Nylon Calculus: Deflection recovery rate as an element of luck
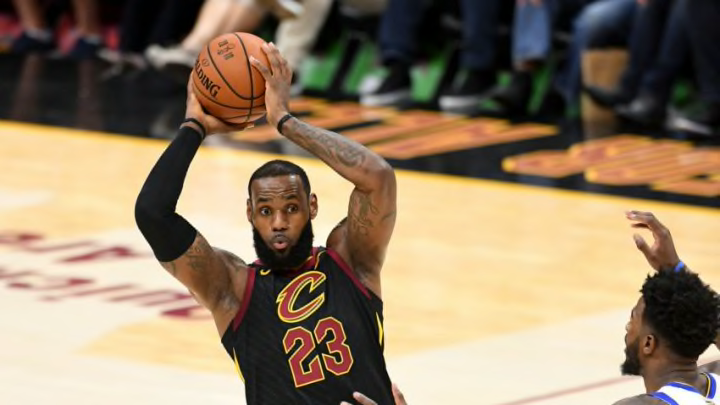
Luck adjustment plays a part in all statistical analysis of basketball, whether explicitly or implicitly. This article seeks to frame a new dimension by which we could adjust for luck — the rate at which deflections are recovered.
A little over three years ago, on June 1, 2017, a weird statistical anomaly happened. In Game 1 of the Finals, between the Golden State Warriors and Cleveland Cavaliers, the Warriors recorded a total of 12 steals on 17 deflections. That .706 ratio was high — league average at the time was around .440 — but it paled in comparison as an outlier to the Cavaliers recording zero steals on 8 deflections total, for a ratio of 0.000. And at the time, threeish-years-younger Joseph Nation, watching from his grandparents’ house in a small Akron suburb named Mogadore and trying to come up with a statistical justification to his grandparents for why the Cavaliers were fine despite losing by 22, started to question whether or not that was a purely random event. At the time, the data didn’t exist to answer that question.
Well, the rest of the series was not particularly close — the Warriors won Game 2 by 19, Game 3 on a fairly well-known Kevin Durant shot, and then didn’t blow their Finals lead. But in the course of the remaining four games as the Cavs were discovering that Kevin Durant did in fact join a juggernaut, the pattern of deflections completely reversed. Not only did the Cavs deflect the ball more frequently than the Warriors, 72 to 57, they also recorded 36 steals to Golden State’s 26, meaning that their .500 recovery ratio also was ahead of the Warriors’ .456.
Mathematically, when you want to look at how repeatable something is, the best thing to do is to compare two large samples. In basketball, that often means comparing one year, Year Y, to the next, Year Y+1. Since there’s often a fair bit of continuity over that short a time frame, you don’t usually see wild overhauls in style that quickly.
We’re going to look, then, at the linear regression of the ratio of steals to deflections in year Y for each team onto the ratio of steals to deflections in year Y+1 for each team. We will do the same with the ratio of opponent turnovers to deflections in year Y and year Y+1.
In fact, the most famous example of a case where year Y fails to predict year Y+1 at all is 3-point defense. The ability of teams to control the percentage of looks converted from basically anywhere except right up near the basket is so low that it doesn’t represent itself statistically, to the point that luck-adjusted RAPM, which forms the core of PIPM, one of the most prominently used aggregate statistics, actually specifically aims to strip out variance caused by 3-point defense luck by calculating what the net rating would be if their opponents had made 3-pointers at an average rate.
If we know recovering a deflection is just luck, can that be used for a larger purpose?
And ultimately, that’s the end goal for this analysis: Now that we know that deflection conversion ratio is heavily luck-based however you form it, it also makes sense to control for it in any luck-adjusted analysis like we do 3 point defense.
Now, stepping back for a second, this isn’t as straightforward as “Let’s go do it”. Hence why there’s not a metric at the bottom of this post with an updated LA-RAPM.
For a minor thing, whoever builds this is going to have some less than straightforward philosophical decisions to make. When removing 3-point luck, we can reasonably state that if the same attempt goes from falling 38 percent of the time to 35 percent of the time that points per possession decreases by .09. It’s probably a little more complicated than that in reality since there’s probably some confounding factors as a result of spacing, but using three times the change in percent is never going to be called unreasonable. But what happens when a deflection goes from being a steal to not being one? You can estimate the point value of a steal, sure, or reject any possession on which a deflection occurs, but both of those have potential issues.
For another, it’s unclear how much of this is purely a result of how tracking data defines a deflection. If the way that a deflection were defined were somehow not picking up valuable information, then of course the result would be meaningless, and so would the conversion rate. Since this is unclear, it’s not certain how valuable it is to know that deflection rate is random, though we can partially mitigate this by showing that deflections are correlated to steals at large, since steals are valuable, and therefore deflections would also be valuable. That regression is shown here:
But most crucially, incorporating this into LA-RAPM is currently not plausible because the data is not available in a sufficiently granular form. No matter how you would incorporate the data into an LA-RAPM, you would have to have some way of determining how many deflections occurred in a given stretch between substitutions (often referred to as a “stint”) to actually calculate that. In an ideal world you would have a record of which exact possessions contained a deflection to have more flexibility with the data, but what we have isn’t even half of that. The best we have right now is the ability to generate how many happened in a specific game, and even that’s not straightforward in the existing data.
LeBron James ‘could care less’ about Donald Trump’s support. dark. Next
If, however, the NBA were to release a play by play that included deflections, or far more likely, an NBA team decided to do something with this internally, this could be a next step in improving the predictive accuracy of aggregate statistics, however marginally.