ShotCaller: Mathematics
By Matt D'Anna
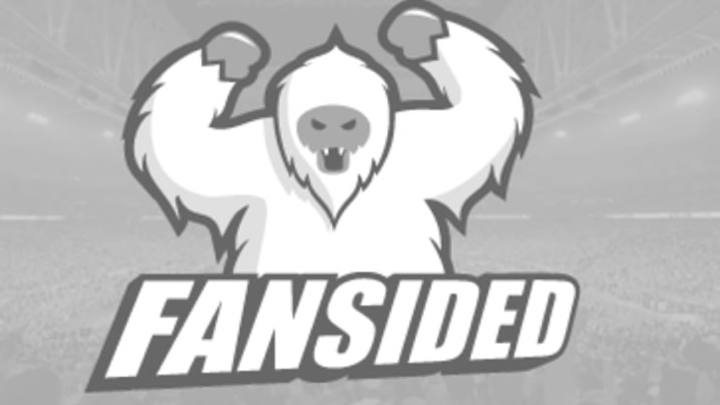
flickr |
This week, I released the 4th prediction in the ShotCaller series. For each of the three previous predictions, I followed up with a quick anecdotal evaluation of the results (as I originally promised). Neat, I suppose. While four predictions is not a lot to evaluate (although by the time of the latest ShotCaller, I had predicted on 1/3 of Melo’s games this season…not too shabby), it’s time to be a little more thorough. Anecdotes are fine, but this is Nylon Calculus; you got to this site because you’re interested in where numb3rs and basketball meet. What these predictions really need is a scientific method for evaluating their utility.
Let me feed the quantitative thirst in your medulla oblongata…without further adieu, here’s my ShotCaller metric:
What does it mean? Well, it’s a single score that measures the accuracy and precision of both components of every prediction: the shot count and the shot locations…
…but what does that mean?
The formula compares the amount of shots predicted versus taken, and the distance from each prediction location to the actual spot on the floor. Here’s what it looks like on the court:
So, if I predict the exact number of shots taken correctly, the first half of the equation gets a 0.5 (which is what happened in the graphic above). If every shot location is a bulls-eye, the second half of the equation is close to 0.5. Only close, you say? Yes, because the size of the prediction areas need to be taken into account. Obviously, the larger the prediction areas, the greater the chance to be “right” (if you remember, I prefer less wrong, but whatever). This is why this concept of “usable court” is introduced; it measures how much of the court available for shot activity is used for the prediction. The point is this: I’m not cheating the system. After a decent amount of thought, this is the best measure I’ve got to objectively compare prediction to reality.
Let’s assume, for now, that this metric is legit. Here’s how I’ve performed thus far:
Let’s examine these results a bit. Of the four predictions (and eight sub-components), there is one bulls-eye: the 6-for-6 shot count for the 1st quarter of the Knicks-Nets game. I’ve never been off by more than four shots (not terrible), and that even includes the injury-riddle-escorted-to-the-locker-room-2nd-quarter of the Knicks-Bucks game (prediction 4). Realistically, I do not expect exact matches every time; however, I do expect to eventually be within 1-2 shots per prediction. The basic rules I’ve been using for shot counts (namely, game-to-game correlation) is decent; however new predictions will need to dig a little deeper.
As far as the spatial predictions, the overall size increases in predictions 3 and 4 naturally; they were two quarters worth. The best indicator of positive learning happening? Those total prediction distances are looking nice, meaning I’m getting closer to nailing these spots on the floor. The point being Hunting Grounds matter. Previous performance versus that opponent matters. Previous activity in the current season matters. It’s not revolutionary, but important: recent previous shot activity – weighted by made shots, opponent, and game quarter – is so far the best predictor of future shot locations.
Bottom line: we are movin’ on up with each prediction. This is consistent with evaluation measures from other social science fields; you expect (and hope) to improve as a study progresses over time. Am I satisfied with a roughly 60% ‘success’ rate? Hardly, but I do like the continued improvement.
Does any of this matter? YES, I would argue; this series is on to something. Player performance is predictable. I am not at the pinnacle of predictive capability (yet), but this process has begun to unlock unique relationships in a player’s activity that can be identified, measured, and exploited. Some of this is very promising; the seemingly significant increase in spatial predictability after only two games comes to mind. Remember, part of this project to see how early into the season (if ever) can a player be close to 100% predictable in their shot selection. Clearly we are not there yet, but with less 1/5 of the season completed we are moving in a positive direction.
Is some of this overkill? Maybe, but how often do people publicly evaluate their forecasts, predictions, prognostications and other outrageous claims? Rarely, if ever; I’d argue that lack of accountability helps foster a general distrust in predictive capabilities. Not here, tho. Going forward you will start to see more multi-quarter (and full game) predictions, as well as examining some other players: a certain sweet-shooting German and record-chasing gunner come to mind. Stay tuned!
Data and photo support provided courtesy of NBA.com, Basketball-Reference.com, and data extraordinaire Darryl Blackport.