Demystification of DIY — Defining Basketball Analytics Down
By Seth Partnow
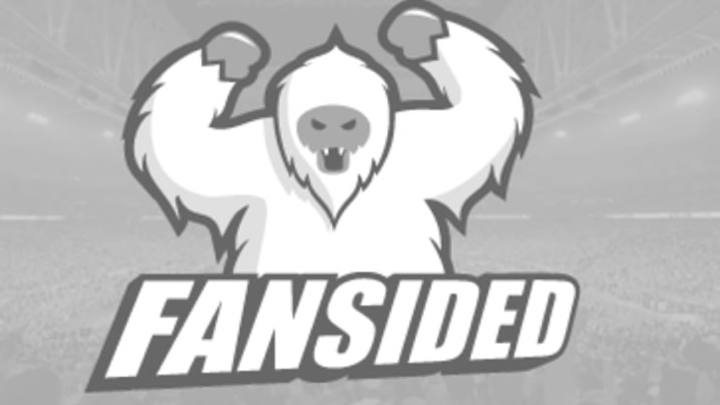
You don’t need much more than this to do basketball analytics. (Image via Wikipedia Creative Commons)
The furor over analytics (re-)sparked by Charles Barkley’s pre-All-Star Week tirade has gotten us here at Nylon Calculus talking. Moving past the immediate, defensive reactions to Barkley’s particular perspective[1. I could link to any of two dozen pieces, but it’s kind of fish-in-a-barrel stuff, really.], there was something to be taken from the discussion. Barkley and many others don’t really know what “analytics” entail. Partially, that’s an error on their part, being paid to observe and comment on the league as they are.
But it’s also on “us,” the numbers-driven community. While things like points, assists and rebounds are analytic tools[2. Though highly imperfect as you no doubt know if you’ve found your way to this website.], they are digestible, non-threatening, and perhaps most importantly, easily related to basketball as viewed – LeBron James scored two points on that dunk, sweet dime by Ricky Rubio, Andre Drummond had twenty rebounds again. There’s a reason for the dominance of box score statistics that goes beyond sheer repetition and tradition. They are basketball.
On the other end of the spectrum are highly involved math-and-formal-statistics intensive systems. These produce things like ESPN’s Real Plus Minus, or Andrew’s Player Tracking Plus Minus, and form the basis of many of the research papers forthcoming at Sloan. These methodologies have their uses[3. And their flaws; the first half of this podcast featuring the eponymous Talking Practice contains a great deal of information about what such models can and equally importantly can’t tell us.]. But they can be technically daunting, prone to misapplication and interpretation[4. Repeat after me, RAPM IS NOT RANKINGS. Thank you.], and exceptionally difficult to differentiate in lay terms. Hell, the sentence explaining their limitations is daunting.
And it’s a key point; how is a basketball lifer with a different approach to the game, or even a more willing student, to decide which set of black-box numbers to believe?
That there is perceived to be a wall between the two camps, or between numbers-intensive analysis and game film-based scouting, then, is largely a failure on the side of analytics. Contrary to the beliefs of some voices hollering in the wilderness, the professionals were doing things pretty ok before the quants came along. There were certainly areas for improvement, but they weren’t just throwing darts. Most of the players believed to be great under the new school were recognized as such by the old school and vice versa. Traditional scouting has done a reasonably good job, on aggregate, of slotting players into the right order in the draft.[3. Any sort of measure of historical production by draft slot drops steeply the higher the number of the pick.] The new methods must be demonstrated to be an improvement. The onus is on the new methods to explain themselves.
In the legal arena this is known as the burden of persuasion. Persuasion. Yet the language used is often more exclusionary than inviting. “Metrics,” “studies,” and “models” are fancy sounding words that serve to simultaneously make the achievements seem more impressive but also less welcoming. The use of this terminology is understandable: describing something as a “metric” rather than just a “stat” is meant to imply a certain progressiveness of thought, a signifier that I’ve moved beyond “Yay, points!” as a good means of judging talent. To some degree, it’s perhaps a more accurate use of the language. But we probably go too far too often and end up sounding more than a little douchey to the not-already-converted.
Perception is a two-way street, after all.
