Projecting the 2014 Rookie Class Forward
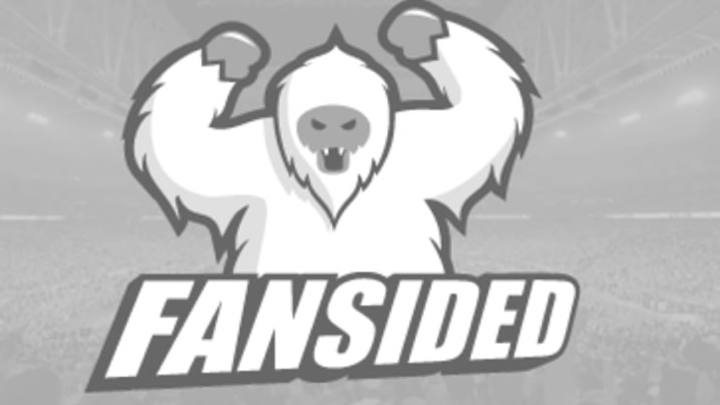
Peak Bogdanovic? Mandatory Credit: Adam Hunger-USA TODAY Sports
Few of the analytic models around are built to only project rookie performance. Likewise, teams and scouts don’t evaluate players simply on expected rookie production for the very good reasons that rookie players tend to be below average, even the better ones. Still, the shape of age curve and the NBA’s collective bargaining agreement conspire to make the later years of the rookie contract and the right to match any contract in restricted free agency the most valuable portions of a team’s draft right. In pursuit of extracting that value, the first year in the actual NBA inevitably provides a huge new data point to reevaluate each prospect playing against the highest level of competition. However, like any single year of data, it is wise not overreact.
Last year I built a model that combined the projections I had made in my draft model with the performance in the rookie year to get a sense of the relative weight to put on rookie performance and pre-draft data, as well as age and draft position. I made a few tweaks this year, then applied that to the 2014 rookie class that has just completed their rookie regular season[1. Both the draft model and the update used the player’s maximum AWS stabilized by regressing against a replacement level value weighted by minutes.].
The two major tweaks were to explicitly separate the age factor and pre-NBA production, something I had done in the testing phases last year and to construct a version using the total Alternate Win Score (AWS) production as the independent variable. Separating age and production doesn’t do anything to improve predictive power of the model, but it does give us the ability to weigh their relative contributions. The total production model[2. Conceptually the total production model is similar to total Win Shares or various wins above replacement models, multiplying AWS by minutes played.] gives us another measurement of player value that better indicates the total contribution by players and the gap between true stars and efficient role players.
In the training data, I found that for both the AWS Efficiency model and the AWS Production[3. Both models were regression models cross validated to select the most stable predictors] model combining the rookie data, age, and pre-NBA production was able to predict just under 60% of future production. For both models the most important predictor was rookie production. In the AWS Efficiency model that was followed by pre-NBA production then, closely behind, by age[4. Converted to a natural log]. Unsurprisingly, in the AWS Production model time played as a rookie was a much more significant factor as the second most powerful variable just ahead of pre-NBA production and age. A minor factor in both models was the player’s three-point shooting rate, indicating that perimeter players should see slightly more growth from the their rookie year controlling for pre-NBA production and age[5. Similar to findings by Layne Vashro showing height to be negatively associated with future improvement].
The 2014 Rookie Class
I applied both models to the recently completed seasons of the 2014 rookie class. A couple of interesting indications come out of the models such as which players to expect the most or least future improvement and which players damaged their stock the most.
Unfortunately, a number of rookies this year had their seasons cut short by injuries. In those cases, both models may under estimate the rookie’s true talent level, unless the injuries are severe enough to affect the future arc of their career. The AWS Efficiency model is likely to be the closer estimate in most injury cases due to the reduced influence from minutes played.
Below is the AWS Efficiency model for the 2014 rookies including the actual performance this year and the model’s projection for the end of rookie contract performance and the difference between the two. The average NBA player, as well as the average model prediction scores a 4.0 AWS per 40, while the average rookie performance is approximately 2.8[6. An All-Star would typically score around 8.0 and a star like Kevin Durant in his MVP year around 11.0].
The table above is sorted by rookie AWS efficiency regressed against a rookie replacement level from highest to lowest. The Bulls’ Nikola Mirotic is scored as the most efficient rookie, but the model projects him as the second most efficient player going forward to the Sixers’ Nerlens Noel, primarily because of the difference in age. The other notable players projected to decline in rank are L.A. Lakers rookies Jordan Clarkson and Tarik Black as well as Nets rookie and European import Bojan Bogdanovic who also shows the least expected improvement.
The biggest improvements are expected out of the players originally projected to perform the best by my draft model, a number of whom, outside of Noel, admittedly had disappointing first years, such as Jordan Adams and Clint Capela[7. Capela started the season with injuries then spent most of the year playing very well in the Development league and has looked decent in some spot playoff minutes, so I am still high on him.].
As mentioned above, in addition to the AWS Efficiency model I created a model to update the production projections giving greater weight to playing time. A couple of differences come out of this, role players like Furkan Aldemir who put up decent numbers in limited minutes after coming over from Turkey fall in the estimation of their rookie impact as well as expected rank going forward, while the projections for big minute rooks like Andrew Wiggins rise considerably, up to second in Wiggins’ case.
In the table below, I have the actual rookie AWS production as well as that projected by the model. I also highlighted a couple of the players with significant injuries. For those players, like Jabari Parker, for example, the relative appropriateness of this model will depend on any lingering career effects from their injuries. If one expects little or no long term effect, then the Efficiency model may give a better indication of the player’s future prospects.
The average production score for a rookie in this model is 3.6, which rises to 8.3 at the end of the rookie contract. Only Noel is projected at a star level, which would translate into approximately a 50% chance of being a star, while Wiggins, Mirotic, Elfrid Payton and Marcus Smart look very solid in this model.
These, of course, are merely projections and projecting prospects is an imperfect art to say the least, whether using statistical models, scouting or both. The more liberal use of the NBADL may change the historic relationship with rookie minutes and future production. Sometimes a young top draft pick with great numbers in college comes off a decent rookie year and proceeds to make a mockery of the age curve by continuing to decline for years, like say Michael Beasley. But the combination of rookie and pre-draft data makes the art of prediction a bit more accurate.