What the Hornets Preseason Record (7-1) Means
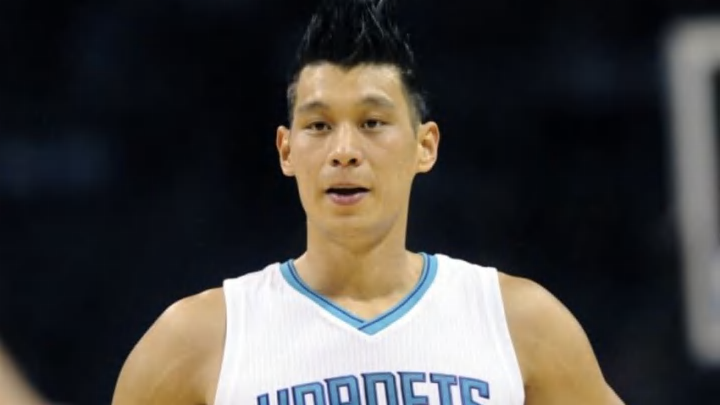
Coming off of a disappointing year the Charlotte Hornets have stormed through the preseason for the 2015-2016 campaign, shrugging off injuries, launching threes like they owe Darryl Morey money and posting a 7 and 1 loss record. Leaving Hornets fans, like die hard fans watching preseason games everywhere, trying to take that information in to project their team into the regular season. I do the same thing, I mean I am watching the games and it’s something the resembles basketball with players that generally resemble NBA players, but, of course, the games don’t count. So what does the preseason mean?
The More You Know
Statistically, basically, the more you know the less it means.[1. This isn’t entirely unique to preseason, for much data elements there are diminishing returns, especially when that data is correlated. For example, forensics can predict height with a high degree of accuracy from just someone’s tibia bone. But adding the femur, a longer bone, adds fairly little to the accuracy. There are, however, other cases where two variables strengthen each other, so this is not a universal rule.] Last year I took a look at that comparing teams preseason record over the prior seven years and their record in the regular season and found that indeed there is a consistent modest correlation from preseason record to the record in the regular season.[2. Regression formula being .379+ .239 * Preseason Win Pct]
So if all we knew about a team was their gaudy 87.5% winning preseason record using the formula from the trained model we would estimate that they were a 48 win team, or a 59% winning record. We could also use a Bayes theorem to update our beliefs by weighting based on the expect amount of variation in NBA regular season records and the higher variation in preseason records, which would give us an expected win total of 49 or 50 games.
But we live in the age of Google, so one can at least look the Hornets’ record last year, which was 33 wins and 49 losses. In the same study last year I combined the record the prior year and preseason record to predict the following regular season record. I found that the preseason winning percentage still has a predictive power for regular season. However, the effect of the coefficient was 25% less powerful than when we had no other information.[3. Formula: .145 + .18 * Preseason Win Pct + .52 * Prior Season Pct] In this case the trained model predicts the Hornets will have a 51% win percentage and the Bayesian formula with reduced variance given the prior season record predicts a 42 win season.
We can do better than that though. The prior season record does not include information about player movement, injuries or aging, It is no wonder the temporarily LeBron-less Cavaliers of 2010-2011 are the biggest outlier from the model. Methods using a combination of box score type data and regularized adjusted plus minus data (RAPM) have been producing better win projections than the prior regular season records consistently for a few years. The model I use based on my Player Tracking Plus Minus and RAPM performed well last year, and it is comfortably in that box score/RAPM family. Using that estimate produces a better starting point, but there is not enough of a track record to train a new model. However, we can use two methods to combine the preseason information and model projection, producing what should be a better estimate; weighted model averaging and the Bayesian update that I used before.
Using simply the amount of variation explained by each model I estimated that a reasonable weight would be 87% analytic model and 13% preseason record and I used Bayes theorem with the analytic model as my prior weighted by the estimated residual error. My analytic model estimated the Hornets with a 46% winning percent, or, a 38 win team after their young defensive star Michael Kidd-Gilchrist went down with a shoulder injury, likely missing the season. Applying the weighted average the estimated wins after a 7 and 1 preseason is raised to 43 wins and the Bayes estimate is at 42 wins.
At this point we could apply a consensus of analytic models. But, as I mentioned above analytic models using some combination of box score, play-by-play or SportVU stats and RAPM estimates have performed well recently. As these models have become more common, many if not most popular public projects become somewhat related. So, averaging and weighting models that are not independent might give us an overconfident estimate of predictive power. But, there’s more we can apply to build our prior estimate, in this case I chose to use the ESPN summer forecast, a forced choice subjective ranking system released in the NBA summer doldrums, and prior to when most analytic models are made public.
The Summer Forecast has done well in the last to years predicting wins and losses, though not as well as most of the hybrid analytic models. Based on that record for the weighted estimate I used the following weights, analytic model at 52%, Summer Forecast at 41% and preseason a mere 7%. In the Bayes theorem estimate, because the analytic model and the Summer Forecast can be considered independent, I can estimate a reduced standard deviation for my new combined estimate before adding the information from the preseason record. The ESPN summer forecasters were less impressed by the Hornets than my analytic model, projecting them to be a 35 win team for the upcoming season, giving a combined win estimate of 37 wins before adding the preseason. The final result using either the weighting method or Bayes theorem is an estimate of 40 wins and 42 losses.
This table tallies up all of the estimates above, where the 7 and 1 preseason estimate falls from 48 regular season wins to 40 as we look at other sources of information:
The falling total team win estimate is a product of the general disconnect between the prior information we have about how good the Hornets should be this year. Using the rough 1 and 6 preseason the Cleveland Cavaliers had this year, for example, we would get the opposite direction in projection movement from a 34 win estimate with only the preseason record to a 54 win season with the analytic model and Summer Forecast information included. The fall in weight given to preseason would, however, be similar. All in all, it is better to have a good preseason than a bad one, but it is not something fans should get too excited about.