their error bands (Jeremias Engelmann does provide them for his model), and this is a problem, but it is superior to not having error bands at all. Now clearly, we’d like to have smaller error bands if possible, and this is where a lot of the methods outlined above are useful, but this remains an important area of APM research.
Consider PER.[2. And I should preface this by saying that I actually like PER, and reference it pretty frequently for a good fast take. Also, to my knowledge John Hollinger has never criticized APMs for failing to reach statistical significance.] Because PER is calculated through a formula, rather than a statistical model, there are no error bounds for it. Does this mean there is no uncertainty about what a player’s PER is? In the sense that PER is a deterministic formula, I suppose you could say that we are 100% certain of a player’s PER, but what if the season was extended by 10 games. Over that stretch, a player’s PER would change, and it seems reasonable to assume we would get a more accurate feel for his ‘true’ PER because the sample size would be larger. So there is in fact some kind of error associated with the measurement of PER, but we do not know what that error is. Not having this error means we have less information about our measurement and we have no idea if the difference between two players is statistically significant. Is the difference between a PER of 18 and a PER of 19 significant in any way? That’s why having a measure of how uncertain we are about an APM estimate is really a strength of the measure.