Nylon Calculus Talks About Variance
By Ian Levy
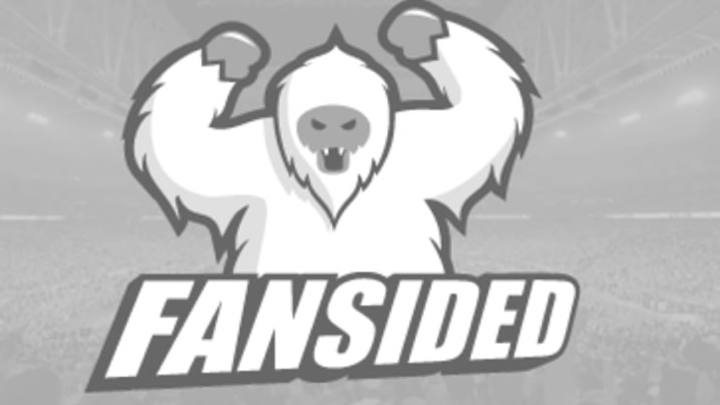
Jan 31, 2015; Minneapolis, MN, USA; Minnesota Timberwolves guard Andrew Wiggins (22) is guarded by Cleveland Cavaliers forward LeBron James (23) during the second quarter at Target Center. Mandatory Credit: Brace Hemmelgarn-USA TODAY Sports
[Ed. Note: We have a daily email thread for Nylon Calculus contributors. There is plenty of organizational chatter (who’s posting what and when), but we also often end up with lively discussions on different statistical points. This conversation about variance, from yesterday’s thread, seemed particularly interesting so we decided to post it in its entirety. Enjoy!]
Layne Vashro: I brought this up once before, but I would love to see a good argument for why game-to-game variance might be interesting.
Should I care if a young player’s peaks are higher, even if the average product is meh? I have seen lots of quotes from NBA guys emphasizing the importance of nights/weeks/months of dominance as opposed to just looking at production over a season. Similarly, big nights play a huge role in popular perception of players. It feels right, but I can’t find a way to convince myself it should matter.
I would be interested in doing some sort of debate article if someone thinks they can make a compelling case for caring about variance. Wiggins, who has had some dominant games and stretches, but ho-hum averages across every season metric makes a great focus for the discussion.
Seth Partnow: The argument for it mattering is playing time isn’t immutable, I would imagine.
Andrew Johnson: I suppose the idea is that the highs represent ‘potential’ more than the average for a young player. And it is more likely they will clean up the mistakes than a steady mediocre player will increase their highs.
If you think of players with the same average efficiency with very different SD’s to their play. It might make sense that both players will develop towards their +1 or +2 SD mark rather than a even movement of the average going up.
Hal Brown: I actually found out a lot of stuff about the value of variance in role players in that cluster analysis. I hope to have it written up this week but I still have to smooth out the edges.
The basic result was that variance and consistency seem to be good ways to separate out different types of role players. Most notably among those role player clusters, the “high variance” role players have a far higher average RPM, SPM, and PER than the other groups. Whether or not this means “higher variance is preferable for role players” or “the players naturally have a higher variance because their role is already bigger” is sort of a chicken-or-egg question, but I tend to think it’s the former because a) variance doesn’t have a strict relationship with minutes, usage, or time of possession, and b) if, as someone suggested on twitter, you wanted to find a “team’s best possible scenario” and “team’s worst possible scenario” using variance, you’d want mid-variance stars but high variance role players to maximize potential there, I would imagine.
But I also could be totally wrong.
Seth Partnow: You might be catching effects of coaching or good team play. If the guys who are “positive floor time” guys have high variance, their involvement may be higher in more advantageous situations rather than constant
Hal Brown: Yeah, though there isn’t much of a relationship between minutes consistency and variance either. But I’m thinking that too, that the naturally better role payers are given both varied playing time and situations that change contextually, as well as are given more free reign to act in “high variance” behavior.
Layne Vashro: Here is the simplest way to phrase my problem with the variance idea:
Nearly everything we do with analytics breaks things down to the level of a possession or instance of event. We do this for a good reason. Despite constant protestations to the contrary, nobody has been able to defend the idea that success/failure on a given possession feeds into the next. To the extent there is evidence for the “hot hand” and related effects, it is extremely weak.
Working from this assumption… what the hell is “variance in performance”? Typically we are talking about games, but there is no good theoretical or empirical argument for treating those particular 100-ish possessions as a unit rather than some other randomly selected cluster of an equal number of possessions.
So… what are we capturing with variance? Well, guys who carry a high usage, especially if they do lots of shooting (which is noisy) are going to have more extreme clusters giving them higher peaks and lower troughs relative to a low-usage specialists with similar average performances. Alternatively… it could just be a sloppy way of identifying improvement. It isn’t variance that is interesting, but that there is a positive slope within that variance.
Hal Brown: My problem with that, though, Layne, is that games aren’t a totally arbitrary way of separating out performance, largely because those games are a unit that ultimately defines success and failure. Using “per game” as a way of judging player performance generally doesn’t do much for all the reasons you pointed out, but if each game is a unit of success or failure, there’s value to be had in seeing how things vary from unit to unit, or how likely you are to get a contribution to a success or more to failure on any given game. There’s a give and take on how much value we can get from considering game-to-game performance, but I don’t think variance is useless because games are arbitrarily delineated, because they’re not.
Kevin Ferrigan: I like Tim’s point there and it goes to simple idea that the point of the games is to win.
Layne Vashro: Sure, games are uniquely interesting in a descriptive sense. However, based on what we know about the independence of possessions, there isn’t any good reason to expect variance in games to predict future variance in games.
Do we agree on that? Is there a reason to believe a guy who scores 15 on 15 shots in each of two games has less/more potential than a guy who misses all 15 shots in one game then hits all 15 in the next? Additionally, I assume nobody thinks the mean expectation for the third game is different, but does anybody think the errors should be seen as any different?
Matt D’Anna: To that, I would say only at the individual player level. If you are trying to predict how many shots Player A is going to take in his next game, his prior games will certainly inform on that decision, as well as games against that opponent, lineups, interval of the season, etc.
Comparing across players and using the stats from Players A, B, and C to predict Player A’s shots would not work IMO. Your crossing signals at that point.
At the individual level, it becomes a question of unique decision-making processes. The more we can identify those decisions, by enriching each possession/shot, the easier to identify them.
I’m talking at the very game-to-game level tho, not at necessarily from college to pro.
Hal Brown: Well, and I don’t even know that this has value predictively. But we can use variance to say what the range is in game to game performance and I think it’s silly to act like that doesn’t have value.
Layne Vashro: Certainly in some contexts, but if you are using the information to make decisions for something like team-building or gambling, it doesn’t have value unless it is predictive.
Hal Brown: Disagree in the sense that if we learn that, for example, you can maximize instances in which a team plays its best possible game by maximizing and minimizing variance in some players, that has team-building value even if it’s not strictly “predictive.”
Andrew Johnson: Game level might be a sample of convenience, but I don’t think that necessarily invalidates looking at that level.
Layne Vashro: I have some more on this…. but Superbowl festivities await. Thanks for humoring me guys. Have a good one.