2016 Neural Network Win Projections
By Nick Restifo
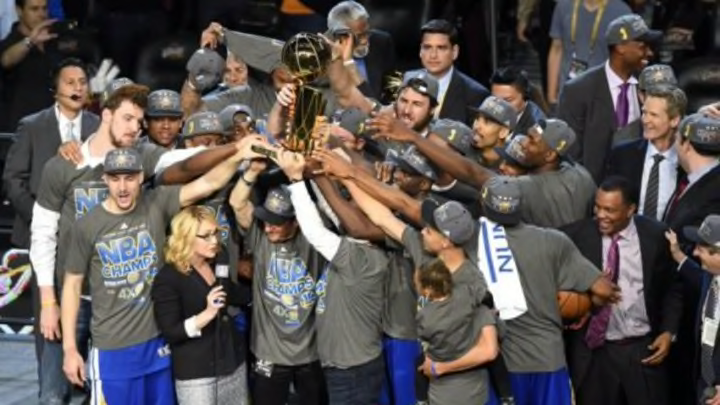
In order to arrive at a reasonable (and hopefully accurate) projection of the 2015-16 NBA season, many factors must be considered. Player value and playing time are among the most important considerations, but strength of schedule, travel, rest, and relative home court advantage are very real, significant effects and must also be included in projections. My win projections are an incorporation of all of this information.
My fellow Nylon-ers Justin Willard, Andrew Johnson, Nathan Walker, and Kevin Ferrigan have also worked hard to create well thought-out win projection systems for this coming season (Tuesday!), and I highly recommend you read up on their projections too. No one projection system we have here at Nylon is the same, and each of them have their own strengths (and weaknesses).
In the win projection system I am sharing here, I use several neural networks and regression models to predict a player’s projected share of minutes, as well as his RAPM for the upcoming season. The RAPM statistic I use throughout my system is a RAPM of Jeremias Engelmann, a prior-informed model that he posted here on the APBR forums. Within the models I use to project player minute share and RAPM, statistics and biographical information such as previous year’s minutes per game and log transformation of draft pick are used. Separate models were built and used to project these values for rookies.
Once a projected minute share for each player on a current roster is created, weighted sums of a team’s production can be built. Using the projected minutes share as the weight, I create weighted sums of several different statistics:
Projected RAPM
Previous Year RAPM
Previous Year WS
Previous Year BPM
Two Years Previous RAPM
Two Years Previous WS
Two Years Previous BPM
These statistics, along with game information, are what the game-predicting models use to predict the outcome of games.
The game-predicting models utilize logistic regression, neural networks, and classification trees to make their predictions. They were trained on all NBA games from the 2002 through the 2015 season and also consider factors such as rest and altitude.
Using those models to predict wins and losses of each game, I then simulate the season 10,000 times in order to get confidence intervals and to see the range of results possible for every team. I choose the iteration that has the best combination of minimizing distance from each team’s mean projected wins over that 10,000 record sample as the “best” result.
So without much further ado, here are my win projections.
Team | Wins |
GSW2016 | 60 |
LAC2016 | 59 |
SAS2016 | 59 |
HOU2016 | 57 |
CLE2016 | 56 |
MEM2016 | 52 |
TOR2016 | 52 |
OKC2016 | 50 |
CHI2016 | 49 |
ATL2016 | 48 |
BOS2016 | 46 |
NOP2016 | 46 |
UTA2016 | 43 |
WAS2016 | 42 |
SAC2016 | 41 |
DAL2016 | 39 |
PHO2016 | 39 |
MIA2016 | 38 |
POR2016 | 38 |
IND2016 | 36 |
MIL2016 | 34 |
DEN2016 | 31 |
NYK2016 | 31 |
DET2016 | 30 |
ORL2016 | 29 |
BRK2016 | 28 |
CHO2016 | 26 |
LAL2016 | 26 |
PHI2016 | 25 |
MIN2016 | 20 |
This is only one way of looking at the problem, and time will tell how accurate this method is.