Nylon Calculus: Which players deserve more shots on offense?
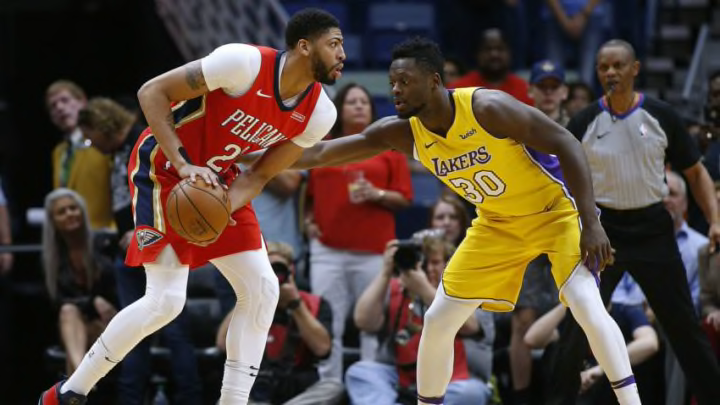
The idea of maintaining shooting efficiency as volume increases has been analyzed dozens of times before. Most of the previous analysis compared either effective field goal percentage (eFG%) or true shooting (TS%) with field goal attempts (FGA) or usage rate. With this comparison, we could then predict which players could increase the amount of FGA while maintaining efficiency.
I am choosing a simple comparison of eFG% and FGA but take it a little further and look at the types of shots. I will attempt to quantify tough shots versus easy shots and borrow some ideas from fellow Nylon Calculus contributors here and here. Armed with some very colorful groupthink and a few interactive visuals I will attempt to find some efficient scorers. By the end of it, we might even get to play a version of basketball “red light, green light”.
My analysis starts with the theory that players who take a lot of tough at high efficiency should be able to increase their workload without much loss of efficiency. On the other hand, players taking easy shots are less likely to maintain efficiency with an increased workload. This theory is derived from the idea that as players shoot more and score at a higher rate, opposing defenses will give them fewer open looks.
The goal is to find players who take tough shots at high efficiency. To understand tough shots we need to first understand easy shots. The easiest shots in basketball are shots that are assisted, close to the rim, and wide open. Even the worst offensive players would have a high efficiency if all they had to do was catch a pass and shoot a layup without any defenders near them.
To define easy shots statistically, we can use the percentage of a players shots that are assisted (AST_FGM), the percentage of restricted area shots (RA_PCT), and the distance of the closest defender. The closest defender is defined by four categories of distance ranging from very tight (0-2 ft), tight (2-4 ft), open (4-6 ft), and wide open (over 6 ft).
To begin our analysis I started by looking at the first two dimensions of shot difficulty by comparing restricted area assisted shots and unassisted shots. In theory, players with a high percentage of unassisted shots (UAST_PCT) are players that are creating their own shots. While players with a low UAST_PCT rely on their teammates to pass them the ball to make their shots. For example, a player with 0.8 UAST_PCT creates 80 percent of their made shots without an assist. The second dimension includes the percentage of shots near the rim that were assisted (RA_AST_PCT). Receiving a pass and finishing at the rim is typically one of the easiest types of shots in basketball and indicates a higher reliance on teammates. An example of RA_AST_PCT of 0.6 means that the player makes 60 percent of all of their made shots at the rim with the help of an assist.
Looking at the above graph we can see there are some natural spots to partition these data points into groups. Instead of just eyeballing it we can use one of the more simple clustering algorithms and let a computer do this for us. K-means clustering is one of the simplest clustering algorithms, both in terms of the math involved and the implementation in computer programming. K-means typically starts out by randomly selecting centroids for k number of clusters. In this scenario, I chose k=6 clusters, we start with 6 randomly chose centers for our clusters. The computer finds all of the points that are closest to that centroid using the standard distance formula. This process of selecting centroids, finding the points closest to each centroid, and minimizing the distance between each centroid and its points is iterated. The iterations stop once each point remains in the same assigned cluster. The graph below is the outcome of this process.
As you can see from the graph, the three previously discussed groups as well as three new ones. Skilled Finesse players have a relatively high UAST_PCT and low restricted area percentage. These players do most of their work away from the rim but use slightly more help from teammates than the Creative Scorers. Players in this category are LaMarcus Aldridge and Giannis Antetokounmpo. Skilled Paint Dwellers are lower unassisted but higher restricted area percentages. Players such as Anthony Davis, Andre Drummond, and Pau Gasol make up this group. Finally, there are the Assisted Finesse players with low unassisted and low restricted area rates. Al Horford, Jayson Tatum, and Aaron Gordon are typical players in this group.
While the analysis of the first two dimensions of assisted shots and restricted area shots gave us our groups of scorer types, the third dimension of closest defender distance will give us our last set of parameters. The interactive visual below allows for hovering over a player to find their relevant statistics as well as filtering by scorer type. The visual incorporates all three dimensions or our analysis and I like to call it the traffic light plot.
The width of a player’s traffic light is determined by their non-restricted area percentage (NON_RA%), a wider box means a lower restricted area percentage. The height of the player’s traffic light is determined by their unassisted percentage (UAST%). More shots unassisted means a taller box. Finally, the colors of the player’s traffic light show the percentages of shots taken with the distance of the closest defender. Red equals very tightly defended, orange shots are tightly defended, yellow are open shots, and green are wide open shots.
You can see the full visualization here as well.
I like pretty pictures and interactive visualizations as much as anybody but what can we do with this information? The goal of this analysis was to find which players might be able to increase their workload and maintain efficiency. My theory is that players who take tough shots should be able to maintain efficiency as their workload increases. Taking more tough shots might be correlated with poor shot selection. Whether the players are taking tough shots due to their own poor choices or out of necessity, tough shot makers will be more likely to maintain efficiency compared to easy shot takers.
To find players that could maintain efficiency I will look for players higher than average eFG%, with larger traffic lights, and more orange and red lights. My pick for increased workload from the Creative Scorers group is Elfrid Payton. I have actually written about him before and I think he could be an asset on a team with quality offensive players. Speaking of quality offensive players on the Pelicans, my second choice is Julius Randle from the Skilled Paint Dweller category. Both Julius Randle and Elfrid Payton should benefit from being surrounded by other great offensive players. There might not be enough shots for both of these players playing with Anthony Davis and Jrue Holiday, but I expect them to remain highly efficient scorers.
My final two choices come from the Skilled Finesse group with Ben Simmons and Enes Kanter. Kanter has almost 0.6 eFG% and takes over 80% of his shots against very tight or tight defenders. With some help from any other Knicks, such as Porzingis coming back, there is no reason he can’t maintain his efficiency even while taking a few more shots per game. Ben Simmons is similar as he takes only 12.3 shots per game with 73.7% of them being contested. Simmons also has a very high unassisted percentage at 65.6%. If Simmons can shoot with .545 eFG% with these types of shots and without being a long-range threat, I would look for him to increase his workload and efficiency next year. These four players, Simmons especially, would have the green light offensively next year.
All data is from stats.nba.com.