Guest Post: Home Court Advantage and Shooting
By Guest Post
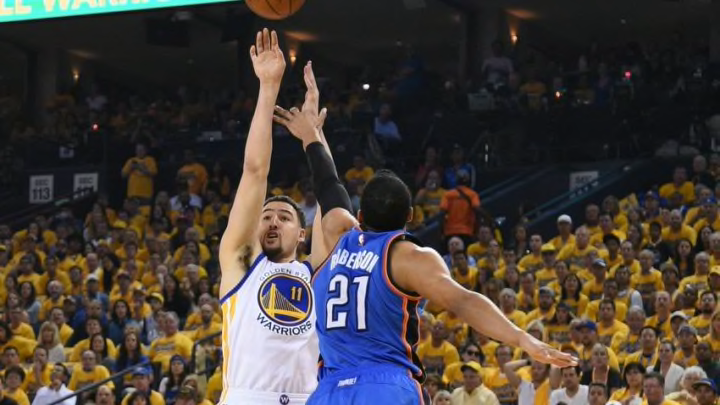
Today’s guest post on the impact of home court advantage on shooting comes from Michael Lopez. Michael is an Assistant Professor of Statistics at Skidmore College. His work can be found at statsbylopez.com or on Twitter @statsbylopez
Released five years ago, the book Scorecasting contains a plethora of interesting examples that will change the way you look at sports. One of the book’s points that stuck with me looked at the roots of the home advantage across different professional leagues. We know that home teams win more often than road teams – in their book, L. Jon Wertheim and Tobias Moskowitz[1. As well as University of Chicago student Dan Cervone, now a data science fellow at NYU who has done exceptional research in applying statistical tools to basketball.] explore why that is the case. By first identifying that players perform no better at home on referee-independent tasks such as shooting free throws in basketball, scoring on shootouts in hockey, or kicking field goals in football, and then by describing unique sets of studies done in soccer, football, and baseball, the authors argue that the primary benefit of playing at home is referee favoritism. It’s convincing stuff, and I encourage readers to check it out if they haven’t already.
It’s with that same ref-driven home advantage in mind that some curious results appeared when looking at shot-level data from recent NBA seasons. The results were somewhat surprising. Even after accounting for several factors that are impacting shooting percentages, including shot distance, location, defender distance, number of dribbles, and time on the shot clock, home teams still shot significantly better than away teams. All together, the findings suggest that there is either some advantage to shooting in familiar confines, or that despite the richness of our shot-level data, factors that would help the home team shoot better remain unaccounted for.
Data and preparation
I used shot-level data for all two and three-point attempts during the 2013-14 and 2014-15 seasons, as generously provided by Krishna Narsu, formerly of the Dallas Mavericks and a contributor to Nylon Calculus.
On aggregate, home teams shot better on both 2-point (49.5% versus 48.1%) and 3-point (36.7% versus 36.1) attempts. But these edges alone don’t entail an advantage to shooting at home, as home team players could be shooting from shorter distances, with fewer defenders nearby, or on more catch-and-shoot opportunities, each of which is linked to increased accuracy. Instead, a more appropriate mechanism for understanding a home advantage would account for several of these factors simultaneously. That’s what we’ll try and do here.
I used two approaches, as detailed below. While I cannot provide the data, the R code and output are on my Github page; feel free to expand on what’s done below, which is far from exhaustive.
Approach one: generalized mixed models for binomial outcomes.
One complication in shot-level data is that there is a natural dependence in outcomes. As an example, Steph Curry shots are more likely to go in than Emmanuel Mudiay shots, and so a model that fails to account for such differences in shooter talent would not reflect the underlying data generating process. Mixed models are attractive in that they can account for both shot-level fixed effects (such as distance), while also accounting for the individual talent of each shooter.
Using terms for game location (home, away), shot distance (linear and quadratic terms), shot clock (early, mid, late), defender distance (very tight, tight, open, wide open), dribble type (catch & shoot, off dribble), height difference between the shooter and nearest defender, and shooter age, as well as a random intercept for each shooter, I fit a generalized linear mixed model (GLM) of shot success (Yes/No) using a training set that included 90% of shots in Krishna’s data.[1. Continuous variables were standardized.]
