2016 Usage-adjusted Win Projections, Part 1
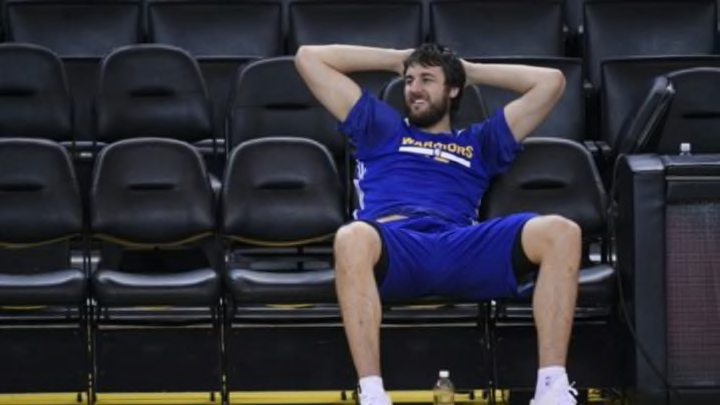
Win Projections are my speciality — not because I’m especially good at them[1. Though I have done extremely well the last two years thanks to Real Plus-Minus] but because I think they’re especially fun[2. Puts on nerdy glasses]. If you’re familiar with my style of win projections, you know that I am a big fan of Real-Plus Minus for its out of sample prediction ability. So without any further ado, here’s how I projected win totals this year.
Step 1: Player Ratings: Blend RPM and BPM
First, a quick overview of why RPM and BPM can be used to predict wins:
RPM’s strengths:
-Most accurate prediction ability of future possessions for any publicly available all-in-one statistic.
That seems like a good summary.
BPM’s strengths
–Still very good out of sample prediction[3. About the link — BPM used to be called ASPM]
-Passes the eye test a little more strongly[4. Somewhat because people use per-game info to make judgments, and BPM really only includes “visible” things like scoring, assists, etc].
-Much more data on player progression and regression (36 years’ worth).
-On par with RPM in terms of predicting offense
The last two points are the salient ones here. While RPM is the heralded champion at predicting future possessions and wins, BPM has way more seasons under its belt to help us predict player development/regression. So – my assumption is that BPM has some information to give us regarding offensive development that RPM might not.
The weights are relatively simple: Box Plus-Minus gets between 0 and 25% weight, based on how much of a player’s RPM rating last year came from their offense. For a Russell Westbrook type, BPM’s projection is weighted higher (22.5%) say than an Andrew Bogut (12.4%)[5. Notice that despite Bogut’s low RPM rating on defense, he still gets a share much higher than zero. That’s because his offense is particularly negative according to RPM – so I assume there’s significant information there to not be ignored] or a Dwight Howard (9.4%).
Translation: I blend RPM and BPM ratings, RPM for its known predictive ability, and BPM for its larger dataset of player regression.
Note: For rookies, I used Kevin Ferrigan‘s RAPM projections.
Step 2: Adjust player ratings for usage
This is the big one. After a whole summer of not being able to come up with good “fit” adjustments, I was able to at last built some semblance of a “Usage” adjuster. This is what my analysis found:
- The BPM of players on a team with too many high-usage players typically regress
- They especially regress when their value is scoring-based (i.e. Russell Westbrook would regress more than Bogut in a scenario where they gain lots of high-usage teammates)
And here’s how it impacted my projections[6. The reason it isn’t perfectly linear is because of #2 — individual players regress at different rates based on their prior-season scoring.].
It may seem extremely counter intuitive, but what I found from 36 years of player-season data is that offensive players regress significantly from their projections when newly paired with other offensive players. Think 2011 DWade/Bosh, 2015 Kyrie & KLove. OK so this makes sense – players will score less if they have to share the ball. I also found the reverse to be true – players newly paired with low-usage guys tend to fill the gap more (i.e. Russell Westbrook without KD last year used I think 14,000% of possessions).
Unfortunately, while my analysis found quite a lot of “signal” — that is, team usage variables that were almost certainly impacting player BPM, there was also a significant amount of noise. I therefore added in some extra regression to prevent my results from being too strange[7. I multiplied the initial adjustment by about 1/3.].
Translation: While high-usage guys provide extra value on their own, regression[8. or diminishing returns] occurs when you team them up *AND* vice versa. So I adjust accordingly.
Step 3: Project Minutes
I spent most of my time last season working on minutes projections. Minutes are extremely important as they can completely skew your results — for example, if you have Clint Capela playing 990 minutes per game, the Rockets might not be a top-5 team[9. ;)]. Rather than spend all my time working on this, I “outsourced” by using the venerable Kevin Pelton’s minutes projections, and combined them with a fantasy sports site’s projections. After averaging, I multiplied each player’s minutes by a constant, forcing the team’s total to equal the magic number, 19,680 minutes.
Step 4: Project pace
WARNING: This method is lazy.
First, I created a “True Pace Estimate,” for 2015. This assumes each team played opponents with average pace last season, and removes that “average team” from their value[10. True Pace Estimate = 2xPace – League Avg Pace]. Then, I regress each team to the mean based on how much roster turnover they had to come up with a 2016 True Pace Estimate[11. 2016 True Pace Estimate = (Expected Roster Minutes Continuity% x 2015 True Pace Estimate) + (1- Expected Roster Minutes Continuity)x2015 ]. Finally, I project each game’s pace by taking the average of both team’s 2016 True Pace Estimates.
This is important because higher pace = lower variance. Better teams win more often in these scenarios and vice versa.
Translation: Good fast teams win more games than good slow teams, so I adjust accordingly.
Step 5: Adjust for Variance and Project Win probabilities for each game
The first four steps give us enough information to project a team’s chance of winning.
Team A Win% ~
(Team A Rating – Team B Rating) * 0.5 * (Team A True Pace + Team B True Pace) / 100 + Home-Court-Advantage
But there is one mystery yet left to solve, which I eyeballed this year: how much should I trust my ratings?
If we take my player ratings, minutes projections, pace at face value, we can calculate win probability as usual — but these team ratings are different than mid-season numbers.
One of the biggest reasons I didn’t win the APBR contest last year[8. Though in terms of Average Error, I actually did win :)] was because my data wasn’t regressed enough towards the mean. Simply said, you can’t use the Pythagorean formula (or in my case, the normal distribution function) as-is to project out-of-sample wins. These win percentage formulas are based on in-sample Efficiency Differential, which is much more highly correlated to win percentage than this preseason data.
So I took my win projection totals and added in a variance factor — increasing the formula’s[9. In excel, the formula is =NORMDIST(Expected Point Differential, 0, Expected Variance (Standard Deviation), 1)] variance by 14 percent[9. Exactly half of the 28% variance increase which I found most closely matched my win totals to Vegas’ predictions].
Note: This is the biggest change I made since we put out the great Nylon team previews (other than adjusting for Tyreke Evans’ injury today). Sorry! Nobody changed more than a couple wins.
Translation: We are less able to predict a whole season’s worth of wins before it starts than we are able to predict a win three-fourths of the way through the season, so I adjust accordingly.
That’s enough words for now. *Here’s* part 2 of 2 — The Results!