Freelance Friday: Measuring Assist Quality
By Guest Post
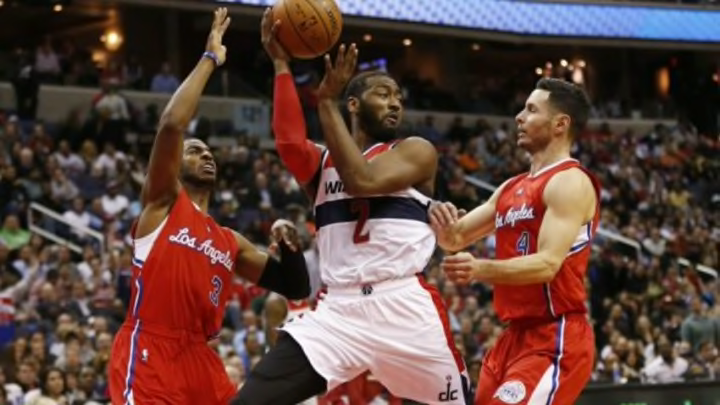
Freelance Friday is a semi-regular series at Nylon Calculus where we run submissions from readers in an effort to further democratize the field of basketball analytics. This week’s entry comes from Alex Lee, describing his work using SportVU data to come up with a better measure of passing ability and assist quality. Alex is a native of Canandaigua, New York. His passion, aside from basketball, is ultimate frisbee and he currently writes at the blog https://upcall.wordpress.com/. He can be followed on twitter at @_at_lee. We welcome submissions via email at TheNylonCalculus at gmail dot com.
Try to think of all the way passes are described: “beautiful cross-court bounce pass”, “pocket pass through two defenders”, “long-range lob”. We currently have very qualitative descriptions for passes. However, in making quantitative judgments about passing, we are starting from close to zero. With the SportVU tracking data, we can do much better. In terms of statistics on the offensive side, our understanding has become much more detailed when it comes to shot type, distance, defender distance. Similar measures of passing and the factors that distinguish between good and bad passers and passes have not kept pace.
At present, the main passing metric is the assist. To be frank, it is a terribly blunt statistic and feels anachronistic compared to all of the new metrics coming into more common use today. The first issue is that it is a subjective judgment that is made by a human statistician which can be both inconsistent and biased towards home-court players. Even if all of the judgments were perfectly uniform across all statisticians, it is still a binary metric for something that is so much more complex, a bad pass can lead to a made shot, while a perfect pass might be spoiled by a missed layup, neither outcome truly reflecting the ability or contribution of the passer.
To dig a little deeper, I chose to look at the physical characteristics of the pass: distance, time, speed and congestion (more details below), then ran a logistic regression, with parameters concerning the shot, defense and the new passing variables. From this regression, we can look at the impact that the passing variables have and create two new metrics, Assist Quality and Assist Points.
Using the “Movement” data found on NBA.com, I looked at the shots taken by the Clippers in the 2014-2015 season. Using the included coordinate data, it was relatively easy to capture some important measurements: pass time, distance, speed. However, these characteristics alone do an inadequate job of describing a pass.
Exceptional passers have great vision and accuracy. While it is impossible to know exactly where a player is looking, we know that part of having great vision is seeing openings in defenses, and having the precision to pass through tight windows. This is where another new metric, which I’ve termed “path congestion”, comes into play.
Going back to the movement data, a hypothetical line was placed between the current position of the ball and the shooter, a projected path. Then the distances between the defender points and this line were recorded.
The theta term is just to determine whether a defender is actually between the ball and the eventual shooter. The gamma term is quadratic depreciating effect was implemented for the distance because we believe that a defender’s effect decreases quickly as distance increases. If the defender is greater than 15 feet away from the path, his congestion contribution is set to 0. Path congestion is a unit-less metric and meaningless out of context, but gives us a relative scale to compare how crowded the pass’ path is, and how difficult it was for the passer to see the passing lane.
Below is a snapshot from a shot taken during the last regular season game between the Los Angeles Clippers and Phoenix Suns. The play is a made Blake Griffin(32) midrange shot assisted by Chris Paul(3) and the image is the mid-pass tracking data.
The SportVU tracking system records new coordinates every .04 seconds. So once we have calculated the range of time that the pass takes place, iterate over each frame, calculate the path congestion for that specific set of coordinates, and average the total congestion over the length of the pass. This leaves us with a value between 0 and 1 for each pass: 0 if there were absolutely no defenders contesting the pass, and 1 if all five defenders were standing along the pass path.
Intuitively, the value of a pass should decrease the greater the touch time of the shooter, so we separated our training data into separate bins of touch times: [0-2], [2-4], [4-6], [6-8], [8-10], [10+]. We utilized a logistic regression for each of the separate touch-time bins. We made a selection among the above factors and used the Bayesian Information Criterion to make our final selections for each individual bin.
Passing parameters were only statistically significant for touch times of between 0 and 2 seconds. This makes empirical sense – the longer a shooter holds the ball, the more the shot creation is dependent upon the shooter’s ability as opposed to the passer’s. Further for shots with 0-2 seconds of touch time, the model saw a sizeable increase in accuracy over a model that just considered shooter and defender information.
For each shot we can look at the value of the combination of all the passing terms. We call the combined value of these terms Assist Quality (AQ). Furthermore, we can multiply this value by the type of made shot, accounting for the added value of a 3pt assist. We call this metric Assist Points (AP). Looking at the top five passers from last year’s Clipper squad gives us the following list:
One would anticipate that a lot of offense is generated through Paul and Griffin’s passing, but Paul’s total stats are still staggering. He accounts for more assist points than the other four combined.
Looking at average assist quality, we see that Matt Barnes seems to slightly separate from the rest of the group. This matches what we empirically see during the game: Barnes is an underrated, excellent passer who can whip the ball across the court. His assist quality is also boosted by some of his longer, faster inbound passes.
Finally when looking at assist points, one possible explanation for Griffin and Barnes’s higher average assist points is that they pass to 3pt shooters more often. Hypothetically if all five were on the court: Griffin can pass to Paul, Crawford or Redick, but when Redick has the ball, his only 3pt passing options are Crawford and Paul. 3pt shooters lose opportunities for valuable 3pt assists because they cannot pass to themselves.
This is just scratching the surface of what we can do with the tracking data and passing. Hopefully, moving forward this or a similar methods can be used expand and refine passing metrics to do a better job of assessing playmakers by introducing a more robust quantitative component instead of relying on broad labels such as “floor generals” or “horrible passers.”