Nylon Calculus: Recapping the Great Lakes Analytics in Sports Conference
By Vijay Vemu
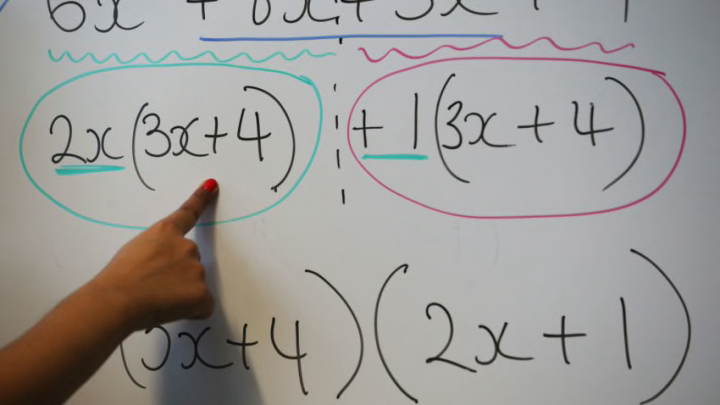
One of the amazing things about the growth of sports analytics is the proliferation of academic conferences where this is the focus. This summer, I attended the Great Lakes Analytics in Sports Conference hosted at the University of Wisconsin-Stevens Point. The conference is only in its second year but still had a good deal of intriguing topics, with quite a number of them being basketball-related. Here are some of the cool basketball talks I attended during the nine-hour conference on a Thursday in June.
The value of free throws in the NBA
The obvious phrase “you have to make your free throws” is tossed around during almost NBA broadcast and rightly so. As the term says, it’s a free point you have a chance to earn. Andres Alvarez (@NerdNumbers), a basketball writer and top Twitter follow in the sports analytics community, gave a great talk on the importance of free throws. He showed some well thought out graphs/charts on how free throw percentage can affect a given game and explained how making your free throws literally is the difference between wins and losses.
Finding a new way to make basketball better at the end of games
The Basketball Tournament has become a summer fixture and this year the experimented with some new rule changed to up the excitement level. Nick Elam (@ElamEnding), who works as an Assistant Professor of Education of Leadership, came up a method called the Elam Ending which is a way to make the ending of games more efficient and less time-consuming.
Elam noticed the obvious change in duration and quality of play which happens at the end of most basketball games. Teams are hacking to put the opposing team at the free throw line and basically extending the game. While it could possibly lead to some brilliant finishes like Ray Allen’s game-tying 3 in Game 6 of the 2013 NBA Finals, it often leads to nothing at all. The Elam Ending changes all of that. It makes games more efficient and as Elam mentioned a lot throughout the talk, allows teams to run their normal offenses instead of scrambling to find a semi-decent 3-point look and chuck it.
The first thing it does is the remove the game clock, hence taking away the race against time panic we see from teams who are losing late in games. It also sets a target point total in which one team has to hit and the game is over. Essentially it’s the same as you would have it when you were playing basketball as a kid with your friends. Whether it was first to 21 or 32, the team which gets there first wins, this is exactly what the Elam Ending does. One example the speaker gave was in the NCAA title game between Michigan and Villanova. The game ended up being a blowout and the last 10 minutes of it was unwatchable. Using his method, Elam showed us what the target score would have been and how much earlier the title game would have ended.
He also went more into his new proposed rules he would bring about to the TBT. Elam described the different ending game scenarios some of the coaches in The Basketball Tournament could use. This was a way to ensure the quality of play would stay throughout the game, especially in the final minutes.
How possible NCAA rule changes could affect the game
This was the first presentation where the speaker, Scott Nestler of Notre Dame (@ScottNestler), specifically talked about how coding and sports analysis can go together. Nestler showed us how he scraped NCAA data during the regular season, NIT, CBI, and March Madness. Using R to gather a bunch of this data, Nestler also showed how some NCAA rule changes, which were used in the NIT, affected such data. The rule changes included changing of the court size, pushing back the 3-point line, and changing from halves to quarters among other things. A lot of questions about how these changes will affect the pace of play, 3-point shooting, and turnovers were presented. Nestler analyzed data from all different tournaments, juxtaposed them, and gathered answers from the results. It was super informative and credit to the speaker for also talking about the packages one would need to install in R/how the code works when it came to finding the data.
Throwing out stats when the game is over in NCAA basketball
Now, this talk’s main topic wasn’t specifically about the title I just wrote above. It was a small part of it but it stood out. In college basketball, there are few advanced metrics and sites which we can pull conclusions from. Ones like KenPom are famous and the best when trying to find clues about certain teams, especially heading into the tournament in March. But what about some of the other ones? Erik Haslam created his own website (@Haslametrics) and it’s filled with numerous CBB statistics one could use. One interesting part about it is when Haslam explained how he dumped out of the data in games when it was deemed “over”. It’s often a big talking point of when the tracking data in certain situations become “useless” or not. You can’t always implement statistics in a situation where you have the end of the bench players on the court. Haslam explained the different metrics he uses to determine the rankings for his site as well as what goes behind his projections part of his site which predicts which teams deserve to make the NCAA tournament come Selection Sunday.
A different method of analyzing NBA lineup data
Lineup data has become a common statistic in the basketball community. It’s often used to determine how effective certain players can be or how their roles can be more clearly defined on a given team. Stephen Devin showed the different ways we can use lineup data and not just in the standard 5-man lineups. Devin used combinations of 2 to 4 player lineups in his analysis. He then explained how it can help NBA teams learn more about their team structure as well as for coaches to gain more insight on their players.
Next. Color-mixing and lineup synergies. dark
Basketball analytics trickling down all the way to high school
One great thing about the advancement of analytics in basketball is how it has gone in terms of growth. Kyle Allen, who is a coach at Pine City, a high school in Minnesota, is just another example of the future of analytics. Unlike most high school teams, which run very simple offenses and ones which are not always taking the most efficient shots, Allen’s team has flipped that on its head. Allen encourages his players to take only 3s and shots at the rim (which are the best shots as we all know). A mid-range shot is not acceptable.
Obviously, Allen’s methods of coaching and style weren’t always popular among the community but he stuck with hit and greatly improved his team. Now his side is one of the best in the state and, as you expect, one of the best 3-point shooting teams. In the talk, Allen gave what inspired the change, how his practice methods, which requires self-evaluation reports from his students every day and tracking stats hand by hand in each game has helped him learn more about the game. The talk offered a nice change of pace and showed us that analytics has reached even the lower levels of basketball.