Introducing KOBE: A measure of shot quality
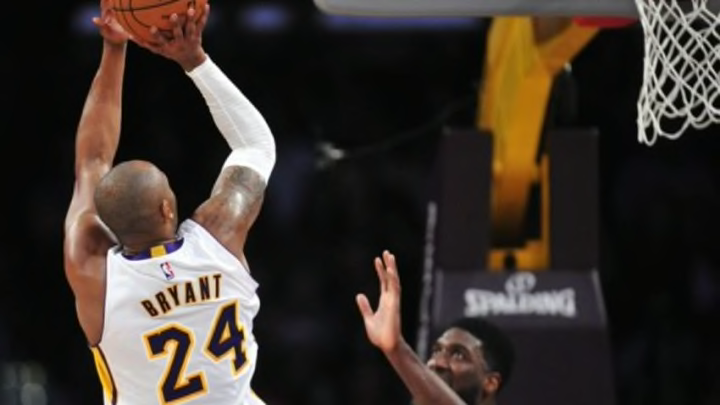
During the 2014/15 season, I introduced my Shot Difficulty metric and then aXPPS, which builds off the Shot Difficulty metric. And so now I present to you KOBE (Krishna’s Objective Basket Estimator)[1. What kind of person puts their own name in a metric…? Well unfortunately, there’s not a lot of words that begin with K that would make any sense and it’s absolutely vital the metric be called KOBE so I’m going to be that guy who puts his own name in his metric][2. Ed. Hockey guys are wondering what’s the big deal? Corsi this, Fenwick that…], which is admittedly just a reworked and improved version of aXPPS but with one notable change: the name![2. Cmon, it’s a much better name right?]
KOBE is split into two models, one for close shots and one for longer shots. For some of the nitty gritty details, please see the previous posts.[3. Regarding the changes, I added an interaction term for the long shots model (shot distance*dribble type), changed ln(dribbles+1) to a dummy variable for catch and shoot vs. off dribble and removed shot distance squared and cubed (coefficients were so small that it didn’t seem to be adding anything). Those may seem like a lot of changes but none of the players really changed much, nor did it alter the “difficulty rating” of the vast majority of shots all that much.In terms of material improvements to the model, the interaction term seems to do a better job modeling shots 5-10 feet now. For the close shots model, I removed the game time variable which made no sense in the context of the coefficient and added a dummy variable for open shots in order to correct for the ease of dunks and open layups being underestimated in the original model. The overall cross-validated accuracy% was around 62-64% for both models.]
However, I would like to go into how the metric performs though. I think it’s important to know the strengths and weaknesses of a metric and I do not want to blindly share KOBE without detailing how it performs. Here is the accuracy% of the metric,[4. To determine the accuracy%, if KOBE was over 0.5, it was given a value of 1 (or a made shot) and if it was under, it was given the value of 0, which is a missed shot. The reason for this is if a shot has a KOBE of 0.52 for example, it is more likely to go in then it is to be missed.] with 2015 being the year the metric was trained on and 2014 being out-of-sample:
2015 | 2014 | |
Long shots model | 0.6151 | 0.6098 |
Close shots model | 0.6360 | 0.6349 |
The accuracy isn’t the greatest but I also think that using this method of looking at the overall percentages underestimates the actual strength of the model.[Ed. Also, we’re probably picking up some of the inherent randomness of shots going in or not, regardless of difficulty, in a Make or Miss league.] So I also decided to bin the data and look at the accuracy across different shot and defender distances[5. Obviously there are many different ways I could bin the data but I decided to use similar bins to the ones NBA.com uses on their site.]. Here were the results[6. Again, 2015 is the year the model was trained on with 2014 being out of sample.]:
2015:
2014:
We can see some clear strengths and weaknesses of the model but on the whole, I think it does a good job. Also, when looking at the results, keep in mind some of the small sample sizes for the bins.[7. For example, in the 5-10 foot shot distance range with the defender 10+ feet away, the total FGAs is less than 100 for both years and so the 20%+ error is a bit deceiving given that small sample size.] The main weakness in the model seems to be the ability to estimate wide open shots in the 5-10 foot range and the ability to estimate wide open shots near the basket. The later probably end up being mostly dunks and while the adjustment I made does help, it still underestimates shots in that 10+ foot defender distance range by a little over 5%.[8. In the previous iteration of the model, it was underestimating those shots by 10+%.]
Finally, before presenting the players’ KOBE, I wanted to look at a strength and real innovation of the model: the incorporation height difference. This is particularly important when looking at shots near the basket:
Height | FG% | KOBE | FGA |
-10+ | 0.5307 | 0.5167 | 1613 |
-8:-9 | 0.5392 | 0.5355 | 2385 |
-6:-7 | 0.5549 | 0.5555 | 3478 |
-4:-5 | 0.5806 | 0.5848 | 5119 |
-2:-3 | 0.6025 | 0.6040 | 8656 |
-1:1 | 0.6272 | 0.6277 | 17113 |
2:3 | 0.6541 | 0.6562 | 7376 |
4:5 | 0.6887 | 0.6871 | 3691 |
6:7 | 0.7166 | 0.7104 | 1955 |
8:9 | 0.7469 | 0.7342 | 1126 |
10+ | 0.7444 | 0.7500 | 626 |
As we see here, there’s nearly a 20% difference in FG% when the shooter is 8+ inches shorter than the defender versus 8+ inches taller.
I’ll end the post with the KOBE for each player, broken down by team and total for players who changed teams in 2014/15. These will be added to the stats section and updated throughout the season. The lower a player’s KOBE rating, the harder his mix of field goal attempts:
You might notice I’ve added two columns capturing a metric I’ve[1. Cleverly…] named SHAC, or “SHot Adjusted Capability,” which is simply the difference between the player’s expected points per shot as measured by KOBE and their actual points per shot for the season. SHAC has been included on both a per shot and total basis. Klay Thompson! The chart below visualizes the KOBE and SHAQ for individual players in 2014/15
Some final observations and notes on KOBE:
- Chris Paul is among one of the worst players in KOBE. This may seem odd to some people because he’s one of the five or so, best players in the NBA but think about his shot selection. There’s a lot of midrange shots and there’s a lot of contested midrange shss ots. He just happens to be a magician at pull up jumpers from the elbows.
- Also per Nathan, “Easiest” miss of the year was this Dion Waiters 2-footer (which he immediately rebounded and made).