Fairly plausible differences in offense and defense analytics
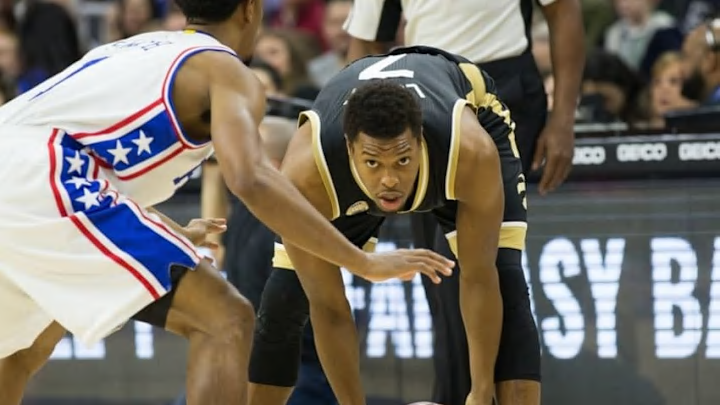
Ever since of the invention box score, basketball statistics have skewed towards the offensive end with things a little more muddy on defense. There have been incremental improvements along the way with the addition of steals, splitting offensive and defensive rebounds, play-by-play data and eventually SportVU tracking data. Those new tools are improving our understanding of defensive prowess like rim protection , the creation of regularized adjusted plus-minus models from play-by-play lineup data, or additions to SPM models, like my Player Tracking Plus Minus (PT-PM) and Justin Willard’s Dredge.
Still the general consensus is that our analytic understanding of offense remains far higher than of defense. This is particularly true when comes to the analytic understanding of individual player’s contributions, in terms of both public and NBA front office understanding.
In part as a test and to provide some quantification to the offense/defense gap, as well as to improve my projection system, I decided to break up the prediction metric I use for team projections into the the offensive and defensive player rating components for each player and retrodict last season’s win total for each team. My projections — using a blend of my PT-PM and RAPM as player metrics –have been among the more successful systems in the last two years based on the APBR metric contest. (I have beaten both the Vegas lines and 538’s CARMELO system each of the last two years.)
The deconstructed offensive and defensive projections don’t exactly mimic my overall projections due to differences in the aging curve adjustments, regression towards the mean adjustments, and schedule adjustments, all of which are applied to the overall metric in the pre-season win estimate.
For the offensive and defensive retrodictions I am testing them against the offensive and defensive team ratings (ORtg and DRtg) from Basketball-Reference converted to Pythagorean wins, which gives an approximation of the team’s record had the other side of the ball been exactly league average. This is an imperfect estimate of team quality, as teams frequently slightly over or under perform their Pythagorean wins. Last year’s Warrior’s outperformed their point differential by about eight wins.
A further caveat is that performance on each end of the floor is not independent of the other. Good offense helps set up the defense and vice versa, evidenced by a modest correlation between offensive and defensive team ratings.
But, ORtg/DRtg are likely the best, most straight forward approximations of contribution to winning or losing on coming from each side of the ball.
The evidence from my projections compared to ORtg’s and DRtg’s indicate that my player ratings were somewhat better at predicting offensive prowess (or lack thereof) than defensive prowess. This is shown by the error rates expressed as mean absolute error (MAE), the root mean squared error (RMSE), which penalizes large misses more, though for both measures two tailed t-tests indicated an approximately 16 percent chance of the differences arising randomly, below the traditional level of 5 percent chance to be termed as statistically significant, and lastly, by the coefficient of determination:
Offensive Rating:
- MAE was 4.1
- RMSE was 5.3 games.
Defensive Rating:
- MAE was 5.3
- RMSE was 6.2 games.
Offensive prediction error was 28% lower by MAE and 22% lower by RMSE.
Below is an image of a Bayesian Estimate simulation run of the difference between the error rate on offense and defense based on the 2015-16 numbers, again showing a difference that is less than the 95 percent highest density interval, with the defensive error being less than the offensive error in 8.4 percent of simulations.
Another way to quantify the error in prediction is simply to compare the coefficient of determination, or, R-squared, between the out of sample model prediction and the actual results, which was .70 r^2 for offensive wins and only r^2 of .43 for defensive wins between the projections and the Pythagorean wins indicated by the actual defensive rating. As shown in the scatter plots below.
Projected Offensive Wins vs Actual Offensive Wins
Projected Defensive Wins vs Actual Offensive Wins
In addition to the question of statistical significance there are even more important practical limitations. This study is, of course, just one piece of evidence. It probably depends on where your prior beliefs were in terms of our relative analytic understanding of offense and defense. There are a good number of reasons to think that we have more understanding of individual players contributions on offense, people are only now creating metrics to track who a player is guarding throughout a defensive possession, for example. In basketball, the offense tends to initiate the action, with defense being more noisy to track. But we can not draw too hard of conclusions from a test of one metric for one year. It doesn’t necessarily follow that we can generalize to other metrics that attempt to measure defensive contributions. More years added to the study would help as would other metrics that are split by offense and defense.