Week 22 in Review: Light at the End of the Tunnel
By Justin
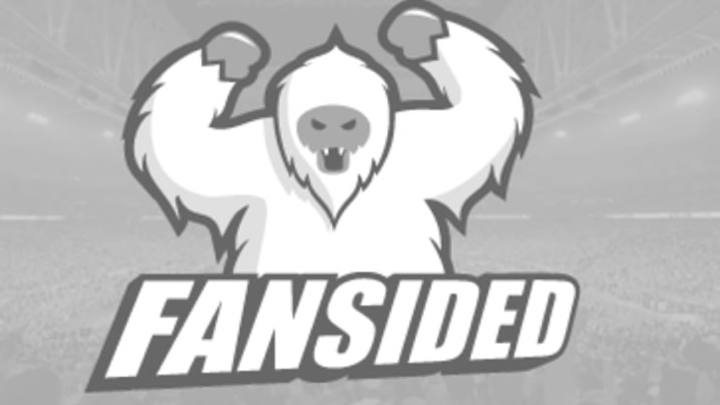
Mar 30, 2015; Charlotte, NC, USA; Boston Celtics guard Avery Bradley (0) drives the ball inside passed Charlotte Hornets forward Jason Maxiell (54) during the second half at Time Warner Cable Arena. The Celtics defeated the Hornets 116-104. Mandatory Credit: Jeremy Brevard-USA TODAY Sports
At this point in the season, the NBA is all about seeding implications and last minute playoff spot battles. Some teams, like the Golden State Warriors and Atlanta Hawks, will just be going through the motions until the post-season is upon us, but there’s still a lot of shuffling for seeds 2-7 in the Western Conference and for the bottom six slots in the Eastern Conference. But even for teams like the Philadelphia 76ers, who as a whole shouldn’t even be attempting to win given their status, the games go on, and the players, thinking about their own futures, will keep fighting and showing us what they have. There’s a light at the end of the tunnel for this season, and it represents something different for every team.
Boston: Scrapping Its Way into the Playoffs
Last week, we had a fairly important game for the bottom-feeders: the Boston Celtics defeated the Brooklyn Nets, which could have huge implications for the last few seeds in the east. Right now the two teams are nearly even, and while that’s not notable for the expensive payroll (Brooklyn) it’s impressive how good the Celtics have been given their raw talent. Boston’s main options this year have been Jared Sullinger, Avery Bradley, Evan Turner, and Brandon Bass. Before the trade for Isaiah Thomas, who hasn’t even played 400 minutes for them, they really had no traditional lead ball-handler and creator. Yet their offense is only a small distance from league average and their defensive efficiency is rated as 14th in the league, per Basketball-Reference, despite a short frontcourt without anyone resembling a rim protector. Their best defenders are probably in the backcourt: Bradley and, quite possibly, the rookie Marcus Smart. There are no stars on the team, and on paper this looks like a high lottery team, so what are they doing right?
Broadly speaking, they do a number of things well with few glaring weaknesses besides an inability to get to the line. They are a three-point happy club without any credible threats from outside, with the exception, maybe, of the newly acquired Thomas. In fact, their frontcourt often has better outside shooting than their backcourt, which has Bradley, Smart, and used to have Rondo. Sullinger is out for the season, but they still have Kelly Olynyk and they use Jonas Jerebko as a stretch power forward. You can see the problems this creates when they have an outside shooting center via recent win over Brooklyn: here’s a play where Olynyk is twice the target of a pick and pop play (the first instance was nixed by a bad pass) where the defending center, Plumlee, is trying to corral Evan Turner, leaving Kelly Olynyk open. Yet if you do stay close to Olynyk, like Brook Lopez does here, you can concede an open driving lane and you no longer have a 7-footer near the rim. You can do all sorts of thing with more space, like during this semi-transition play where Jerebko sneaks to the rim because one Brooklyn defender was late and every Boston player was hanging out at the three-point line. With some real offensive firepower in the form of an elite ballhandler and at least one elite shooter, that kind of offense can be especially potent — just look at Atlanta.
