Nylon Calculus: College basketball historical plus-minus data
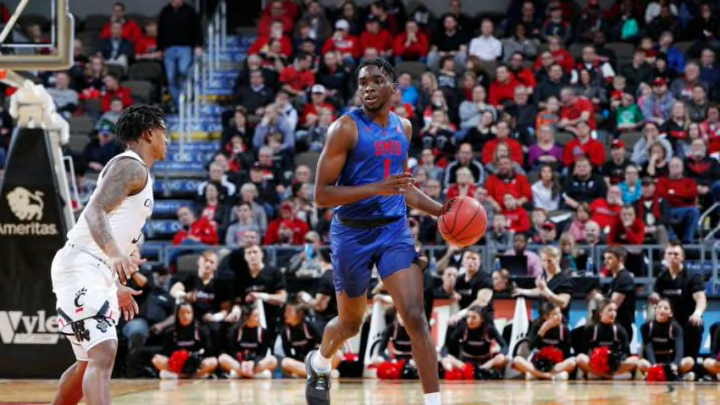
Plus-minus stats have become part of the everyday vernacular in the NBA. From simple plus-minus to NBA WOWY and the development of more sophisticated metrics like RAPM, RPM, and PIPM, it’s become a part of how many of us process the game.
Stats with a plus-minus component are not necessarily perfect. It’s been demonstrated that plus-minus and its more sophisticated derivatives are volatile over the course of single NBA seasons – let alone a college season that’s less than half as long — and should not be trusted on their own, with no context or additional investigation. But these stats are an incredibly valuable tool, an intriguing way to go beyond simple box score stats and get at the simple question behind almost all basketball analysis — who’s contributing to winning games, and how are they helping their teams do it?
As someone whose interests are largely in the college game, the ready availability of these stats in the NBA — and the high quality play-by-plays that let you build them — has been frustrating. The quality of play-by-plays in the college game are at best inconsistentm rarely include substitutions, and the sheer number of teams (about 350 in a given year) can make it more difficult to analyze. There are paid services that provide college lineup and WOWY (with-or-without-you, in case you made it this far in this article without already knowing) analysis, but this type of analysis is largely out of the mainstream in the college hoops discussion.
With this post, I’m aiming to start contributing to making this type of data and analysis at the college level more accessible to any basketball fan with an interest in these sorts of things. This has taken way more time than I’d like to admit, but I’m happy to finally debut on-off offensive ratings, defensive ratings, and net ratings for every player in college basketball back to the 2009-10 season.
What I’m sharing here is largely restricted to links to google sheets containing data from each of these years. The documents contain number of possessions logged for each player on offense and defense, as well as offensive and defensive ratings (simply, points scored while the player was in or out divided by possessions in or out).
As an example of what you’ll see — here’s the top-30 leaderboard for on-off net ratings for single players I have in my sample (minimum of 400 possessions played):
Drexel’s Frantz Massenat (2011-12) and Syracuse’s Michael Gbinije (2015-16) take top honors on this board. The sheets are generally presented alphabetically, but you should be able to download into Excel and do your own filtering pretty easily. The year I listed in the sheets is the ‘first’ year in any college season (i.e. 2016 is 2016-17).
Having just finished this analysis, I wanted to put it in the public sphere so anyone who wants to can poke and prod through. I’m working on a web application that will use the raw data behind these sheets to build a freely accessible platform for WOWY stats, both on the individual and team level, back to 2009-10 in college basketball — and I’m sure there’s still some cleanup work to do in there. Once finished, it will likely be posted on The Stepien, a free draft website with an excellent collection of truly talented writers & analysts.
The Data Dump
But, here’s what you’re really here for. These are the links to the Google Sheets:
2009-10 to 2016-17 Net Rating Sheet (all games)
2009-10 to 2016-17 Net Rating Sheet (KenPom top 100 opposition)
2009-10 to 2016-17 Net Rating Sheet (Conference)
2009-10 to 2016-17 5-Player Lineup Leaderboard (min. 150 possessions)
2009-10 to 2016-17 4-Player Lineup Leaderboard (min. 250 possessions)
2009-10 to 2016-17 3-Player Lineup Leaderboard (min. 400 possessions)
If you’re looking to compare to a service that already does this — such as Hooplens — you’re going to find that the data rarely match up perfectly. I don’t know what their methodology is (for fixing bad subs, calculating possessions, etc.), so I can’t speak for what explains the differences. Read on if you’re interested in the general methodology, and some info on what I put into each sheet.
If all you’re here for is the data, there’s plenty above to pick through. Enjoy!
Methodology
I believe in general transparency (though don’t ask me for the code — this was entirely done in Excel with VBA), and I know these stats are not built on something perfect. Here’s how I got there:
The work starts from play-by-plays available from stats.ncaa.com, the only publicly available source I’m aware of that includes any substitution data. They’re also quite excellent at getting most of the games for each season, something that can’t be said for most other publicly available sources.
Something I’m acutely aware of is that these play-by-plays are not perfect. There are misspelled names, varying team names in the same season, games with only one half, games that use numbers instead of names (and the numbers — of course — are often associated with no one on the team), and a few (maybe 40-50 total) that are flat-out missing or completely flawed/unusable in the full historical sample. And that’s without getting to the substitutions themselves, which are quite often imperfect — taken by themselves, there would often be 10 players (or 2) in the games.
Approximately 40-45 percent of the games in the sample did not have errors in the substitution data. Five players were in the game for all D-I sides (I didn’t bother with non D-I teams), and listed play-by-play actions were not being completed by a player not currently in the game.
The rest were ‘fixed’ using the play-by-plays. At any point where a team had more or less than 5 players on the floor for more than a line, I used automated tools to search down the play-by-play for players who, in the same half or overtime, did something in the game besides enter it. This process was repeated at each point where a bust occurred, to try and minimize errors.
This method of fixing certainly isn’t perfect. There are points at the ends of halves and overtimes that necessarily end up with less than 5 players, because the routine doesn’t have the space to find five unique players that (based on the play-by-plays) are certainly in the game. And I know the names used aren’t always correct — I’ve found women’s CBB players in these play-by-plays, and something around 40,000 unique misspellings for D-I players alone — and I don’t doubt there are some errors in the sequencing of the play-by-play lists.
This is a long way of saying: know that what you’re getting here isn’t perfect, because the source the analysis is based on certainly isn’t. But I’ve put a lot of work into ensuring what you’re getting is as close as I can make it to correct.
Next: Nylon Calculus -- Trey Burke is finally making jumpers
What’s in There
Have the usual suspects for the basics of this analysis — offensive and defensive possessions logged, plus-minus, and calculated offensive and defensive ratings for the teams during those spans. The possessions are calculated from play-by-play logs, rather than estimated.
I’ve also added columns that show the average offensive and defensive KenPom rankings each player (and five-man lineup) logged their respective possessions against, to give you an idea of the quality of opposition each player faced. Non D-I teams defaulted to 351.
I apologize for any bugs — and I’m quite sure there are some — and would certainly appreciate you pointing them out. It’s a ton of data for one person to spot-check, and I always appreciate the extra eyes.