A Random Walk Through NBA Variance
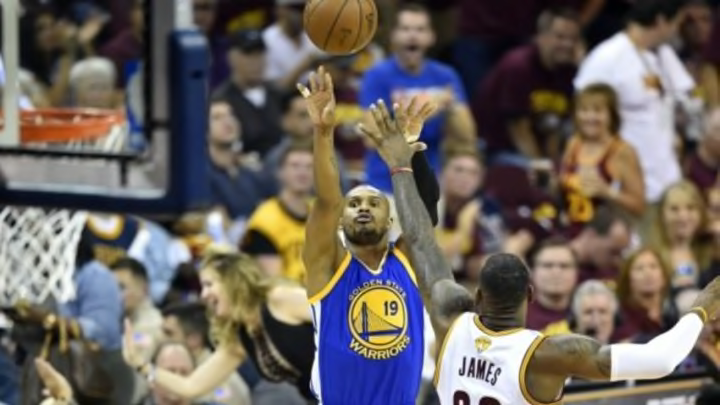
The hope of many, is that the Golden State Warriors championship will finally bury the phrase “Live by the three, die by the three.” Although it contains a positive and a negative, the usual translation of this phrase is, “three-point shooting teams will lose at some point.” However, a more accurate translation would be, “three-point shooting teams have a higher variance in their game outcomes.” And this is often found to be true. There are implications to high variance strategies. In a nutshell, if you are the better team, you want to avoid variance, as it gives your opponents a higher chance to win. And if you are the underdog, you want to have the variance as high as possible, so that you have a ‘puncher’s chance’. This is very nicely described in this blog post.
The Random Walk Model
Being interested in taking a closer look into all things that might influence variance, I came up with a random walk model to simulate a basketball possession. Thanks to the omnipotent Basketball-Reference, I had information about every teams shot distribution, shot percentages and the amount of turnovers, free throws and offensive rebound percentage for each team during the last two seasons. Thanks to the similarly potent Darryl Blackport, I knew for each team the possessions per quarter and the points per quarter. To now simulate one quarter for a team, I could randomly draw the number of possessions per quarter and then simulate each possession using a random walk. Here is a slightly simplified scheme of this random walk:
The dice symbols are a simplification of a random event, where different parts of the event are weighted differently for different teams. If the event is a turnover, the possession is over. A possession is as well over if a shot or the second free throw is made. If a shot or the second free throw is missed, the team has the chance for an offensive rebound and the same game can start again. That’s it (more or less).
All I had to do was to let my computer simulate 500 seasons for each team and calculate mean points per possession and the variance or standard deviation.
Does This Simulation Work?
I’m glad you asked! There are two parts to answer this question: First, we need to show, that our random walk is good at simulating the mean expected points. Comparing the Basketball-Reference net ratings with our estimate, we see:
It’s off, but it works! The correlation between a season and the actual Basketball Reference ratings is close to perfect. That it is off by around two or three points might be easily explained. My model does not include And-1’s, technical fouls or three free throw attempts, all of which increase the number of points per possession.
The
more interesting question
is: Gives the random walk a good estimation for the variance of a season? And the answer is: The estimation is as good as possible.
The reason I say “as good as possible” is that our real variance is calculated during the time span of one season. If I use my random walk to only simulate one season and compare this simulated season with the 500 seasons, I can get very different results:
On average, I only get an r-squared of around 0.2, which shows how variable variance can be during an 82-game span. So my resulting r-squared of 0.25 are actually better than expected. (Note: This is a euphemism for ‘I guess I’ll never be able to test how accurate my model actually is, because there is way too much noise…’)
Results
This is even more true for the simulated data, for which 50 percent of the variance can be explained by three-point field goal attempts (I hope I did my math right…). Three-point field goal percentage is another important factor for variance (at least for the simulation), which also explains why Philadelphia has such a low variance.
Your three-pointers simply do not create variance if you miss almost all of them…
Before “How’s it goink?” Gets a Second Wind
Of course variance is only secondary. First, your team must score more points on average, before even considering to minimize their variance. And there I have bad news for Phil Jackson. Apart from field goal percentages, three-point frequency is the best predictor for offensive rating, better than turnover frequency or free throw frequency:
Close range frequency for example does not tell you anything about offensive rating, so you might reconsider forcing it to your center;
So yes, you might die by the three, but good luck trying to live without it.
Epilogue
A lot of things remained untouched (Note: Now I sound like a Game of Thrones Epilogue…). The problem with the whole variance thing is that all the values can be correlated. Teams with more three-point attempts might have less offensive rebounds. So I’ll move a lot of the murkier information into the eventual next chapter (Note: I hope I write faster than George R.R. Martin…). Trying to think about all things that could influence variance, I came up with the following:
The obvious influences
Three point shooting: Imagine you shoot 100 threes and are a 33% shooter, or you shoot 100 two’s and you are a 50% shooter (not a realistic outcome but bear with me). Now, your 95% confidence interval for points outcome would be between 72 and 123 points for three’s and 80 and 118 points for two’s. This leads to higher variance for threes.
Pace: A higher pace means that you have more possessions. More possessions mean that you are more likely to regress to the mean. Interestingly, both pace and three point shooting were things that were advantageous for the Cavs during the last Finals, as both teams shot a lot of threes and the Cavs tried to slow the game down as much as possible.
The not so obvious influences
Shooting 50%: Player A shoots 80% on free throws, Player B shoots 20% on free throws and Player C shoots 50% on free throws. Around 95% of the time, Player A will make between 72 and 88 of 100 shots, Player B between 12 and 28 and Player C between 40 and 59. The difference is not enormous, but you simply have more wiggle room if you shoot 50%. A 0% shooter and a 100% shooter have no variance. Interestingly, this tends to even out with…
Shooting inequality: If a team takes 50% of it’s shot from midrange (40% shooting percentage) and 50% of it’s shots from close range (60% shooting percentage), they’ll end up taking more midrange shots on one day and more close range shots on the other day. So, if a Team of a 90% and a 10% free throw shooter would play against a team of two 50% free throw shooter, they would have the same expected mean and variance. I haven’t done Bayesian statistics in a while.
Free throws: Free throws itself are the anti-three pointer. You basically double the amount of possessions and therefore reduce the variance of the free throw. The problem of course is that free throws usually yield more points per possession than an actual shot attempt. So, the aforementioned shot inequality can come in place.
Turnover and offensive rebound percentages: Intuitively, you would assume that more offensive rebounds or more turnovers lead to a higher variance. But it really might depend on how much you actually score. If you don’t score that much in the first place, turnovers might not have a big influence on your variance. And if you score more than one point per possession, offensive rebounds might be helpful during those days where you have a cold streak.
I’ll try to untangle all these effects – the next time…