Draft Projections and Visualizing Predictors for the 2016 NBA Draft
By Nick Restifo
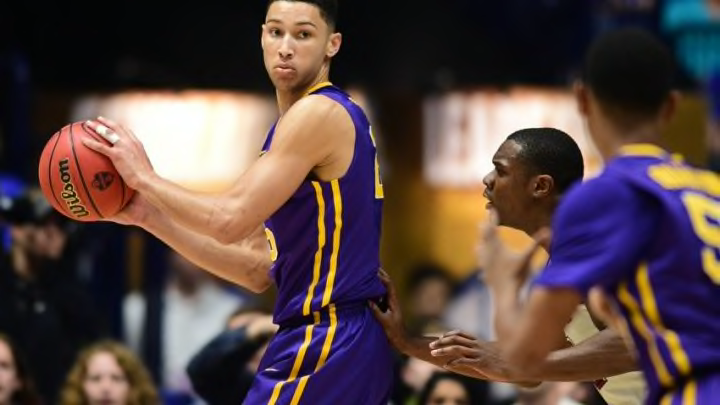
A few months ago I presented early draft model projections that represented a first pass at predicting the success of future NBA draft prospects. That set of projections were made prior to the 2016 draft combine, and therefore used estimated draft combine results for a portion of their predictions. Now that the 2016 NBA Draft combine has come and gone, draft model projections for the entire 2016 NBA draft class can be made using actual combine results for most players, though those who did not attend the combine still use imputed results.
Comparing my first set of predictions to this set would not be an exercise in the absolute change that the projections of players that participated in the combine experienced, however. I also made several minor methodology techniques that increased my projections’ ability to predict out of sample and beat the historical pick order since 2002.
My most up to date results are presented below.
The target variable for this projection system two year career peak blend of RAPM wins, BPM wins, and Win Shares. I use a combination of two ensemble predictions to reach the final numbers presented above, a prediction of productivity assuming NBA play, and a prediction of likelihood of playing in the NBA. For each prediction, a weighted average of five base models is taken based on each base model’s ability to predict out of sample (the more accurate models are weighted more). Random Forests, Gradient Boosted Regression, Neural Networks, Decision Trees, and Least Squares/Logistic Regression are used in each ensemble. I explored the predictive effectiveness of all five base models individually and all their possible ensemble combinations, but I (unsurprisingly) found that taking input from all five base models in each calculation produced predictions with the least amount of error. I take a two prediction, conditional probability approach to the process because it allows my system to be applied to thousands and thousands of prospects, not just those scouts consider to be among the most likely to be drafted. It’s also interesting to compare the different attributes that get a player a chance to play in the NBA versus those that help him succeed once there.
Like many other draft models, Ben Simmons and Brandon Ingram net the top two spots here, and at this point it’s almost foolish to subscribe to anything but the notion that this is a two player draft. All available evidence, objective and subjective, points that way. Though the difference between Ingram the number 3 player (Henry Ellenson) in my draft model is very small, other models and the scouting consensus (probably correctly) consider this gap to be far wider.
Aside from overall results, another thing I thought to be illuminating were these predictor curves that once can create using the plot.gbm function in the gbm R package. These curves represent the non-linear relationships between each predictor variable and the final prediction of the Gradient Boosted Regression base models, after integrating out the other variables. The predictor variables are presented in order of importance, with the top most important variable in the top left box, and the least most important in the bottom right box.
In the GBM model predicting the probability of NBA play, high school rank is the most valuable predictor, before points, games played, and strength of schedule rating. It shouldn’t surprise anyone that highly ranked high school players are very likely to get a chance to play in the NBA. On the other side of the coin, steals, the international control variable, three point field goal attempts, turnovers are all just important enough to warrant inclusion in predicting NBA play, but their curves are barely distinguishable from straight lines.
The most interesting curves here, in my opinion, are the wingspan, max vertical leap, and blocks curves. For the most part, for all three variables, the value added from low to medium values of these variables is roughly the same, until a spike in positive NBA probability that accompanies high levels of all three of these variables.
Like most draft models, the GBM model here predicting NBA production considers age and steals to be among the most important predictors. Note that many of the variables used in predicting NBA play were not even important enough to warrant inclusion in predicting NBA production, not even the single most important predictor of NBA play, high school rank. It seems that being a highly ranked high school recruit gets you the chance to prove your stuff at the NBA level, but it doesn’t help you succeed once there. Indeed, the general trend is that athleticism gets you a chance to play in the NBA, but pre-NBA success in the more tangible measures of production are what differentiate the better NBA players from the worse ones.
NBA production curves are more roughly linear in the NBA production GBM model, with the notable exception of age. Age takes a distinct “U” shape, with NBA production peaking at the lowest ages of 18 and 19, bottoming out and remaining low at 23 and beyond, and actually rising again when players reach their early 30s, an age window when veterans are often plucked from international leagues and prove their value in the NBA. (Think Pablo Prigioni).
When performing any kind of analysis, whether it be draft related are not, it is important to remember that relationships between predictors and target variables are often non-linear, and these GBM curves provide a relatively intuitive way to digest that information. NBA draft models are just one of many methods to assess the value of incoming talent, and just as it is important to evaluate and assess the value of all base models in an ensemble model, it is important to evaluate and assess the relative value of all types of NBA draft projections, completely objective or otherwise.