It’s also important to be clear on what analytics is not. It’s refinement, not reinvention. For as much as Barkley and other commentators bemoan the lack of a midrange game, I think they would agree with this fundamental notion: teams should get good shots. Layups and dunks are good shots. Wide open three pointers are good shots. We don’t need MoreyBall to tell us this. Moreover, the “insight” that a midrange shot is lower efficiency than a dunk tells us virtually nothing about how to produce more dunks from an offense.[7. Aside from signing Hassan Whiteside. That seems to help.] When Hall of Famers scoff at the notion that the midrange is somehow less than ideal, they’re not scoffing at the idea that teams should take good shots. They’re amused and probably a little pissed off at the idea that getting to the rim is somehow easy. Efficiency is difficult to come by; no one knows that better than a 6’4″ power forward who made a career of fighting for rebounds — and, somewhat incongruously, taking a lot of 3s.
Further, while some of the insights coming forth are truly PhD-level[6. In terms of math or computer science. Basketball subject matter expertise is a whole other beast.], most of it isn’t that hard. At least, not that hard from a technical or mathematical perspective. It’s much more about the logic. “What question am I trying to answer?” is often the most important question, followed closely by, “Do the tools I’ve chosen answer that as well as possible, given what’s available?”
Or to put it more simply, the difficulty isn’t in the math. It’s the basketball, silly.
If a stat/metric/number/analytic/gizmo can’t be related back to basketball in a recognizable way, there is no good reason for someone already knowledgeable of the game to accept it. To paraphrase an old saw, there are lies, damned lies and bad analytics. Even a properly specified, rigorously tested and logically sound model that we as analytics types know is meaningful is a lot to digest from a cold start. An analogy I’ve used before is one does not learn the guitar by playing Jimi Hendrix licks, rather working their way up to that point. And that ramp up is maybe where the “community” has lost its way a bit.
Our founding mission here includes offering up numbers-based work from the perspective of both the rocket scientist and the raconteur. The deep-dives and the quick hits. As is perhaps too often the case in the community, we’ve erred a little on the side of the abstruse. [Ed. note: Mostly by using words like abstruse, SETH.] Part of allowing a more inviting point of entry into a quantitative mode of enjoying the game,[4. a mode which is by no means mutually exclusive with aesthetics-based fandom. Follow any of us on twitter and you’re likely to see me making horrible puns, Kevin bashing Pau Gasol and Bulls management equally or Matt trying to keep good humor amidst another season of bleh in Minnesota. We’re fans of the games as games not just as number-generation machines.] is demystifying this stuff.
For example, almost nothing I publish here involves anything more conceptually difficult from a numbers standpoint than compound multiplication and division. Partially, this is because I’m more interested in discrete areas of study like “who’s getting the most open shots?” or “which players have been most effective defending the paint this season?” — the kind of thing you’d talk about over beers with a friend.
For the purposes of fan-serving analytics, good enough really is good enough.[7. Also, the “perfect metric” in my experience often calls for data not publicly available or at least not easily collated.] Most analysis should not be taken as a rank ordering, but rather more of a tiering system. While Rudy Gobert has been at the top of the Rim Protection charts by my methodology over the course of the season, I don’t think it’s a demonstrated fact he’s “better” than Roy Hibbert. He may have performed better in certain ways over the course of the season[7. The difference between “a player did this” and “that player is this” is a much broader topic for discussion.], but both have been very good and any direct comparison is best left there. It’s an application of the 80-20 rule, in that a good first approximation of the answer being sought is often available reasonably quickly, but that last fine tuned bit of accuracy is going to take a disproportionate amount of work. But, again, that 80% answer is often a pretty good one.
And that’s true here. This post isn’t an attack against anyone; if anything, it’s the extension of an olive branch. We all love basketball; if there’s been confrontation, it’s in the past.
But if you want to dive a little deeper and take a peek behind our curtain, keep reading, because there’s some fun stuff ahead.
Even internally here at Nylon Calculus, we often mistakenly see wizardry where there has simply been thoughtful application of tools and questions. In examining how the addition of a third ball-hungry guard had disrupted Phoenix from last season to this, Jacob was momentarily taken aback by a stat I’ve been using for Time of Possession %, or a different way of looking at how much different guys have had the ball in their hands on offense. He wanted to know how I calculated it and was surprised to learn it was simply SportVU’s time of possession divided by minutes played to allow for a meaningful comparison across players with different minute loads. A simple calculation for a simple, descriptive stat which allows a rough measurement of how often various players possess the ball. Complexity and usefulness don’t always go hand in hand.
