Replacement Level: Role vs Quality
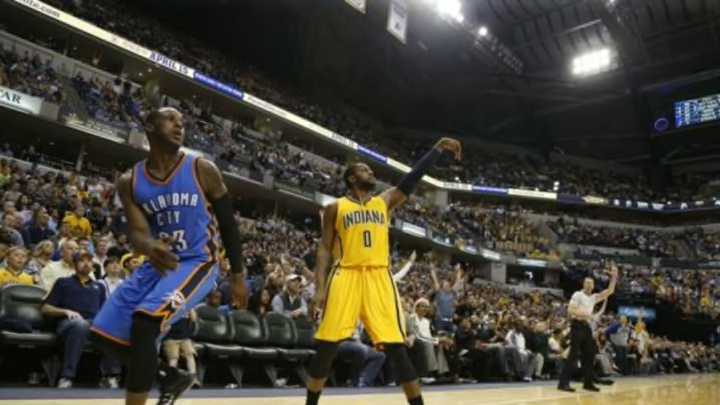
Note: Right before the author wrote this article, he stared at way to many numbers for way too long. Also, it’s 96 °F right now. Please expect a bit of weirdness.
When the Nylon Calculus gang (Nylon Gang ain’t nothing to math with) discussed replacement level recently, a few things went not optimally. The most blatant error we made (at least in my opinion), was to not more thoroughly check the literature beforehand. Otherwise, we probably would have included the work of Tangotiger, who also mentioned our writings shortly after we published it[1. Between Tangotiger and Pizza Cutter, baseball stats guys seem to have some kind of random name generator thing going on. Or I’m just not in on the joke]. The good old APBR-board had their own very noteworthy thoughts on it as well. As you can see, we reinvented the wheel a bit. With all this information in mind, I came up with the following thoughts and methods:
Replacement level is divided into role and quality
This is, in my opinion, a bit underrepresented in the previous work, where replacement level is more observed as a quality term, than a role term. Sure, players that are paid the minimum salary usually produce worse outcomes in metrics. But a lot of players that are central for their team do so as well, but in completely different roles. If Kyle Korver had to play the shooting guard position like James Harden, or of Tristan Thompson had to play power forward like LaMarcus Aldridge, they would surely have statistics too. If Carlos Boozer’s job on the Lakers would have been to carve up secondary units (maybe alongside a rim protector instead of Robert Sacre), he might be a plus player. So, while I understand the train of thought in terms of quality, for me the question went further: Which are typical roles for replacement type players?
The NBA as a Random Forest
To get an idea about roles and different player types, I did the following:
- Get as many ‘minute-less’ stats as possible. With minute-less I mean stats that are either per 36 minutes, or percentages (e.g. shooting percentages or turnover percentages). In my case, I picked the tracking data from NBA.com and the per 36 data and advanced stats players tables from Basketball-Reference.
- Sort Players into three groups by minutes per game. I only used players that played at least 10 games. Players that played between 0 and 16 minutes per game were classified as ‘Replacement Level’, between 16 and 26 minutes as ‘Role Players’ and more than 26 minutes as ‘Starters’. This was based on Tangotiger’s idea and an easy way to label players. Of course this is far from perfect, as we transform a continuous function into a discrete – but it’s a start.
- Throw the whole thing into a Random Forest. Short reasoning: Random Forests are great for throwing the kitchen sink. I have no exact idea what will be the output, but I can expect that the Random Forest finds a relatively close to optimal solution without risking to much overfitting thanks to cross-validation (Note: I’m always interested in better algorithm)
Results 1: Classification
As a Random Forest has some randomness (duh), results tend to slightly vary between repeats. In general, the Random Forest spits out:
- A predicted class probability for each class and each player. For example, the five players with the highest probability to be a starter (>95%) are Chris Bosh, Gordon Hayward, LeBron James, Marc Gasol and Rudy Gay.
- The classification works well, given that I try to put labels onto a very fuzzy setup. For example, of the 149 players with starter minutes, only three are robustly classified as ‘Replacement Level’: Dion Waiters (who has a lot of similarities to Eric Green and Jimmer Fredette), Elfrid Payton (who is similar to Nick Calathes and Ish Smith) and Tristan Thompson. Thompson is of course a very interesting case, due to his new contract. I guess he is somewhere between DeAndre Jordan and Tyson Chandler on the one side and Greg Stiemsma and Chuck Hayes on the other side. OF COURSE, this is in a lot of regards more a question of style than quality. Kyle Korver for example has as well an around 30% probability to be replacement level, as some of his most similar players are James Jones and Stan Novak.
Results 2: Classifying variables
You probably where curious what I previously meant with similar. What Random Forests tell you, as well, is which statistics are the most useful for separating your groups, something that is probably even more interesting than the classifying because somehow that answers the question: What are deciding classifiers for playing time? The answer:
- Win Shares and BPM: Unsurprisingly, Win Shares per 48 and Box Score Plus Minus are the best classifiers. Just using those three alone gives us a kappa of around 0.35, which is not so far off of the 0.43 kappa of the complete set of 73 statistics. The difference between offensive box score plus minus and defensive box score plus minus is huge. While OBPM alone has a kappa of 0.26, DBPM’s kappa is 0.11 – which is close to flipping a coin. Furthermore, I don’t find rim protection stats, nor blocks, steals or rebounds to be very telling about a players minutes. This probably means a mix of two things:
- We are still not very good at evaluating defense
- Teams have a much higher focus on the offensive output of a player
- Hustle Plays: The best separating statistic is a bit of a cheat – it is fouls per 36 minutes. Of course I commit more fouls if I know that I have 6 of them to give and only 12 minutes playing time. Replacement level player commit 4 fouls per 36 minutes, around 1.4 fouls more than Starters. A similar thing is average speed. I can run much faster for 12 minutes than for 36 (duh). The average speed for starters is 4.07 mph, for replacement players 4.24 mph. The last difference is offensive rebounds, with uncontested offensive rebounds being the most informative one. I would guess that you forget more often about Festus Ezeli than about LeBron James. But they also get more contested rebounds, which shows that offensive rebounding might be a skill that is not typical for a max salary.
- The license to dribble: On the other hand, it is not very surprising that not everybody has the same right to put the ball on the ground. In short, everything from pull up attempts, over dribble drives to usage and time of possession is in the hands of the Starters. And of course Points, where Starters score 16.6 and Replacement players 11.6 per 36. (Note: In comparison, the difference in steals is 1.72 for starters and 1.57 for Replacement.) The difference in percentages (example: TS% for Starters is 54.2%, for Replacement 49.6%) is as well a good indicator
- Daddy eats first: This one does not help so much for classifying, but is more of an interesting tidbit. Both Starters and Replacement Level Players get 1.11 contested rebounds per 36 minutes. BUT Starters get on average 3.81, Replacement Level Players 3.44 uncontested rebounds per 36. I cannot imagine a nicer symbol for pecking order 🙂
Conclusion – Not much conclusion but it’s a start
That’s it for now. I see the whole thing as a work in progress and hope that it explained the difference between the more literal role of a Replacement player (or any player) and the more abstract metrical use of Replacement player. Underneath you find one of my famous cluster heatmaps. The numbers in circles underneath are according to Results 2. I only show players that played at least 42 games – and I guess it is still way too much. But maybe you can at least enjoy the Cluster Group names. I think I’ll take a shower…