On defense, the team is bizarrely average given their non-traditional set of players. It was difficult to find a defensive stat where they were in the top five or the bottom five. They’re 13th in effective field-goal percentage, 14th in turnover percentage, 15th in defensive rebound rate, 16th in free throws per field goal attempt, 17th in giving up shot attempts at the rim, and 22nd in three-point rate (not percentage.) The only top five category I found was proportion of field goals given up between 10 and 16 feet: 5th, which is a pretty good rating because it means you’re giving up more of these low percentage shots. They do have some interesting defensive pieces, particularly in Marcus Smart. You can see him here shadowing Deron Williams, taking away passing angles, and coming up with the steal — he’s one of the better rookie defenders at guard we’ve had in years. Tyler Zeller is better on defense than I expected; here’s a play he reads correctly and comes up with the block, although they fail to secure the miss. His rim protection numbers are nothing special, but he’s no disaster either and that’s apt on the “league average” defensive squad. One would probably use the word scrappy to describe their defense overall. You see a lot of scrambling plays like this one where Evan Turner gets a block at the rim to cover for his teammates or this last one where Brooklyn can find a good opportunity while the Boston players are synchronized in their efforts to seal driving lanes and stop open three-pointers. Most teams with Boston’s talent would rather tank for a better lottery pick, but they’re fighting with Indiana, Brooklyn, Charlotte, Miami and Detroit for one of the two last spots, and they’re doing it with execution and unorthodox lineups. Let’s hope that if they make the playoffs they meet Atlanta, another non-traditional, and don’t get steamrolled by Cleveland.
Mar 25, 2015; Memphis, TN, USA; Memphis Grizzlies center Marc Gasol (33) drives against Cleveland Cavaliers forward Kevin Love (0) in the first half at FedExForum. Mandatory Credit: Nelson Chenault-USA TODAY Sports
Love’s Low-Post Defense
Some players can play bad defense and not get called out for it, while others, like Kevin Love, often receive an undue amount of criticism. It’s true that he’s a poor defender, but he’s not an outrageously awful liability, and I wouldn’t peg him as the limiting cog in Cleveland’s defense. For one, a frontcourt of him and Pekovic played better defense last season as measured by team defensive efficiency. (Love’s back issues, or whatever else is plaguing him, could be affecting him, however.) But Love can do a few useful things on defense, like rebounding, taking a charge every now and then, which is quite valuable, and defending the low-post versus strong guys. If Love is truly the leak on defense, then Memphis should be have crushed Cleveland, but Love defended the taller Marc Gasol and did decently well, while Cleveland blew them out. Here’s one play where Love holds his ground as Gasol posts him up, which leads to a missed sweeping hook and a Love rebound. Versus players more his size and not too speedy, Love does quite well — here’s one against David West, who fails to create a good opportunity and misses the shot, and then another where Love gambles up high but stays with West to the basket and stops an easy attempt. In the playoffs, Love will have a target on his back, but attacking him through the low-post is not a smart idea.
Mar 27, 2015; Brooklyn, NY, USA; Cleveland Cavaliers forward LeBron James (23) looks towards forward Kevin Love (0) against the Brooklyn Nets during the second half at Barclays Center. The Nets defeated the Cavaliers 106 – 98. Mandatory Credit: Adam Hunger-USA TODAY Sports
Dynamic Point Differential
People who pay close attention to NBA stats probably know the power of point differential in explaining wins for teams. There’s this heavily used formula where you take the points a team averages per game and the points their opponents average and use it like this: Games * PTS^14 / (PTS^14 + OppPTS^14). It’s the basis for Basketball-Reference’s well-known SRS and Pythagorean win estimates. Of course, as with every method it’s not perfect, but there’s one little kink people should keep in mind.
Let’s say a team has a Jekyll and Hyde-esque season. For the first half, they’re almost exactly average, and the during the second part they take off and outscore teams by about 10 points per game. This happened, by the way — split Cleveland’s season by their first 38 games and their next 36. Given their point differential over those 74 games, you’d expect 49.0 wins. However, if you just look at the first 38 games you’d expect 18.9 wins, and if you do the same for the next set of 36 games you’d expect 28.8 wins for a total of 47.6 — note the disparity between that and the aforementioned 49 wins. Why does this happen? Because the win formula is non-linear, and every unit of point differential for mediocre teams has more of an influence over wins than it does for elite teams. In other words, wins are more sensitive to point differential when you’re average. For a team with an opposite path — average for one half and awful for the other — they’ll typically win more than you’d expect from a full season point differential, not fewer like Cleveland. This effect is pretty minor in most cases, but it’s worth keeping in mind for those teams with weird seasons.
Cleveland, by the way, won 47 of those 74 games, not 49.
Mar 4, 2015; Minneapolis, MN, USA; Minnesota Timberwolves forward Kevin Garnett (21) looks on during the second half against the Denver Nuggets at Target Center. The Nuggets won 100-85. Mandatory Credit: Jesse Johnson-USA TODAY Sports
How Players Respond in Comebacks
Some players get tagged with a “loser’s” mentality or a characterized as a fake superstar, unable to carry his team or lead them when it matters, so to speak. There’s a lot to unpack there psychologically about which players are perceived as alpha and what factors matter, but the winning bias is fairly important — if a superstar’s team fails to pile up wins, especially in the playoffs, instead of finding problems elsewhere we retroactively change the evaluation of the star and write in new critiques. For example, we may decide that a player is unable to push his own teams forward while they’re down. This happened a lot to Kevin Garnett, who did just about everything in Minnesota but it wasn’t enough. Based on some new research by someone on the APBR board, we have data on how every player since 1997 scores when the team is behind. As you can see from the charts below, Garnett usually shoots much more often when his team is down, while someone like Kobe, who is well-respected in his ability to “carry” teams on offense for a comeback, actually shoots a little less often when his team is behind. Of course, there are a lot of other factors here, but it’s interesting how many narratives the media create that are completely unsubstantiated, and in a world of data we can tackle these mountains of ignorance.
