Projecting the 2016 rookie class forward
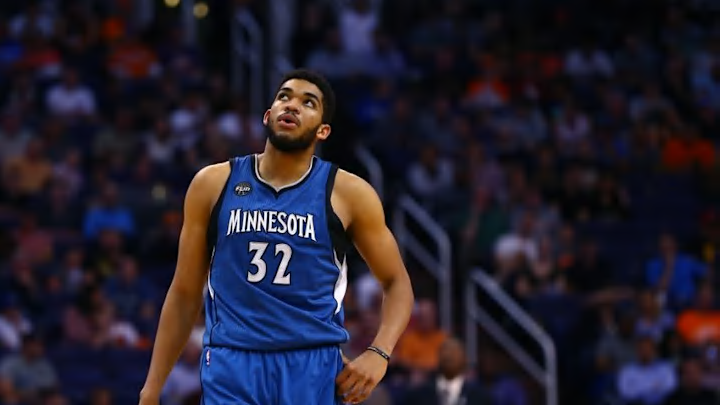
Over the past few years I have been using a few analytic models to help project rookies’ development towards the end of their rookie contract. The models combine data from the rookies’ pre-NBA career, age, and rookie stats to project the players’ peak performance in their third and fourth years, a critical time for both the player and the team in terms of providing contract value for the team and setting the player and team up for restricted free agency.
I looked at two different measures, one keyed on efficiency using a box score based metric, Alternative Win Score (AWS) efficiency adjusted for minutes played and one that multiplies AWS by minutes played to AWS Production similar to Win Shares (And in fact is highly correlated to Win Shares, but slightly more stable because of less emphasis on scoring efficiency).
More from Nylon Calculus
- Nylon Calculus: Reviewing preseason predictions for the Western Conference
- Nylon Calculus: Reviewing preseason predictions for the Eastern Conference
- Nylon Calculus: LeBron James and the slam dunk aging curve
- The Whiteboard: Why has the NBA seen so many 50 point games this season?
- Nylon Calculus: 15 early season predictions for the NBA Western Conference
The primary difference between the two models is the weight given to minutes played in the players’ rookie year, with more weight given in the AWS Production model. The Production model may be better able to separate stars-in-the-making from role players. However, interpreting minutes played can be tricky, injuries can distort minutes, the rise of the D-League could change the relationship between minutes played in the NBA and future success, and occasionally a high draft pick is force fed minutes with little future development.
In any case, I applied both models to the 2016 NBA rookie class. The AWS Production model gave results that largely fit with the general consensus among NBA writers and fans with Karl Anthony Towns leading the field by a good margin, followed by Nikola Jokic, D’Angelo Russell, and Kristaps Porzingis. The rest of the top twenty are below:
There is the somewhat surprising projection of D’Angelo Russell surpassing Porzingis, a projection built both on the factor that back court players tend to show more growth after their rookie year, at least in terms of box score production, and Russell’s superior production prior to being in the NBA. Also, a surprisingly low growth projection in production from Towns, that mostly has to to do with regression to the mean in the model from such a stellar rookie performance.
Here’s a visualization of the production model using all of the rookies I had adequate data to create projections for that highlights the relationship between production, age and minutes played:
While there is a high degree of correlation between the Production model and the Efficiency model there were some differences again primarily centered on playing time in the player’s rookie year. Here’s the top twenty ranked by AWS Efficiency projection model:
In this model the expected difference between Towns and Jokic is much narrower, as reflected in most of their efficiency numbers.
The biggest relative beneficiary moving to the efficiency model is Spurs garbage time destroyer Boban Marjanovic, an older undrafted rookie out of Europe. Marjanovic is sort of what analysts sometimes call an “edge case” for the model, in this case meaning he much older than most of the rookies in the training model and there were few cases with production as efficient as Marjanovic’s who played as relatively few minutes. Added to that is Marjanovic’s absolutely stellar numbers the last two years in the Adriatic League and Euroleague before coming over to the NBA.
Another player the model is higher on than the general consensus at the moment is Jahlil Okafor. The biggest reason for this is simply the measure used to train both models rely heavily on box score measures that are by their nature less able to capture defensive production, the area where Okafor struggles. But, it is also worth noting the model does capture Okafor’s youth and very high NCAA production, things which might be overly discounted at the moment. With the logjam in the front court in Philadelphia, there may be a buy low opportunity according to the model. (The same with Richuan Holmes as well, if in a less dramatic fashion).
Both models are, of course, just projections. For the sake of stability in the modeling process I did not attempt to include every detail or bit of context that affected a player’s future development, like injuries, the nitty gritty of team depth charts and opportunities, or injury risk, nor could I have. Those things would have to be considered separately in any individual player evaluation by a team. But, the results for both certainly represent a big leap forward in terms of accuracy over my and pretty much any other available draft model.