Nylon Questions: How does coaching affect a team’s performance and player development?
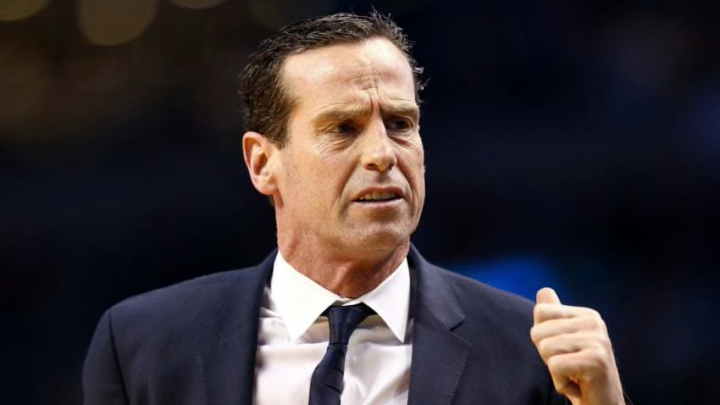
Sports analytics is a constantly evolving field and keeping up can be a challenge, especially with so much work being divided between the public and private spheres. As we head into the 2018-19 season, Nylon Calculus wanted to take stock of where we are and of what’s coming next.
This project is a throwback to work Keith Woolner did for Baseball Prospectus nearly two decades ago, and an update to Kevin Pelton’s basketball-specific version from five years ago. Our staff compiled 10 questions whose answering will likely guide the next few years of public analytic work. Not knowing what has been accomplished in private by NBA teams and consulting firms, we focused on questions that could be worked on in the public sphere, wouldn’t have to be answered with existing datasets (we don’t want to imagine the data we have now is all we’ll ever have) and things that would theoretically have an effect on how teams operate on and off the court.
Hopefully, these questions will help spark, refocus, and recalibrate conversations and lead to collaborative progress here at Nylon and everywhere else sports analytic work is being done.
5. How does coaching affect a team’s performance and player development?
I think it’s important to note off the bat that that question actually contains two different questions: “How does coaching affect a team’s performance?”, and “how does coaching affect player development?”. Lumping those two together would be like asking how good is a player on offense and defense, and then answering with a mere “Oh he’s good”, only you’ve asked that question about Damian Lillard and you forgot to answer half the question in answering the whole thing as one piece.
And then you can fracture it down even further. Let’s say you asked that question about Cody Zeller. Is he good at offense? Yeah. Is he good at defense? Sure. Is he good at isolating players on offense and scoring while killing the clock? Not really, no. But in failing to properly specify the question enough, you miss that detail.
But it’s fairly uncommon to do that for coaches. Is Terry Stotts good at developing guards? Maybe. Is Steve Clifford bad at designing inbounds plays? Probably. Is Tom Thibodeau using the rotation pattern that makes the most sense for his personnel? Almost definitely not, but that’s a degree of specificity that you usually don’t see in evaluating coaches. That’s in part because those kinds of questions require a large amount of team context, have a lot of personnel personality juggling required, and ultimately, some might not be all that repeatable in some ways. But that is exactly what makes them a perfect candidate for a data-driven answer.
So then as we go through three key questions that should provide a basis along which the question should be answered, keep in mind the high level of fracturability within the potential answers. Just because we know something currently doesn’t mean it can’t be refined, and the questions we’re asking may not even matter in view of other things we can learn. So then, the three questions:
Why is it important?
Front office staffing, and coaching as a part of it, differs from direct player personnel moves in that there are virtually no rules governing or limiting it. Your budget is only limited by the owner’s pockets and willingness to spend money. There’s no coaches’ draft, and coaching free agency is completely unrestricted. Coaches can move between teams as they please, or at least as far as their contract allows them to. And the only limit to the allowable number of assistants is the limit at three coaches on the bench during games. In a practice setting, you could bring five coaches per player and run afoul of nothing.
That lack of a ceiling on spending or recruiting gives teams much larger margins in affecting their chances of success. Teams should be able to gain a recognizable advantage by investing in their coaching staffs, and they have a lot more room to do that than they would with player personnel decisions, which are highly limited by draft slots and free agency rules. But teams can only do that if they can concretely identify who is and is not a good coach, and whether or not the particular skill set of a coach lines up with what the organization needs.