Best Coaches out of the Timeout
There are few ways to understand coaching objectively, but one I’ve always been intrigued about is how well they do coming out of a timeout. Using the team data from NBAWOWY, I included a table below showing a team’s overall effective field-goal percentage and their effective field-goal percentage after a timeout. Theoretically, this should measure a coach’s direct influence over the game because he typically has more say after a timeout than during the normal flow of the game due to a number of factors like broken plays, fast breaks, and stars chucking their way to the box score line. Specifically, this is a measure of a coach’s X’-s and O’s and what he can add to the team’s scoring power. But in practice, it could mean very little — look at that table closely.
Team | Overall eFG% | Timeout eFG% | Diff. |
PHI | 46.0 | 47.9 | 1.9 |
SAS | 51.2 | 51.7 | 0.5 |
NYK | 47.0 | 46.5 | -0.5 |
PHO | 50.5 | 49.8 | -0.7 |
NOP | 50.0 | 48.1 | -1.9 |
ATL | 52.7 | 50.8 | -1.9 |
CLE | 51.8 | 49.5 | -2.3 |
SAC | 48.4 | 45.7 | -2.7 |
HOU | 51.0 | 48.3 | -2.7 |
BRK | 48.9 | 46.1 | -2.8 |
OKC | 49.1 | 46.2 | -2.9 |
IND | 48.1 | 45.0 | -3.1 |
CHA | 45.8 | 42.2 | -3.6 |
GSW | 54.0 | 50.4 | -3.6 |
CHI | 49.0 | 45.1 | -3.9 |
MEM | 49.0 | 44.9 | -4.1 |
DAL | 51.3 | 46.6 | -4.7 |
DET | 47.7 | 42.4 | -5.3 |
TOR | 50.6 | 45.0 | -5.6 |
LAC | 53.3 | 47.4 | -5.9 |
MIN | 46.7 | 40.3 | -6.4 |
WAS | 49.8 | 42.5 | -7.3 |
BOS | 48.5 | 41.0 | -7.5 |
MIA | 50.3 | 42.7 | -7.6 |
ORL | 49.6 | 41.8 | -7.8 |
LAL | 47.3 | 39.4 | -7.9 |
POR | 50.6 | 42.1 | -8.5 |
MIL | 49.8 | 41.0 | -8.8 |
DEN | 47.8 | 38.4 | -9.4 |
UTA | 49.2 | 39.8 | -9.4 |
If you can find power in all that madness, then kudos, because there’s not much of a pattern. Sure, the Spurs and Hawks are ranked high, but so are the 76ers and the Knicks. You have a couple of well-managed teams near the bottom in the Blazers and Bucks, and one coach known for his cleverness (Carlisle of the Mavericks) is somewhere in the middle. Again, this isn’t measuring every part of coaching, especially defense and player management, but there should be something of use here. Perhaps we need more seasons or we need to parse this by variables like seconds left on the clock and players on the court. But for now, I’ll leave this vexing coaching stat to the world and perhaps one day it’ll have some utility.
Mar 25, 2015; Orlando, FL, USA; Orlando Magic guard Elfrid Payton (4) and guard Victor Oladipo (5) high five after he made a basket in the act of getting fouled against the Atlanta Hawks during the second half at Amway Center. Atlanta Hawks defeated the Orlando Magic 95-83. Mandatory Credit: Kim Klement-USA TODAY Sports
Elfrid Payton and the Magical Hair
Payton is by no means a traditional player, and his hair moves like a non-Newtonian fluid, but at the very least he’s an intriguing project in Orlando. For those unfamiliar, he’s a 6′ 4″ point guard with good defensive instincts, plenty of quickness, deft passing skills, a mop of hair on top, and no jump shot. Given that Oladipo is another ball hungry guard with no outside touch, it’s unclear how Orlando will proceed. He’s essentially a Rondo clone, including the poor free throw shooting. It’s one thing if you need work on finding the NBA range; it’s another if you’re still dealing with the concept of an outside shot. Yet the Magic play better on both offense and defense when he’s on the court and he’s embarrassed a few teams already. He’ll probably never receive the attention Wiggins has now, but he’s someone to track in the future.