Within this subset, and after accounting for the other variables in our model, the odds of a successful shot taken by a player at home were about 4% higher than when on the road. Two reasons point to this number being statistically significant. First, the p-value for the game location coefficient (1.6*10-6) was low. Second, when looking at predicted accuracy on the 10% of shots not originally included in the model fit, a model with the game location term outperformed a naive model without game location, as judged by both the log-loss and AUC criterion. Gains in accuracy were admittedly low, but all together, there does appear to be a *slight* improvement in performance when knowing if the shooter shot at home.
As one way of visualizing the regression model results, here’s a coefficient plot from the model fit that included a home court advantage term.
Estimates are shown on the log-odds scale – this means that terms greater than 0, including game location, height difference, and wide open shots, are linked to higher success rates. Each point is also shown with its associated 95% confidence interval.
What’s useful about coefficient plots is that they allow for immediate comparisons of variables. In this model, defensive type (judged by the proximity of the nearest defender) and shot clock stand out as the most significant predictors. You’ll notice that terms for shot distance are excluded; these coefficients were substantially more important as far as model fit that they rendered the rest of the graph unreadable. This means that, unsurprisingly, shot distance is really important.
Approach two: Regularized regression with cross validation
While the mixed model framework described above provides intuitive interpretations of our home advantage term, in general, it may not provide as accurate of a set of predictions as more advanced machine learning tools.
As a second method, I decided to use a the glmnet function to estimate the chance of a successful shot using a fitting technique called penalized maximum likelihood. One strength of this approach is that it can include cross validation when estimating model coefficients, which prevents overfitting and allows me to look at the interaction of several shot-level factors. Additionally, a separate term for each shooter can be included, which again can help account for differences in shooter talent.
Two glmnet fits were implemented on the training data, one with game location and another without game location. The estimated coefficients were then used to extract two sets of estimated success probabilities for the 10% of shots in the out-of-sample data. Once again, there were slight gains in the accuracy when using a model that included game location.
Putting it all together
After using two statistical tools that can account for other shot-level factors, there appears to be a small home advantage with respect to shooter accuracy. This suggests that either (i) shooters are more accurate in the confines of their own arena or (ii) our approach still does not pick up on other factors that link to both shot success and playing at home. Unfortunately, until we can randomize shooter locations while using identical sets of other game-factors[3. A statistician can dream.], our models cannot show that conclusion (i) is the truth.
There are a few other interesting points to consider. First, one of our approaches for gauging prediction accuracy, AUC, can provide some evidence as far as overall model performance. A perfectly accurate model would yield an AUC of 1.00, while a random predictor, like a coin flip, would score a 0.50. In this example, the highest AUC on out-of-sample data was provided by the glmnet that included game location. However, this AUC (0.64) was quite small, and indicative of an overall poor performance.[4. Glmnet fits performed slightly better than the mixed model ones.] Altogether, while this is just a first step in predicting shot success, it is humbling to know that even when given information like shot distance, the shooter, and type of shot, much of whether or not a player’s shot goes in is left to chance.[6. Ed. MAKE OR MISS LEAGUE!] As comparisons, these predictions fall well short of those looking at batted balls in baseball and first round picks in the NFL.
Second, there is the matter of practical significance. Using a sample of 100 games (200 offensive teams), I estimated the overall probability of each shot going in, had those shots been taken both at home and on the road. Across each game in the sample, the expected number of points scored given all shots taken at home was about 1.48 points higher than had those shots been taken on the road.[6. The expected number of points for a shot is found by multiplying estimated shot probability by the number of points that each shot was worth.] On average, a home advantage was worth between 0.8 and 1.8 points per game as far as team improvement in shooting.
Finally, we know that NBA teams win about 60% of their games at home; related, betting markets generally estimate that playing at home makes for roughly a 7-point swing relative to playing on the road. Given the results shown here, there’s a chance that at least some of that 7-point swing, but likely no more than a point or two, can be accounted for by changes in shooter accuracy. If that were to be the case, the home advantage in basketball would lie not only in referee judgement, but also in shooter performance.