Getting even more granular, though, the individual skillset can also affect personnel decisions. Say you were the seemingly inevitable successor to Scott Layden in Minnesota, deciding how to pursue the next iterations of your franchise. Your analytics department presents to you information that the rotations did not match the personnel, and also directly hurt a specific player. Let’s say that player is Jeff Teague, for argument sake. You’re deciding how to handle Teague’s contract, how to handle Tyus Jones, and how to handle a potential prospect point guard in the draft. Because of your evaluation of the coach’s actions and abilities, you decide to hold off on trading Teague, but also be more ready to take the point guard in the draft by the time that rolls around. In that way, the coaching analytics spread throughout the rest of your decision-making.
What do we already know?
From a publicly available analytics standpoint, what we know is extremely scattered, much like the individual questions here can be.
We have measurements for how teams distribute lineups, but no great way to connect that to actual effectiveness.
We have a highly generalized player development model from controversial Southern Utah University professor Dave Berri, but I have only seen it referenced in a sports economics textbook that he produced in PDF form, which I lost access to when I was six months out of undergrad and my alma mater disabled the Microsoft Outlook access for my old student ID. Based on my recollection of the study, however, it basically ranked coaches on the probability that for a given player who played both under the coach in question and under a different coach, did they improve in your choice of aggregate stat. His was Wins Produced, but there’s no reason the same methodology couldn’t be repeated with a more modern aggregate.
I believe somewhere there exists a study that indicates that Sideline Out of Bounds and Baseline Out of Bounds “set plays” tend to have highly variable success rates from year to year, but I can’t actually find such a study in any of the expected places and none of my colleagues here at Nylon Calculus are familiar with the piece in question.
And if it feels like I’m kind of reaching for “things we know”, that’s because I definitely am. A more accurate way to phrase it is probably that what we know is not much. At least not in the public sphere. The teams, privately, have some investment into coaching analytics, with at least the Nets, Pistons, and 76ers having a staff member directly tasked with exactly that. Further, the current holder of the job with the Nets, Logan MacPhail, previously held a similar job with the Spurs, which I assume didn’t just cease to exist upon his hiring with the Nets. For those teams, there may be a litany of information that hasn’t made it to the public yet, and it may never make it public.
In the public sphere, however, neither of the usual suspects, Nylon Calculus and the APBR forums, has much to offer in the realm of coaching analytics. In fact, in the last six months the only article between the two sites that was directly about coaching was the piece on Steve Kerr linked above. Which leads to question 3:
What are the practical barriers to answering the question?
The reason why we don’t see coaching analytics done heavily in the public sphere, I would propose, is primarily about the nature of the data.
To start, coaching data is not highly centralized. Basketball-reference doesn’t give it in an easily scrapeable form, and as a result, building any database with which to work coaching analytics would require some slightly more proactive coding than most projects.
Further, the data that you would use to answer your questions is still fairly young. If you want a measurement of how a coach structures his rotations, sure, you have 18 years of data and probably have enough, but if you want stuff like how often your coach’s scheme has a player in position to contest a shot, you have four years of data, during which a lot of coaches haven’t seen their jobs turn over, meaning that several players have only one coach for their entire career for something that seems likely to be highly player dependent.
And of course, there are smaller things. You can’t do a longitudinal study as you would with players, a study like the one that produces Real Plus-Minus, since the coaches are practically never symbolically off the court. Assistant coach data, as I discussed in an article for the Step Back about the age of the new Hornets’ staff, is virtually nonexistent, to the point that there isn’t even a well-maintained list of who currently has a job, so you are basically only looking at head coaches or staffs as a whole for potential analytics targets.
But beyond the data, there’s a second major reason you don’t see much in the way of coaching analytics in the public sphere, and that’s just in the nature of the questions. The fractured nature of coaching questions makes it an incredibly broad field, and you’re never going to solve the whole thing at once. Coaching is just too complex, too diverse. And that itself will drive people away. With the draft, you can build one model. With player valuation, you can build one model. With coaching, not only does a coach have to be able to perform in a variety of circumstances, sometimes those circumstances can change before you even coach a game, as they famously did with David Blatt and his unfortunate timing next to LeBron James’ return to Cleveland.
Next. How will spacing and shot selection evolve to maximize offensive efficiency?. dark
So with coaching, there’s not much more than a few shavings off the tip of the iceberg that we’ve tackled, even though it’s such a huge part of what could cause teams to get better, and cause our prediction models to become more accurate. Ultimately, those are some of the biggest goals in analytics, and we haven’t even grazed the surface there. And we may need to wait for some of the data to get a larger sample size behind it, but ultimately all of this is information that everyone would benefit from the development of.