You can see Payton’s skills from last week’s game against the Hawks, who were losing to the Magic as late as 5:00 in the fourth quarter. Payton’s a high-caliber ball thief, and on this play he quickly darts over to Carroll in the corner, thwarting an open shot, and stays so close to him on a drive that he swipes the ball away and somehow manages to secure it. Against Teague, he was an absolute pest, sticking with him through this entire play, stopping a drive to the basket, and forcing Teague into an awkward lean-in jump shot. He has the chops to drive on an NBA defense too, as you can see in this clip, where he seems to grasp when to attack, when to pass, and how to find the appropriate angle — he just needs to polish his finishing skills and cut down on the mistakes. He’s also a surprisingly good offensive rebounder, snatching the ball near the rim in this clip; he rates slightly higher than Marc Gasol on offensive rebound rate as of last week, as strange as that sounds. If you want to see his jump shot, here’s an open one he took. For all his skills, he’ll need a reliable outside jump shot or, alas, he may have problems long-term holding down starting spots on good teams.
Mar 29, 2015; Washington, DC, USA; Washington Wizards guard John Wall (2) dribbles as Houston Rockets guard Nick Johnson (3) defends during the first half at Verizon Center. Mandatory Credit: Brad Mills-USA TODAY Sports
SportVU: Max Velocity
When SportVU’s data was first released to the public, we only saw the proverbial tip of the iceberg: basic pass information, catch-and-shoot and pull-up jump shots, rim protection, and, curiously, miles per hour, among other things. At first glance, having a distance or average speed measurement seems useful for basketball, but it appears to be frivolous, especially because you’re given average speed for entire games and it’s not split by offense and defense. We’ve always yearned for some speed measurement to objectively sort out the fastest guys in the league, but alas, this wasn’t it.
However, with the quiet release of spatial tracking data for every player and the ball for every single play this season, we actually have the resources to find max velocity or any other distance or velocity measurement you want. It just takes a bit of work and a lot of data.
Basically, the movement data are in steps of 0.04 seconds, and you’re given the X and Y coordinate of every player on the court. You’re also given the X, Y, and height coordinates of the ball. Then you have some time-stamps and other critical pieces of information, like time elapsed, the shot clock, and the event ID. This event ID is quite useful because if you go to a play-by-play log, you can click on a line and read what event it is and even watch every event. This is useful for verification purposes and to tie the SportVU data to other sources. Plus, as any NBA data analyst knows, even if you crunch numbers and write code for hours, you still want to watch the actual video and either troubleshooot or brainstorm. (Any analyst who doesn’t watch the video is going down the wrong path.)
To test out my method, I identified a game where two known speedsters, John Wall and Ty Lawson, played against each other. (I grabbed this data a while ago, or else I would have targeted a Westbrook game since he’s been tearing up the league the past couple months.) Then I calculated the velocity for each guy at every possible moment in one-second increments to weed out any unstable results — I’d trust sustained speed more anyhow. Remember how dense this data is; Wall’s dataframe has 50,400 lines all on its own. But with some simple coding, calculating his velocity at every point takes a split second, and that efficiency means I do some more interesting work in the future with more games or players at once.
For John Wall, he maxed out at 17.17 MPH (27.6 km/hr), and hit 17 MPH during another play later on as well. Here’s a link to the first play (note: the event ID is 105, but the camera doesn’t catch the whole play so I went one event earlier.) During a fast break, Wall has the ball and he drives strong to the basket and then shovels an assist off to Beal in the corner while jumping. If the shot clock is accurate, he hits 17 MPH somewhere around the three-point line. I’ve given a speed diagram below where you can see how quickly he peaks in max velocity. And this is when he has the ball.
Here’s the link to his other high speed play. On this possession, he’s just turned the ball over and rushes back on defense, priming himself for a chase-down block, but he’s a split second too slow, despite hitting 17 MPH.
Ty Lawson, however, loses this speed battle: he tops out at a mere 16.64 MPH (26.7 km/hr). He does so on this fast break play where he has the ball and he’s starting a fast break off a made basket. He beats Wall down the court, but Gortat is already waiting at the rim. You can see his speed profile below. Given how small the court is relatively speaking, these players have to accelerate quickly, and in just a couple seconds they can reach a speed where they blow past everyone.
In the future, perhaps I’ll have the time to grab more games, and ones that include players like Westbrook and LeBron. Basketball games are not track events, and there are hardly any good opportunities to run as fast as you can unimpeded, especially since you’ll run out of court quickly. It takes even guys like Usain Bolt three or four seconds to get up to speed. Also, it’s fascinating that Lawson and Wall hit their peaks with the ball; that’s something we don’t (yet) see at the 100 meter race at the Olympics. But we finally have some hard data on speed, and we got it from real NBA games, not a combine. The next question is, who’s the fastest player in the league?