Prior to start of the 2013-14 season, so much of the analysis focused on the in-depth because the existing “raw” stats (primarily from the box score) had been so well picked over. True Shooting Percentage can only be invented once. But, with the release of building block level information from SportVU and Synergy and Vantage, not to mention the ever-growing array of filters and sorting tools for more traditional stats available on NBA.com, there are so many more possible relationships to examine. Even with the public-facing information on all those platforms being the mere tip of the iceberg, the amount of raw material at our fingertips is exponentially greater than it was two, much less ten, years ago.
The raw data might not tell us much in itself. It takes some work, both computationally and logically to get from a simple count of a players’ touches to something descriptively or evaluatively useful.[10. Not every metric has to stop plate tectonics on its own to be useful.] I quite like this taxonomy of types of metrics from a piece on building a data-driven baseball organization:
- Descriptive analytics: Data that describe what has happened or is happening — essentially, reporting. In baseball, think “the back of the baseball card.”
- Diagnostic analytics: Data that show a potential relationship between two or more variables and help explain why something happened.
- Predictive analytics: Data that are used to predict future events. As Ian said here, picking the right data to answer the question you are looking at is the trick.
I could talk around this for far longer, but it’s probably best to give a practical demonstration of a few things[11. And hey, drop some metric bon mots on you as well as a reward for making it this far.].
The other day, I tweeted out this chart talking about the league leaders in offensive rebound “chase percentage” through the All-Star Break:
This is meant to represent how often a player “crashes the offensive glass.” That’s all it is! We know some guys — some teams — crash the offensive boards more than others; this is just a number for that. It’s capturing what has happened in the game in a more exact manner and answering the question, “Who’s really attacking the offensive glass?”
And it’s interesting for a few reasons. Offensive rebounds are good. They get a team extra possession and often easy baskets off of tip-ins or put-backs. On the other hand, being too aggressive in pursuing offensive rebounds is bad, because it allows the opposition to fast break more and get their own easy baskets. So in addition to the number of offensive rebounds a player secures, it’s nice to know how many they go after as a means of comparison, and, by extension, how many times they’re playing that risk/reward of offensive rebound vs. a potential break the other way. It’s the same question asked by teams that eschew offensive rebounds to prevent transition opportunities.
The chart above seems precise, thanks to the small magnitudes of change between different players, but it’s just an estimate. I haven’t charted each shot by hand, after all, so we’re forced to rely on some proxies for our data. In that case, how do we go about figuring this kind of thing out?
First, we need some raw measure of how often a player goes for offensive rebounds/ Thankfully, this information exists, in the form of SportVU’s rebounding data:
One of the data points is “rebound chances.” This sounds promising, but what does it mean?
That’s helpful! And it’s worth noting that since SportVU marks a player’s position on the court as the location of their torso, this basically means times a player is within arms’ reach of where the rebound is secured. Also important: this is a highly imperfect method of measuring rebound attempts. Sometimes a player is close to the rebound by happenstance while making no real effort to secure it, while (probably more frequently) a player pursues a rebound but the ball bounces a different direction. So “rebound chances” simultaneously over and undercounts what we might term “rebound attempts.” If we were laboriously watching every possession of every game[11. Which would add up to about 250,000 possessions over the course of a full season. Have fun and make sure your insurance covers vision.] and recording via some definition of actual attempts, we’d get different numbers, and that’s important to always keep in mind at this stage in analytics; we’re dealing with very good estimates that are nowhere near perfect. But it’s far better than nothing, and as long as we know what we don’t know, it’s entirely appropriate to make a “best guess” based on available information.
By now you might have recognized another problem with this data point. It’s recording ALL rebound chances, not just for offensive rebounds. Thankfully, much more data is available from the league than is currently shown on NBA.com. Without going into laborious detail on how to get at it[12. But check out this article by Greg Reda for a primer.], the raw data is available at here. In Excel, here’s what it looks like:
There’s a lot more there than displayed on NBA.com. Including offensive rebound chances! So we know how many offensive rebound chances each player gets per game by the above definition. Now all we need to determine the percentage of his team’s misses is the number of those misses while he’s on the floor. And here, we’re in luck! Among the first wave of “advanced” stats for the NBA was rebound percentage, which is split into both offensive and defensive rebound percentage, so the number we need is right there on NBA.com!
Since offensive rebound percentage is offensive rebounds / total available offensive rebounds, and we know both the number of rebounds collected and the percentage, we can simply flip the equation and find Available OReb = Orebs/Oreb%. This number of available offensive rebounds then becomes the denominator in determining a player’s “Chase %.”
While the above process is slightly convoluted and more than a little laborious[12. Also it is illustrative that finding, collating and cleaning the raw data is often the hardest part of the analysis. At very least, facility with a spreadsheet program like Excel is a must, and even better is familiarity with a stats package such as R. Big, BIG fan of R. After learning some basics of the programming language, analysis which took several hours to complete purely in excel is largely automated and takes maybe 10 to 15 minutes to tweak using R.], there is nothing that requires any math past about 10th grade level. And if you’re unfamiliar with those concepts, that’s okay; that’s why nerds exist, and we know that you know that we know how to do math. You can trust us on that part. The more important part was asking the right question and identifying the sources for the data which could provide an answer if not the solution for examining players’ relative propensity to crash the offensive glass. It is also a demonstration of the power of combining multiple data sources to best address the issue. The math is unimportant; it’s about the right basketball questions and using the right tools — among those we have available.
Okay, let’s do one more.
As a further example of how additional sources of data can help refine and improve analysis, consider early shot clock defense. Earlier this season, I looked at how various teams were successful in using the shot clock on defense, by not allowing early shots and forcing the opposition to work against both defenders and time to find a makeable shot. A seemingly odd result to come from that analysis was that a couple of very good defensive teams in Atlanta and Golden State allowed more early shots that I was expecting to see:
In part, this result reflects that the initial analysis was just looking at time on the shot clock. One of the primary weaknesses for both the Warriors and Hawks is defensive rebounding, as they rank 21st and 22nd in the NBA as of this writing in DREB%. With these offensive rebounds allowed come putbacks, presumably early in the shot clock. This basketball concept skews the data; without a firm understanding of what’s actually going on during the game, the numbers become much less useful. If what the analysis is attempting to show is which teams play good “early” defense by not allowing teams to get out on the break or to get easy shots of their first or second offensive options, tap-ins and the like don’t really fall into that category of defensive breakdown; instead, they’re their own problem, and should be treated as such.
However, with the release of Synergy play type data, this can be accounted for. In Synergy, putback attempts occur “[w]hen the rebounder attempts to score before passing the ball or establishing themselves in another play type.” Thus, by subtracting these putback attempts and makes allowed from the early shots category listed above, we can get a better sense of which teams (like Atlanta) really do defend well early in possessions:
As with rebounding chase %, this is imperfect analysis. The definition of putbacks means there are still some possessions where the offense gets an easy shot in one or two passes from an offensive board that aren’t being accounted for, and this is possibly still “penalizing” the early defense numbers of teams which aren’t stellar on the defensive glass. But that factor is at least partially controlled for, so while not perfect, it’s better.
Again, nothing much more involved than simple algebra once I identified what I wasn’t already accounting for and how I might go about doing it. Did it all take some time, thought and elbow grease keystrokes? Absolutely. But it required very little in the way of high level math or programming skills. Basically, my message is you can do this. If there is a question that intrigues you, dig into the data yourself and try to find an answer. Sometimes you won’t find what you are looking for, but even when that happens you might discover something completely unexpected by accident.[14. The discovery that corner threes tended to be more open than above-the-break was a total accident. I was trying to determine which players shot the best from the corners when completely unguarded and discovered that seemingly every player was unguarded on a very high proportion of their corner three attempts.]
In baseball, the statistical revolution was driven in large part by everyday fans asking themselves “what about…” The tools are out there for basketball fans to do much the same. It’s an important part of our charter at Nylon Calculus to assist that journey of discovery and where possible to help publicize important, interesting and relevant results.